Towards Scalable Geospatial Remote Sensing for Efficient OSM Labeling
Editors(2019)
摘要
The past decade has shown a dramatic increase in the amount of openly available geo-spatial datasets such as multi-spectral and RADAR satellite imagery from space agencies like ESA and NASA government-sponsored, high-resolution aerial survey raster data, eg, USDA (US Department of Agriculture) weather reanalysis model data based on sensor networks, eg published by the PRISM Climate group geo-tagged messages as well as images from social media platforms such as Twitter and Instagram, etc. accumulating geo-tagged information at data rates easily exceeding tens of terabytes a day. Given that an open database project such as OpenStreetMap (OSM) relies on volunteers to spend their valuable time to generate vector datasets that annotate and update information on roads, buildings, land cover, points of interest, etc., it is natural to ask how sources of freely available spatio-temporal information might help to support and guide mappers in their work.At the same time, major progress has been made in the open-source digital arena of big data processing and artificial intelligence (AI). For example, projects for distributed non-relational database systems such as HBase ( https://hbase. apache. org/) or in-memory distributed compute frameworks such as Spark are available to run on commodity hardware to scale analytics. Deep learning libraries such as PyTorch ( https://pytorch. org) in accordance with the explosive amount of neural network architectures published by academia enable, for example, state-of-the-art computer vision algorithms which can be leveraged for remote sensing tasks: detection of buildings, land …
更多查看译文
AI 理解论文
溯源树
样例
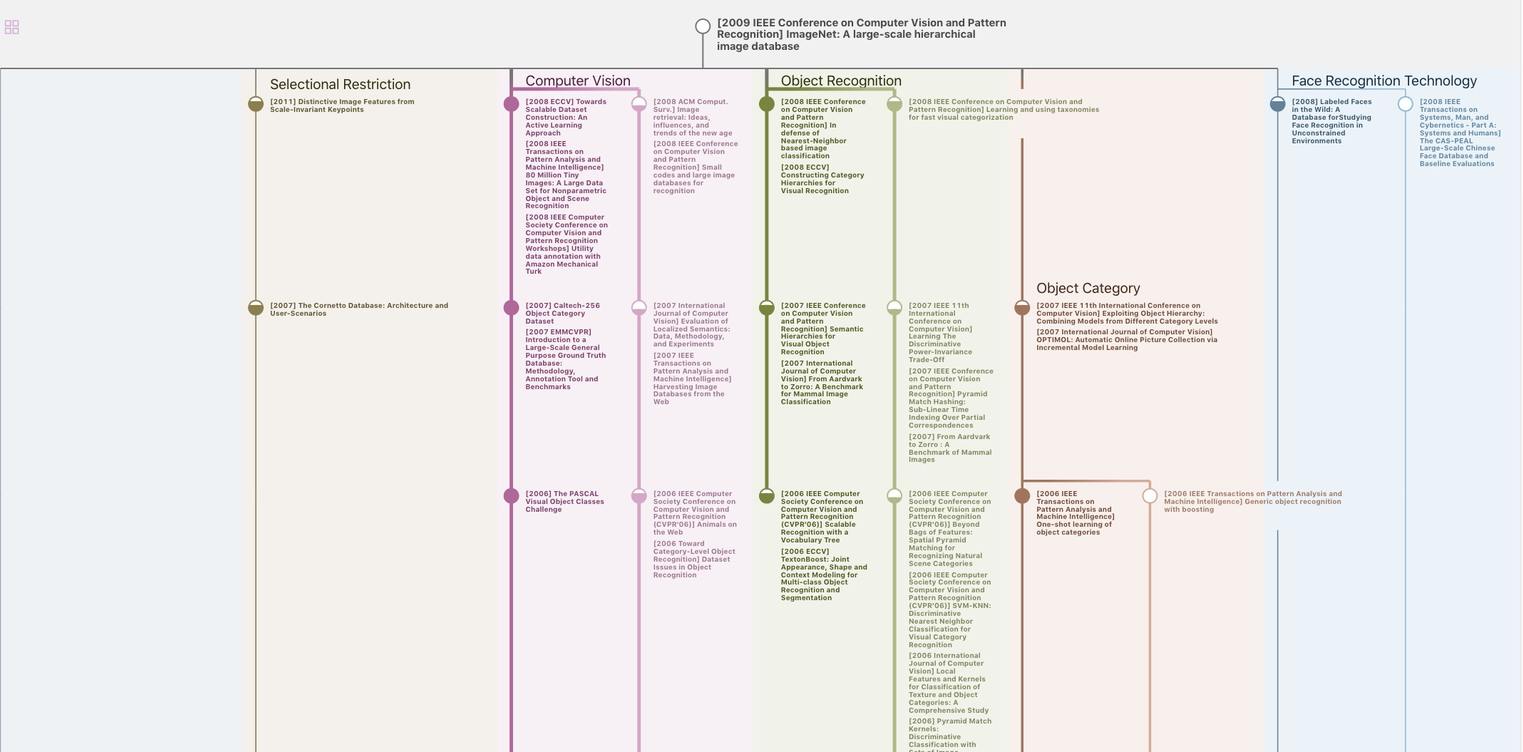
生成溯源树,研究论文发展脉络
Chat Paper
正在生成论文摘要