Accurate and Reliable Prediction of Relative Protein-Ligand Binding Affinities via Free Energy Calculations: Validation in Prospective Drug Discovery
semanticscholar(2015)
摘要
Designing tight binding ligands is a primary objective of small molecule drug discovery. Over the past few decades, free energy calculations have benefited from improved force fields and sampling algorithms, as well as the advent of low cost parallel computing. However, it has proven to be challenging to reliably achieve the level of accuracy that would be needed to guide lead optimization (~5X in binding affinity) for a wide range of ligands and protein targets. Not surprisingly, widespread commercial application of free energy simulations has been limited due to the lack of large-scale validation coupled with the technical challenges traditionally associated with running these types of calculations. Here, we report an approach that achieves an unprecedented level of accuracy across a broad range of target classes, with retrospective results encompassing 200 ligands and a wide variety of chemical perturbations, many of which involve significant changes in ligand chemical structures. In addition, we have applied the method in prospective drug discovery projects and found a significant improvement in the quality of the compounds synthesized that have been predicted to be potent. Compounds predicted to be potent by this approach have a substantial reduction in false positives relative to compounds synthesized based on other computational or medicinal chemistry approaches. Furthermore, the results are consistent with those obtained from our retrospective studies, demonstrating the robustness and broad range of applicability of this approach, which can be used to drive decisions in lead optimization.
更多查看译文
AI 理解论文
溯源树
样例
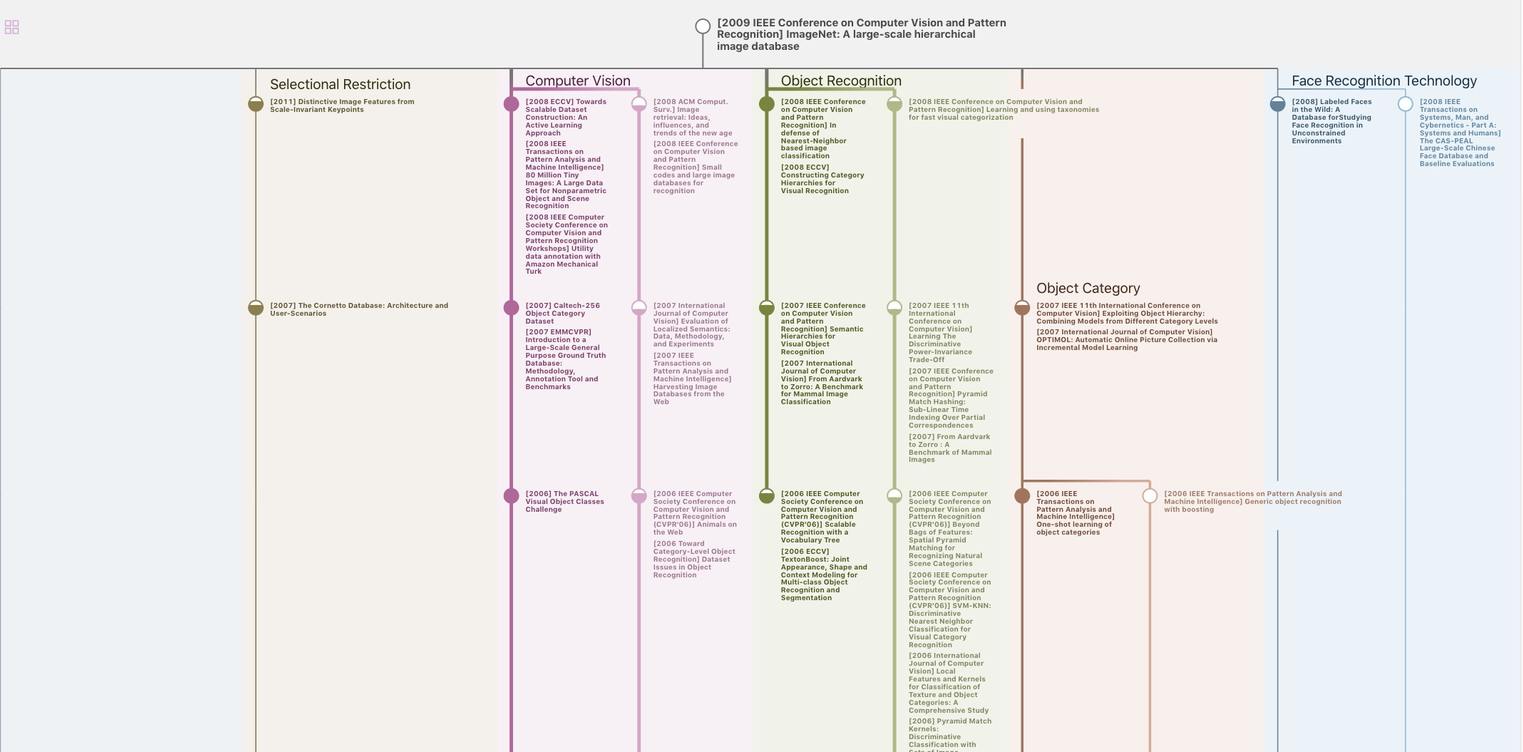
生成溯源树,研究论文发展脉络
Chat Paper
正在生成论文摘要