Benchmarking Learning Algorithms for Dexterous Multi-Arm Insertion of Semi-Deformable Objects
semanticscholar(2019)
AI Read Science
Must-Reading Tree
Example
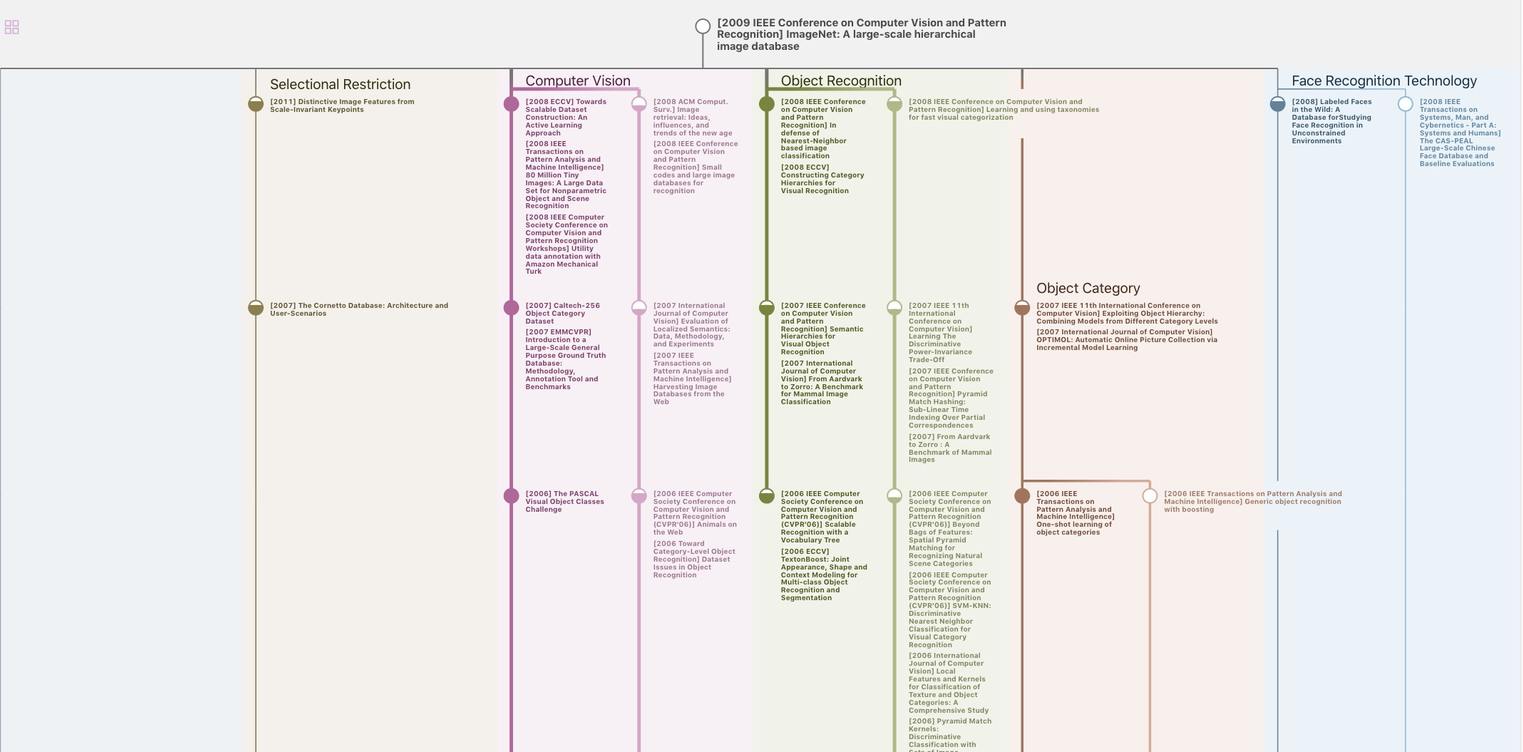
Generate MRT to find the research sequence of this paper
Chat Paper
Summary is being generated by the instructions you defined