Deep-Learning Traffic Flow Prediction for Forecasting Performance Measurement of Public Transportation Systems
user-5f03ee444c775ed682ef5240(2020)
摘要
In this project, the authors developed a deep learning approach for traffic flow forecasting and bus arrival time estimation in Los Angeles. First, they developed a novel Graph Convolutional Recurrent Neural Network (GCRNN) to model and forecast traffic flows at different spatial and temporal resolutions. The authors' GCRNN model considers not only the location of traffic sensors but also their relationships (ie, topological dependency) in space, which was critical to achieving the best performance for all forecasting horizons compared to the existing methods. Next, the authors implemented a Geo-Convolution Long Short-Term Memory (Geo-Conv LSTM) framework to model bus Estimated Time of Arrival (ETA) by incorporating the traffic flow predictions of their GCRNN. Using the real-world traffic sensor datasets archived in the authors' data warehouse, they showed that their proposed bus ETA model is more accurate than the existing method, Gradient Boosted Decision Tree (GBDT), by 27% in estimating bus travel time. Lastly, the authors deployed both models as web applications so that users can access traffic prediction data and check bus arrival times to a destination location from a starting point.
更多查看译文
AI 理解论文
溯源树
样例
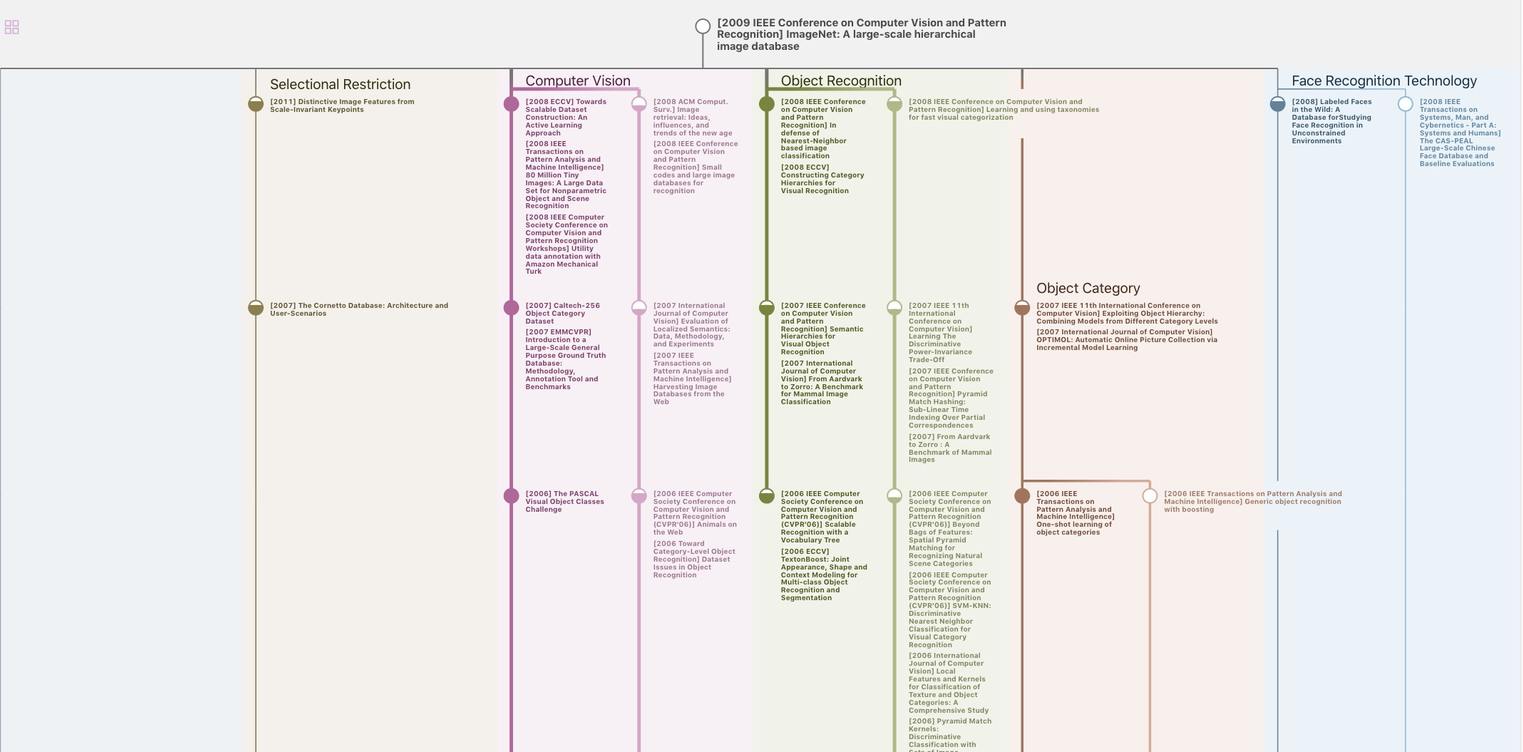
生成溯源树,研究论文发展脉络
Chat Paper
正在生成论文摘要