AirCapRL: Autonomous Aerial Human Motion Capture Using Deep Reinforcement Learning
IEEE ROBOTICS AND AUTOMATION LETTERS(2020)
摘要
In this letter, we introduce a deep reinforcement learning (DRL) based multi-robot formation controller for the task of autonomous aerial human motion capture (MoCap). We focus on vision-based MoCap, where the objective is to estimate the trajectory of body pose, and shape of a single moving person using multiple micro aerial vehicles. State-of-the-art solutions to this problem are based on classical control methods, which depend on hand-crafted system, and observation models. Such models are difficult to derive, and generalize across different systems. Moreover, the non-linearities, and non-convexities of these models lead to sub-optimal controls. In our work, we formulate this problem as a sequential decision making task to achieve the vision-based motion capture objectives, and solve it using a deep neural network-based RL method. We leverage proximal policy optimization (PPO) to train a stochastic decentralized control policy for formation control. The neural network is trained in a parallelized setup in synthetic environments. We performed extensive simulation experiments to validate our approach. Finally, real-robot experiments demonstrate that our policies generalize to real world conditions.
更多查看译文
关键词
Reinforecment learning,aerial systems,perception and autonomy,multi-robot systems,visual tracking
AI 理解论文
溯源树
样例
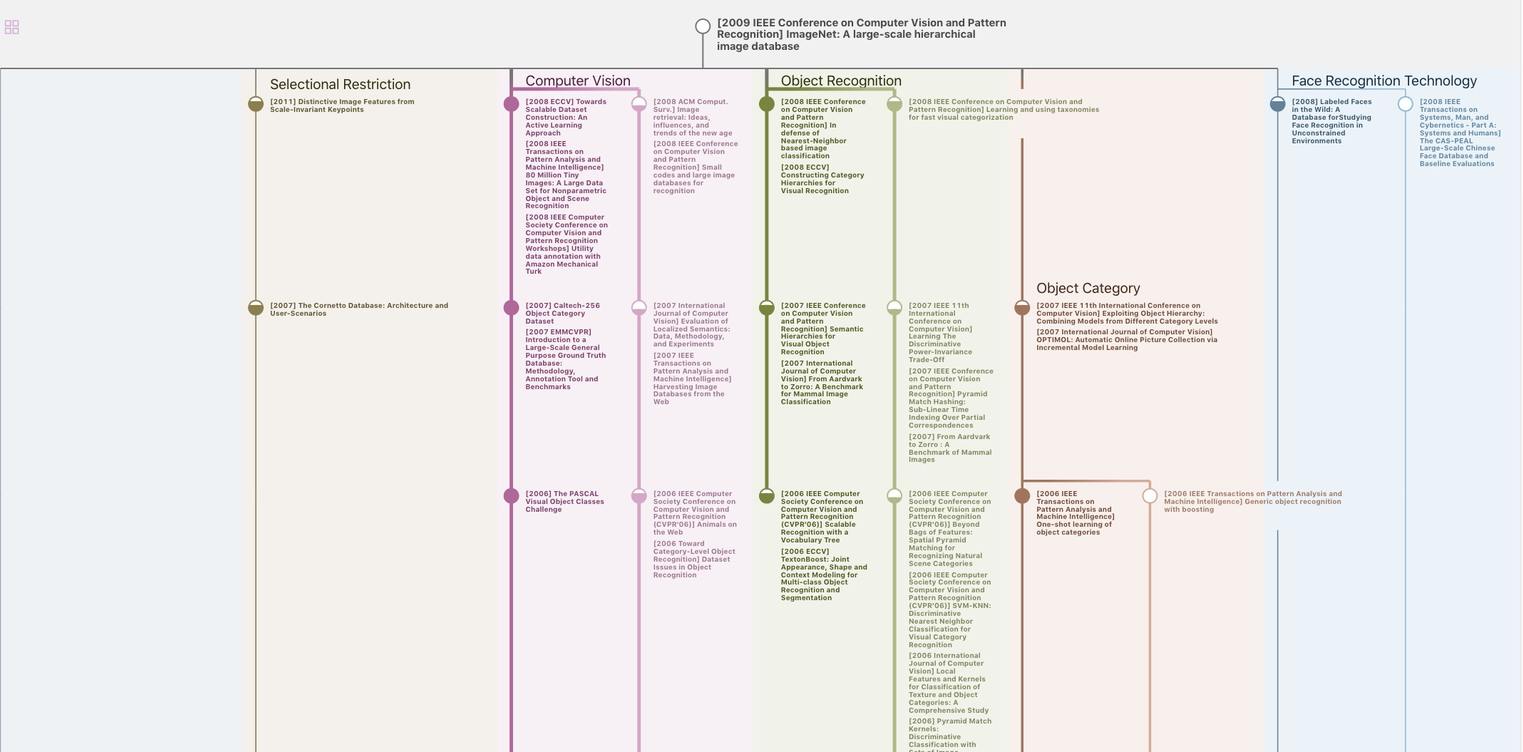
生成溯源树,研究论文发展脉络
Chat Paper
正在生成论文摘要