Relaxing the iid assumption: adaptively minimax optimal regret via root-entropic regularization
Annals of Statistics(2023)
摘要
We consider prediction with expert advice when data are generated from distributions varying arbitrarily within an unknown constraint set. This semiadversarial setting includes (at the extremes) the classical i.i.d. setting, when the unknown constraint set is restricted to be a singleton, and the unconstrained adversarial setting, when the constraint set is the set of all distributions. The Hedge algorithm-long known to be minimax (rate) optimal in the adversarial regime-was recently shown to be simultaneously minimax optimal for i.i.d. data. In this work, we propose to relax the i.i.d. assumption by seeking adaptivity at all levels of a natural ordering on constraint sets. We provide matching upper and lower bounds on the minimax regret at all levels, show that Hedge with deterministic learning rates is suboptimal outside of the extremes and prove that one can adaptively obtain minimax regret at all levels. We achieve this optimal adaptivity using the follow-the-regularizedleader (FTRL) framework, with a novel adaptive regularization scheme that implicitly scales as the square root of the entropy of the current predictive distribution, rather than the entropy of the initial predictive distribution. Finally, we provide novel technical tools to study the statistical performance of FTRL along the semi-adversarial spectrum.
更多查看译文
关键词
Sequential decision theory, prediction with expert advice, adaptive minimax regret, aggregation, robust prediction
AI 理解论文
溯源树
样例
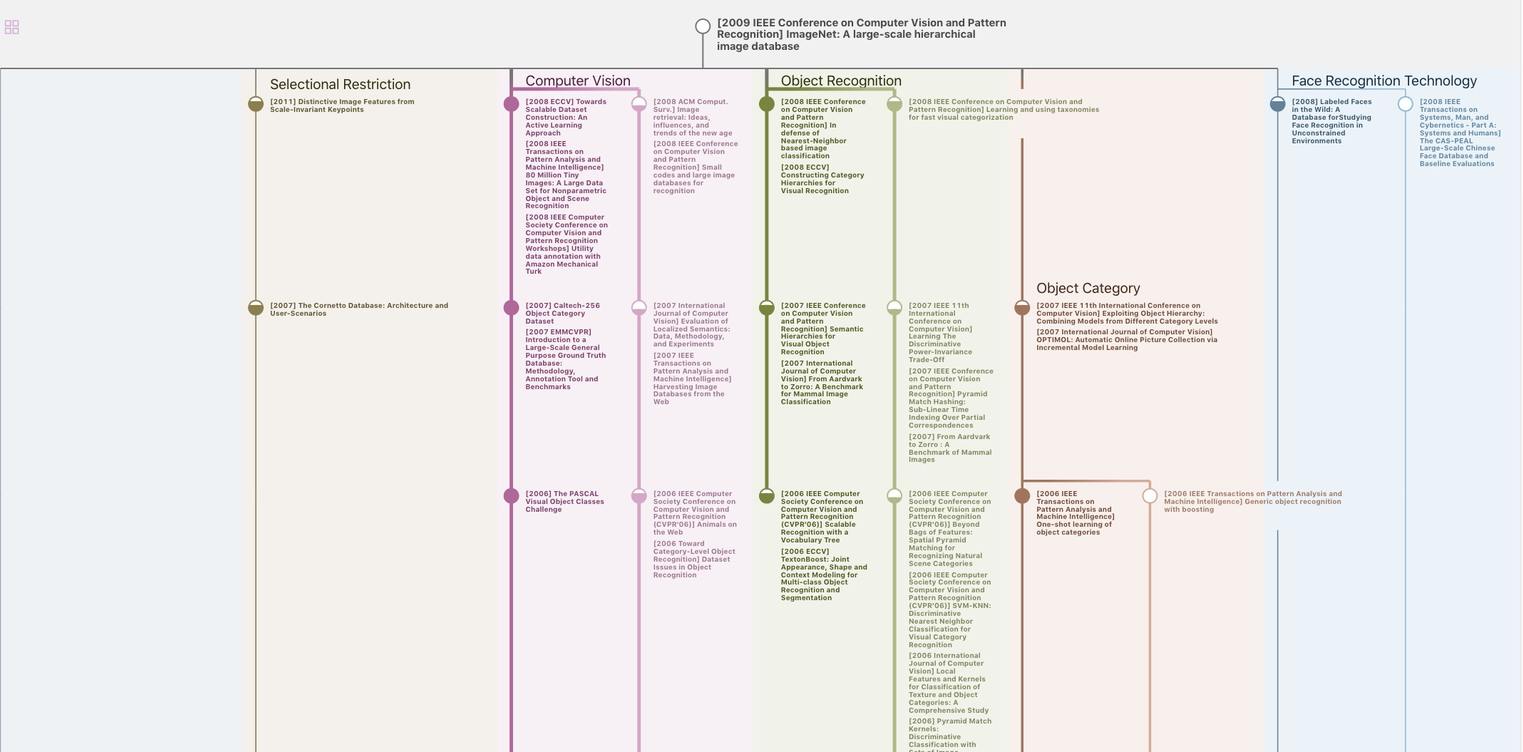
生成溯源树,研究论文发展脉络
Chat Paper
正在生成论文摘要