Receptive Fields Neural Networks using the Gabor Kernel Family
semanticscholar(2017)
摘要
Image classification is an increasingly important field within machine learning. Recently, convolutional neural networks (CNN’s) have been proven to be one of the most successful approaches. CNN’s perform outstanding when ample training data is available. However, because CNN’s have a large number of parameters they are prone to overfitting, meaning it will work well on training data, but not on unseen data. Moreover, there are no specific mechanisms in a CNN to take variances, like scale and rotation, into account. Jacobsen et al. [1] proposed a model to overcome these problems, called the receptive field neural network (RFNN). We extend upon the results of Jacobsen et al. to use a weighted combinations of fixed receptive fields in a convolutional neural network. The key difference is that we are using the Gabor family basis for the fixed receptive fields, instead of the Gaussian derivative basis. The use of the Gabor family is inspired by their ability to model receptive fields in the visual system in the mammal cortex and their use in the field of computer vision. We performed an exploratory study on the Gabor family basis in the RFNN model using three well established datasets of images, the Handwritten Digit dataset (MNIST), the MNIST Rotated and the German Traffic Signs dataset (GTSRB). Our results show that for fewer training examples the Gabor RFNN performs better than the classical CNN. Moreover, the results compared with the Gaussian RFNN suggest that the Gabor family basis has a lot of potential in the RFNN model, performing close to state-of-the-art models. In the future, we should look for a method of learning the Gabor function parameters, making it less sensitive to parameters chosen a priori.
更多查看译文
AI 理解论文
溯源树
样例
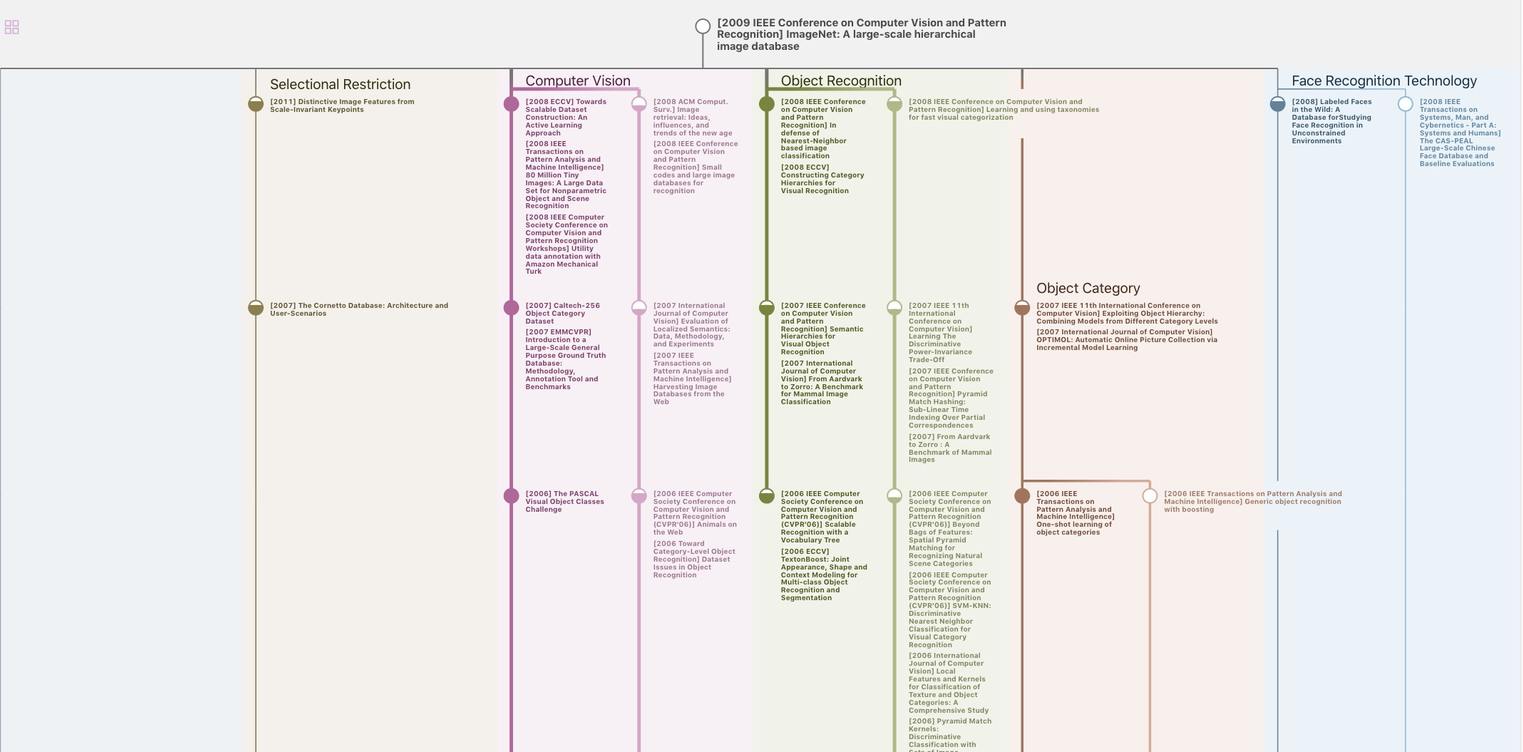
生成溯源树,研究论文发展脉络
Chat Paper
正在生成论文摘要