On Overfitting and Asymptotic Bias in Batch Reinforcement Learning with Partial Observability (Extended Abstract).
IJCAI(2020)
摘要
When an agent has limited information on its environment, the suboptimality of an RL algorithm can be decomposed into the sum of two terms: a term related to an asymptotic bias (suboptimality with unlimited data) and a term due to overfitting (additional suboptimality due to limited data). In the context of reinforcement learning with partial observability, this paper provides an analysis of the tradeoff between these two sources of error. In particular, our theoretical analysis formally characterizes how a smaller state representation increases the asymptotic bias while decreasing the risk of overfitting.
更多查看译文
AI 理解论文
溯源树
样例
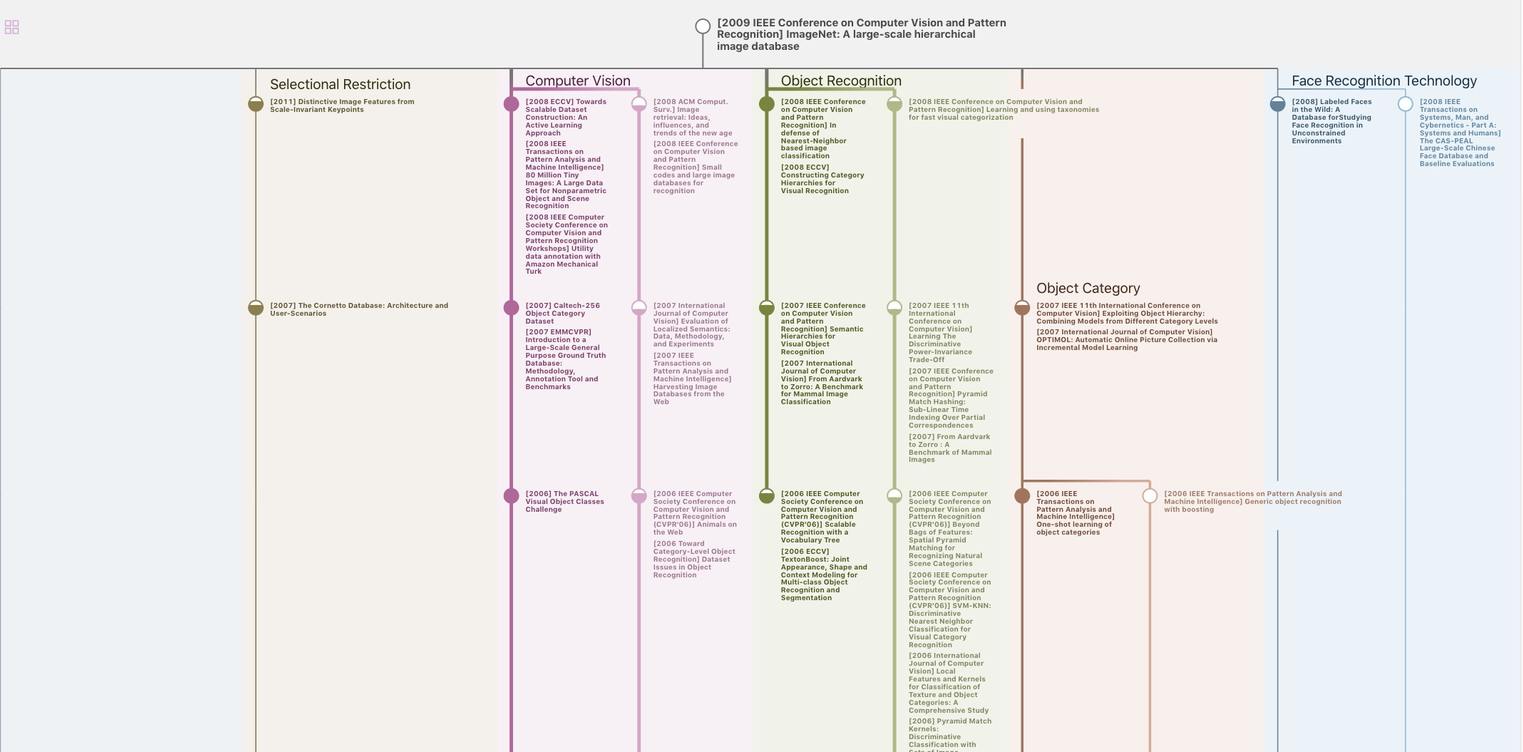
生成溯源树,研究论文发展脉络
Chat Paper
正在生成论文摘要