Private Speech Adversaries
semanticscholar(2019)
摘要
The fact that deep learning models are surprisingly fragile – yielding drastically different outputs upon small changes in input – has been well studied in the image recognition space, but this property is shared across other input modalities. In the audio domain, minor perturbations to a waveform impact speech-to-text translation and allow an attacker to arbitrarily change a recognized phrase while remaining barely noticeable to an outside listener. We demonstrate that that these perturbations can also be used for good – by applying simple learned perturbations to audio signals, we are able to prevent successful transcription in speech-to-text systems; our approach defends against malicious eavesdropping and mass transcription of private audio content while still maintaining human interpretability of the signal. Furthermore, our learned perturbations, including a fixed universal perturbation, do not require optimizing towards a specific audio sequence or having the full audio recording available in advance. These perturbations are simple to apply in real-time and provide a step towards ensuring greater privacy in largely unmonitored digital environments.
更多查看译文
AI 理解论文
溯源树
样例
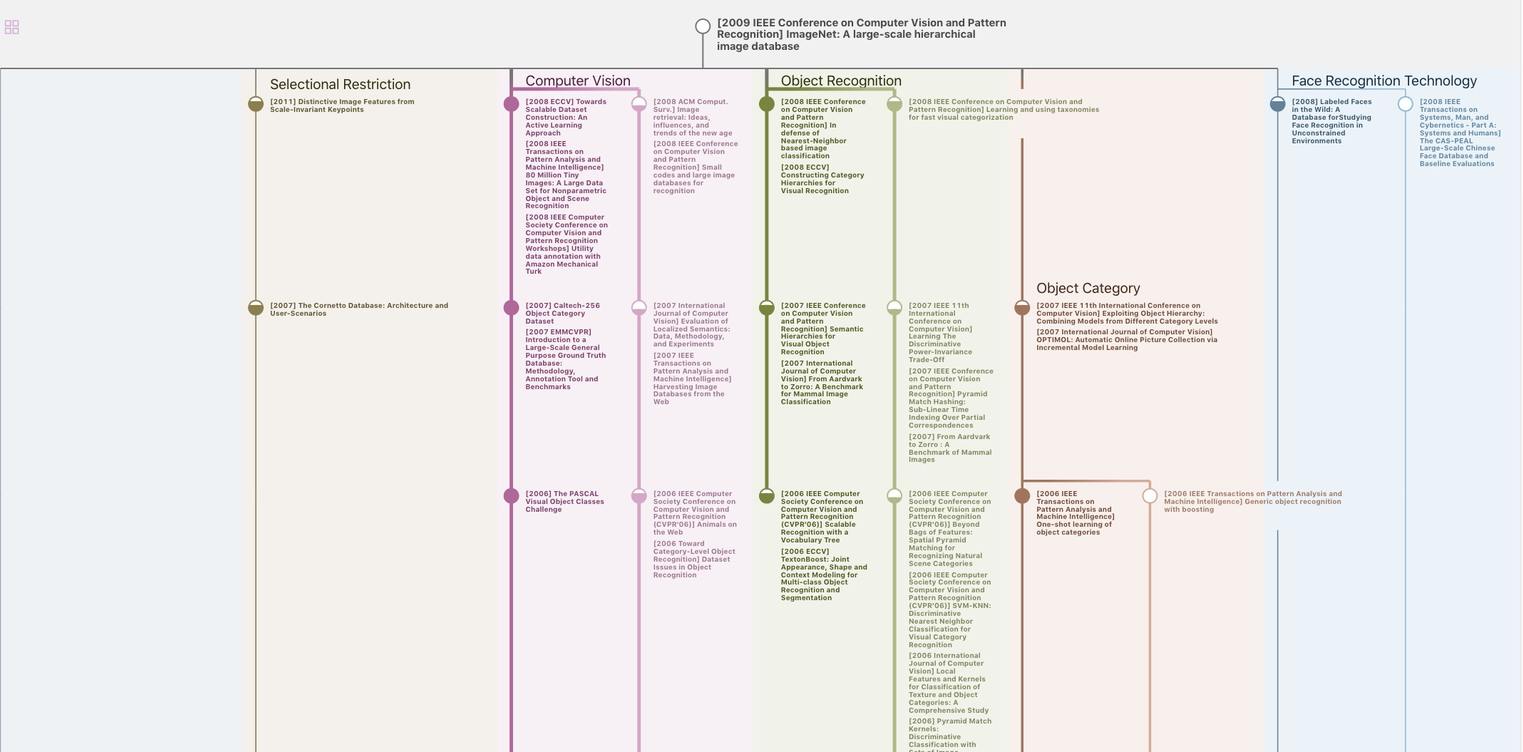
生成溯源树,研究论文发展脉络
Chat Paper
正在生成论文摘要