Understanding Satellite-Imagery-Based Crop Yield Predictions
semanticscholar(2017)
摘要
Researchers like [26] have already trained Convolutional Neural Networks to predict crop yields by county in the US using satellite images. We aim to improve upon and better understand [26]’s methodology and results. In line with their work, we use nine spectral and temperature bands from relatively low resolution satellite images as our features for predicting county-level corn and soybean yields. To ease training, we reduce the dimensionality of our data by assuming that the position of pixels doesn’t impact the average yield (the permutation invariance assumption), which allows us to use pixel intensity histograms as features. By making [26]’s model deeper, we achieve better prediction accuracy, showing that there is still signal to extract from the data. To better understand whether our models can distinguish between crops, we compute saliency maps for each image/crop pair and compare maps for various crops. We find that our model distinguishes between crops, and that, in line with previous yield prediction research, the infrared and temperature bands of images taken during peak growing season contribute the most to discrimination ability.
更多查看译文
AI 理解论文
溯源树
样例
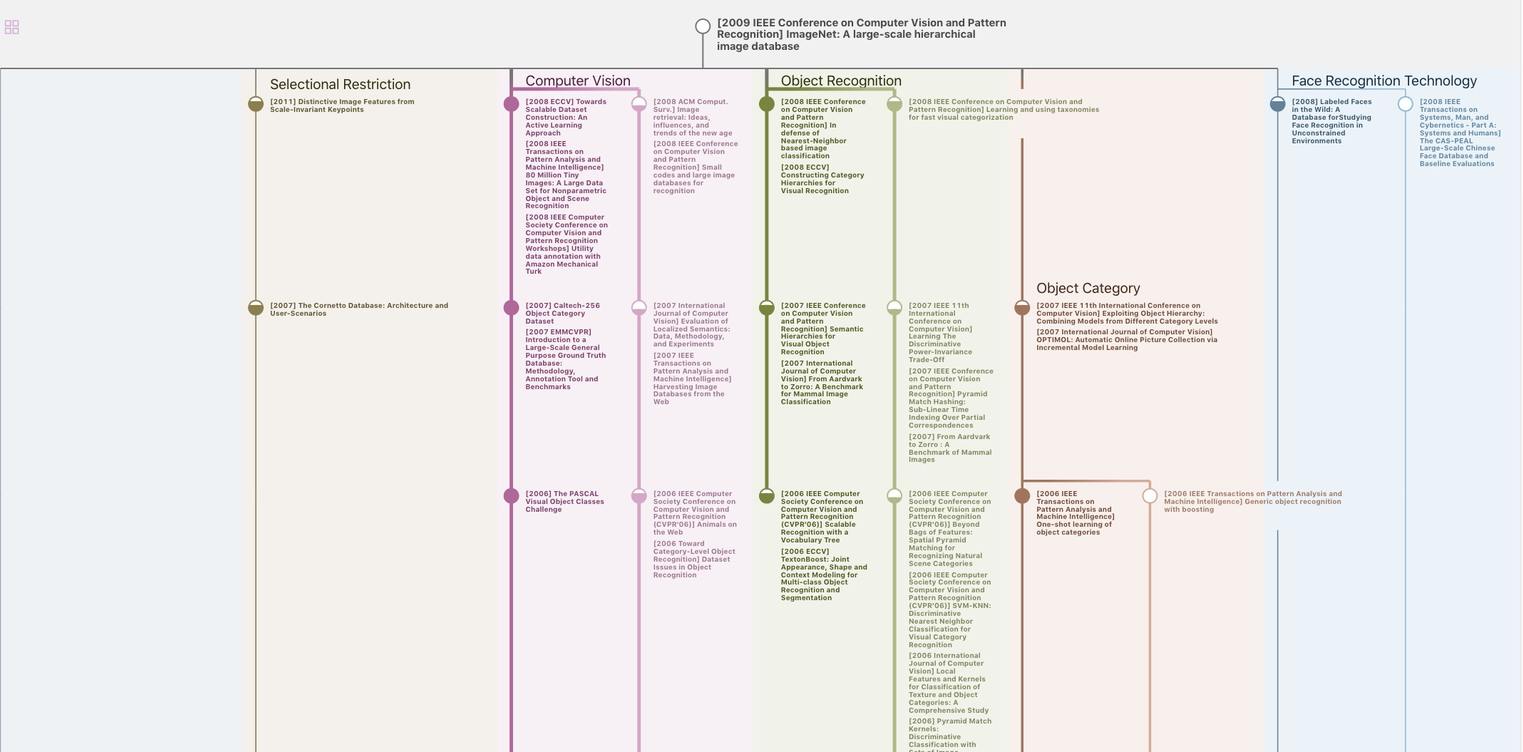
生成溯源树,研究论文发展脉络
Chat Paper
正在生成论文摘要