Web-Age Information Management
Lecture Notes in Computer Science(2016)
摘要
Modeling user behavior and latent preference implied in rating data are the basis of personalized information services. In this paper, we adopt a latent variable to describe user preference and Bayesian network (BN) with a latent variable as the framework for representing the relationships among the observed and the latent variables, and define user preference BN (abbreviated as UPBN). To construct UPBN effectively, we first give the property and initial structure constraint that enable conditional probability distributions (CPDs) related to the latent variable to fit the given data set by the ExpectationMaximization (EM) algorithm. Then, we give the EM-based algorithm for constraint-based maximum likelihood estimation of parameters to learn UPBN’s CPDs from the incomplete data w.r.t. the latent variable. Following, we give the algorithm to learn the UPBN’s graphical structure by applying the structural EM (SEM) algorithm and the Bayesian Information Criteria (BIC). Experimental results show the effectiveness and efficiency of our method.
更多查看译文
AI 理解论文
溯源树
样例
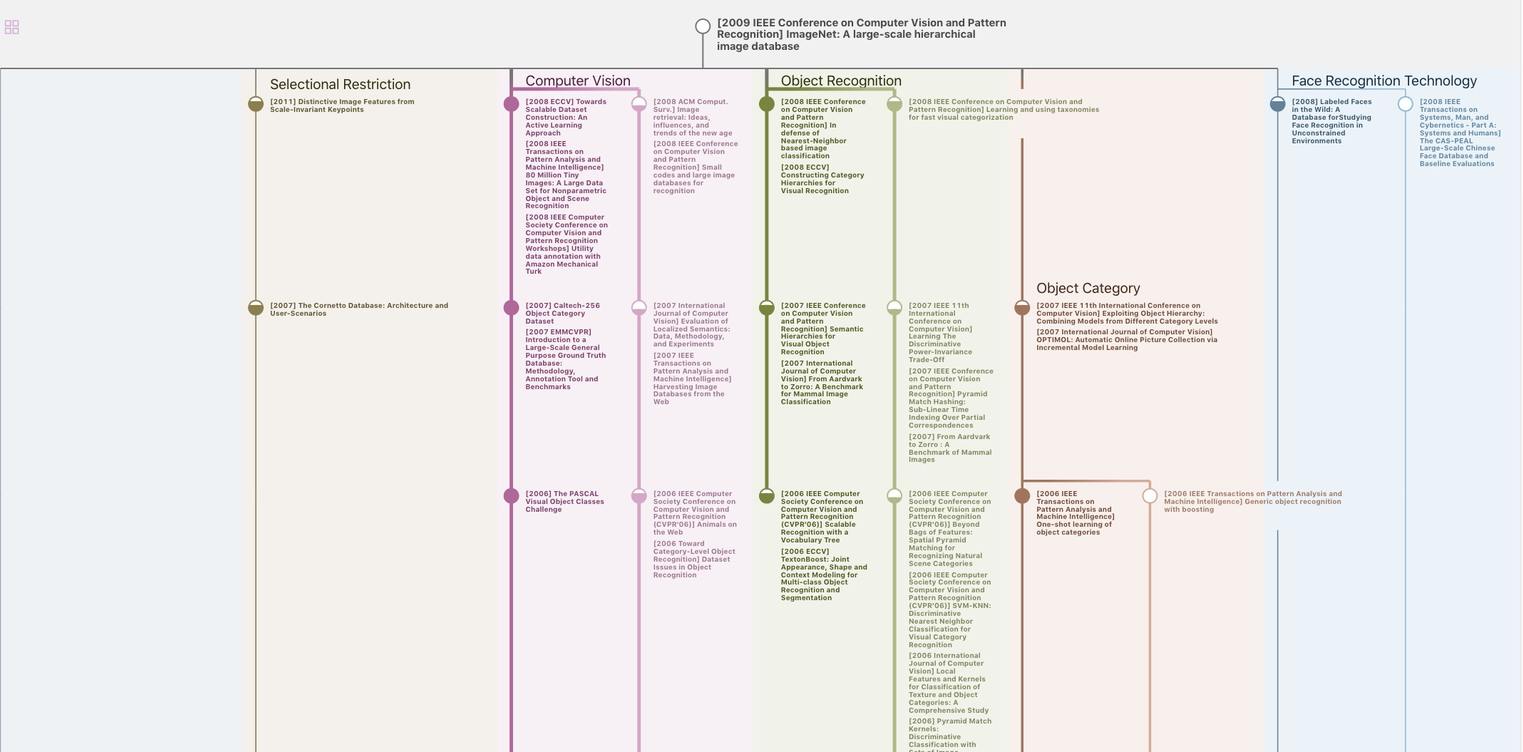
生成溯源树,研究论文发展脉络
Chat Paper
正在生成论文摘要