Decoupling Inherent Risk and Early Cancer Signs in Image-based Breast Cancer Risk Models
medical image computing and computer assisted intervention(2020)
摘要
The ability to accurately estimate risk of developing breast cancer would be invaluable for clinical decision-making. One promising new approach is to integrate image-based risk models based on deep neural networks. However, one must take care when using such models, as selection of training data influences the patterns the network will learn to identify. With this in mind, we trained networks using three different criteria to select the positive training data (i.e. images from patients that will develop cancer): an inherent risk model trained on images with no visible signs of cancer, a cancer signs model trained on images containing cancer or early signs of cancer, and a conflated model trained on all images from patients with a cancer diagnosis. We find that these three models learn distinctive features that focus on different patterns, which translates to contrasts in performance. Short-term risk is best estimated by the cancer signs model, whilst long-term risk is best estimated by the inherent risk model. Carelessly training with all images conflates inherent risk with early cancer signs, and yields sub-optimal estimates in both regimes. As a consequence, conflated models may lead physicians to recommend preventative action when early cancer signs are already visible.
更多查看译文
关键词
breast cancer risk,early cancer signs,breast cancer,inherent risk,image-based
AI 理解论文
溯源树
样例
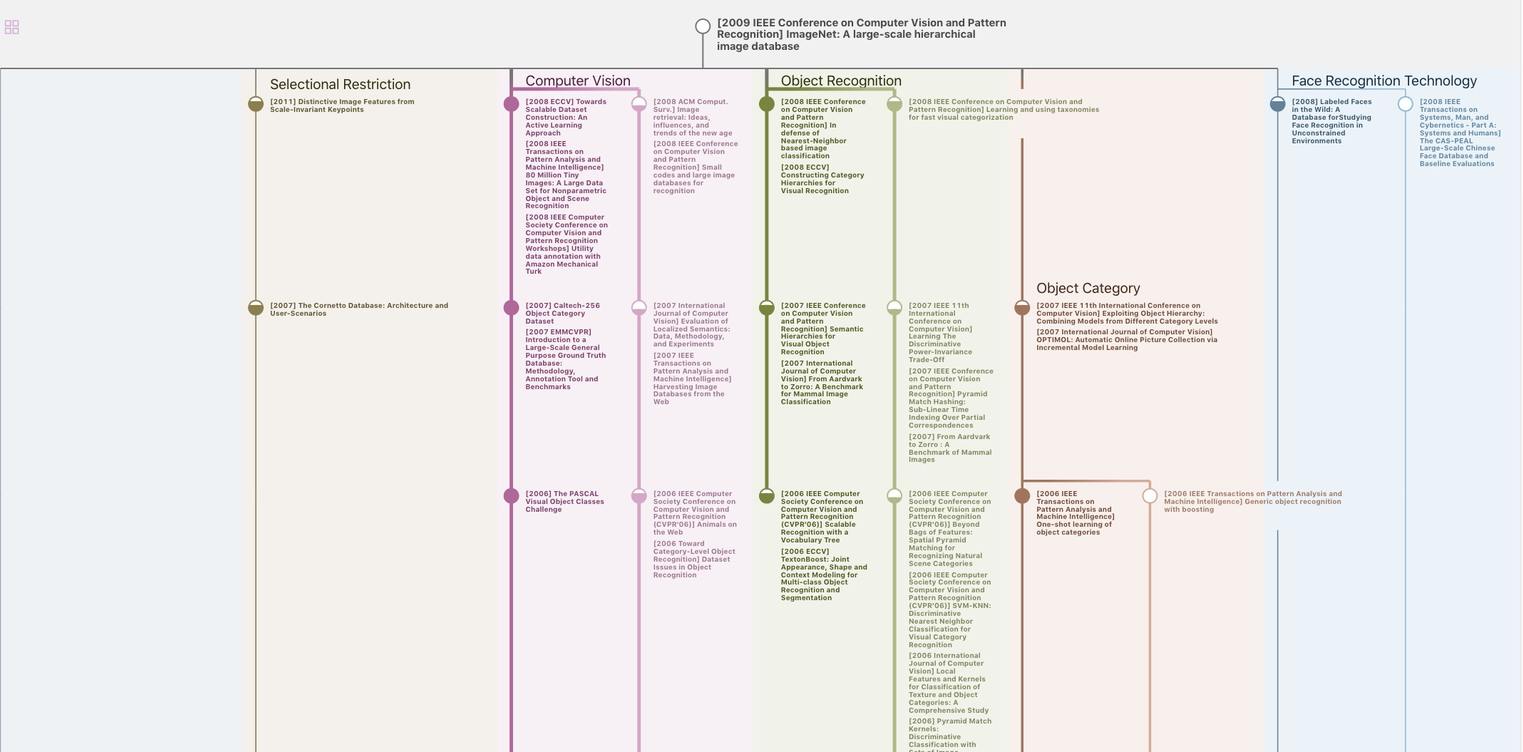
生成溯源树,研究论文发展脉络
Chat Paper
正在生成论文摘要