A Multi-Scale Best Basis Sparse Learning Framework for Efficient IoT Big Data Applications
semanticscholar(2018)
摘要
With the emergence of the Internet of Things (IoT) and cheap wireless sensors networks, IoT telemetry has received much interest over a wide range of fields including industrial applications and machine monitoring, telemedicine, and wearables. In the era of Big Data, there has been an ever-growing demand for efficient modeling and analyzing of high resolution and large-scale volumes of data. In response to these challenges, efficient signal representation has been drawing much attention lately within the research community. Concepts of sparse representation and compressed Sensing are emerging techniques that have great potentials to meet some of challenges faced by IoT and Big Data such as device power consumption, data redundancy, bandwidth, and data storage and transmission. In this paper we present a novel Multi-Scale Adaptive sparse learning framework for efficient signal IoT BIG DATA applications. Given a test set of the signal of interest, an adaptive dictionary learning process is proposed for sparse and redundant signal representation. First, a Joint-best basis representation is learnt in the wavelet packet domain that is optimized for the class of signals of interest. Next, dictionary learning is performed in the wavelet packet domain. The proposed framework enjoys the merits of being adaptable, efficient, and easy to deploy. The proposed framework allows for the extract of an optimal sparse representation for the signals of interest while preserving the discriminatory characteristics. Experimental results are presented to evaluate the performance of the new framework using a wide range of signals including: faulty machinery vibration signals, ECG signals, Surface Myoelectric signals, and seismic gamma ray signals.
更多查看译文
AI 理解论文
溯源树
样例
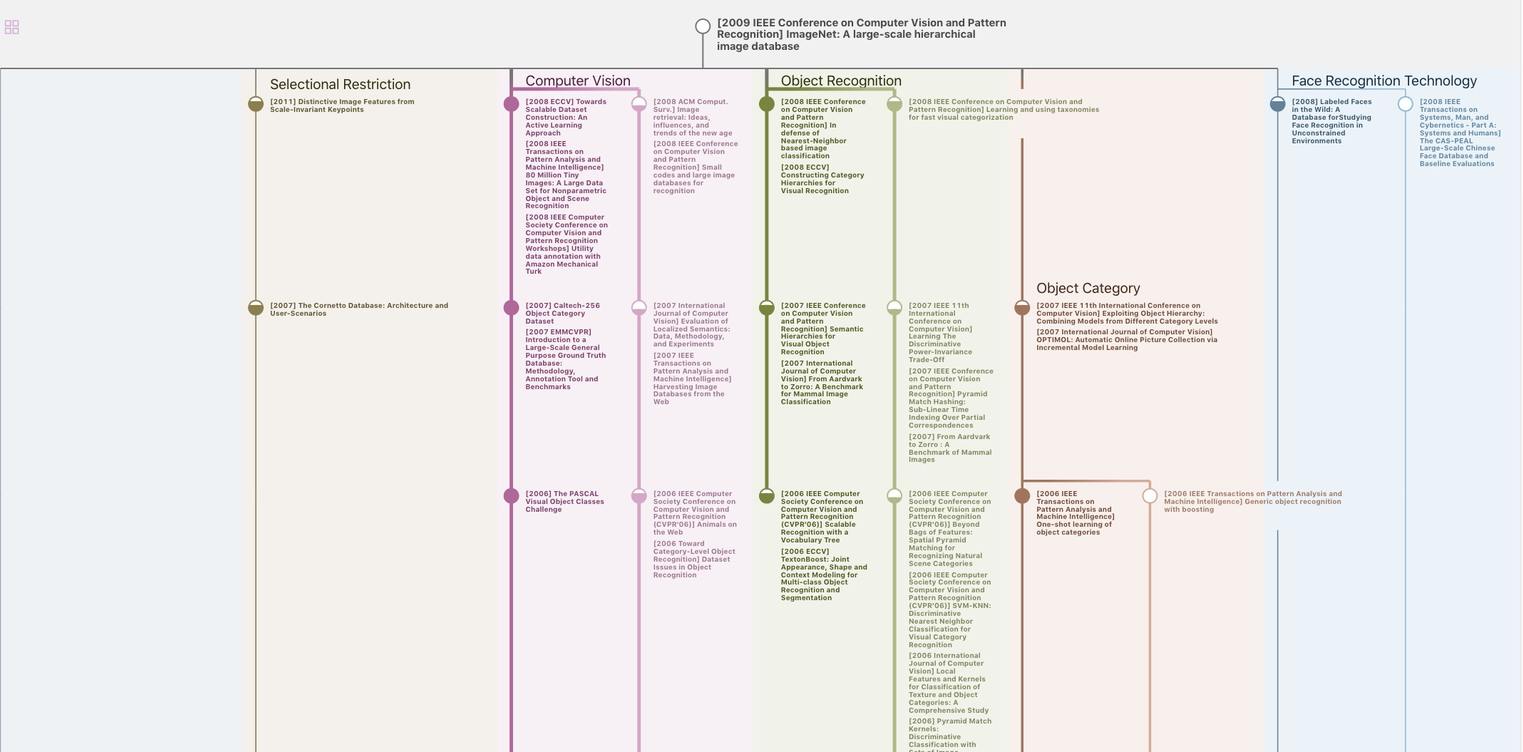
生成溯源树,研究论文发展脉络
Chat Paper
正在生成论文摘要