Transaction / Regular Paper Title
semanticscholar(2018)
摘要
Type 2 Diabetes is a leading worldwide public health concern, and its increasing prevalence has significant health and economic importance in all nations. The condition is a multifactorial disorder with a complex aetiology. The genetic determinants remain largely elusive, with only a handful of identified candidate genes. Genome wide association studies (GWAS) promised to significantly enhance our understanding of genetic based determinants of common complex diseases. To date, 83 single nucleotide polymorphisms (SNPs) for type 2 diabetes have been identified using GWAS. Standard statistical tests for single and multi-locus analysis such as logistic regression, have demonstrated little effect in understanding the genetic architecture of complex human diseases. Logistic regression is modelled to capture linear interactions but neglects the non-linear epistatic interactions present within genetic data. There is an urgent need to detect epistatic interactions in complex diseases as this may explain the remaining missing heritability in such diseases. In this paper, we present a novel framework based on deep learning algorithms that deal with non-linear epistatic interactions that exist in genome wide association data. Logistic association analysis under an additive genetic model, adjusted for genomic control inflation factor, is conducted to remove statistically improbable SNPs to minimize computational overheads. SNPs generated with a p-value threshold of 10 are considered, resulting in epistatic analysis in the remaining 6609 SNPs. Latent representations are extracted using a stacked autoencoder model and used to initialize a deep learning classifier for binary classification tasks. The performance of the proposed stacked autoencoder model is evaluated and benchmarked against traditional machine learning models. The findings show that using 2500 hidden units from our stacked autoencoder it is possible to obtain (AUC=92.89%, Sens=90.87%, Spec=80.53%, Logloss= 45.82%, Gini=85.78%, and MSE=11.62%). Deterioration in classification accuracy (AUC=89.38%) is observed using 700 hidden units.
更多查看译文
AI 理解论文
溯源树
样例
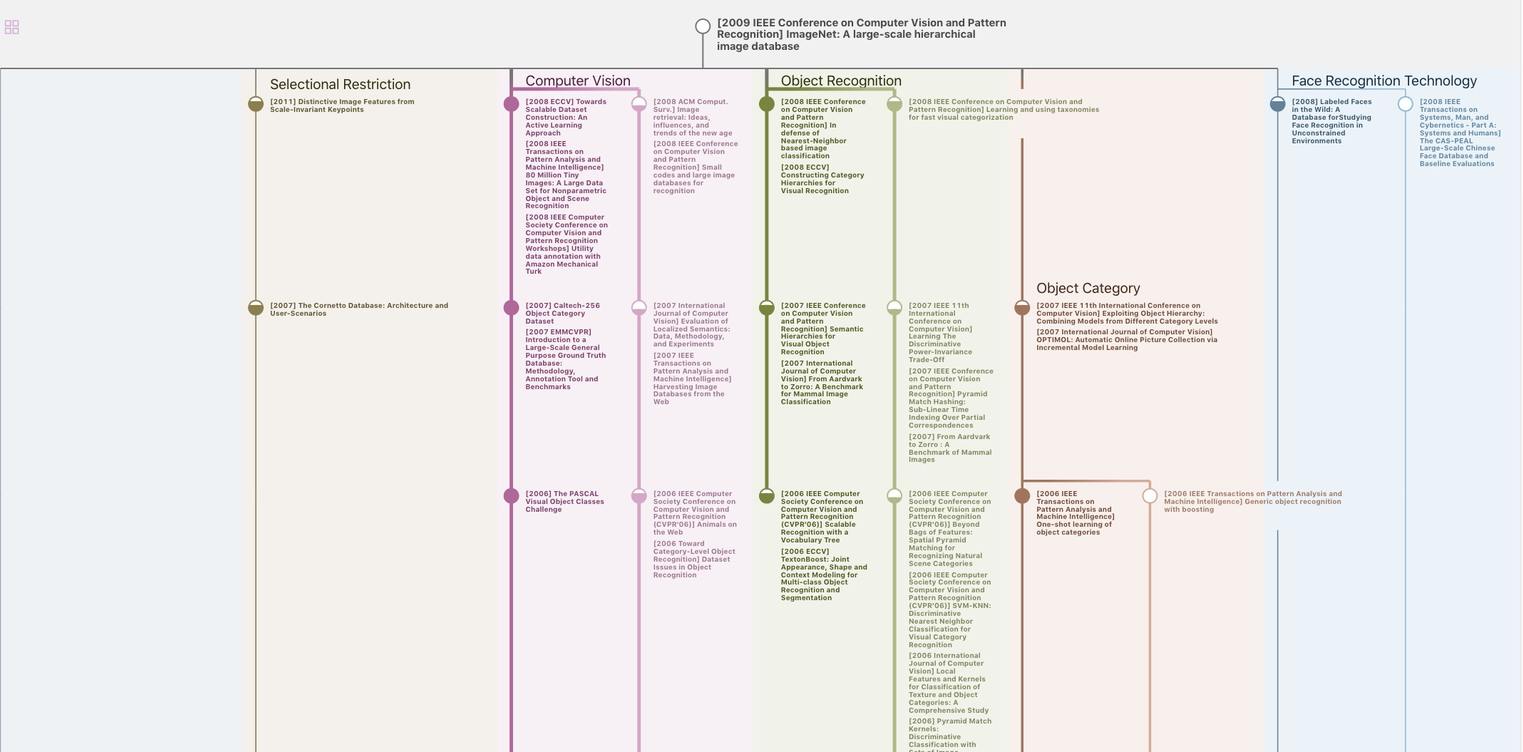
生成溯源树,研究论文发展脉络
Chat Paper
正在生成论文摘要