Improving Adversarial Robustness by Enforcing Local and Global Compactness
European Conference on Computer Vision(2020)
摘要
The fact that deep neural networks are susceptible to crafted perturbations severely impacts the use of deep learning in certain domains of application. Among many developed defense models against such attacks, adversarial training emerges as the most successful method that consistently resists a wide range of attacks. In this work, based on an observation from a previous study that the representations of a clean data example and its adversarial examples become more divergent in higher layers of a deep neural net, we propose the Adversary Divergence Reduction Network which enforces local/global compactness and the clustering assumption over an intermediate layer of a deep neural network. We conduct comprehensive experiments to understand the isolating behavior of each component (i.e., local/global compactness and the clustering assumption) and compare our proposed model with state-of-the-art adversarial training methods. The experimental results demonstrate that augmenting adversarial training with our proposed components can further improve the robustness of the network, leading to higher unperturbed and adversarial predictive performances.
更多查看译文
关键词
Adversarial robustness,Local compactness,Global compactness,Clustering assumption
AI 理解论文
溯源树
样例
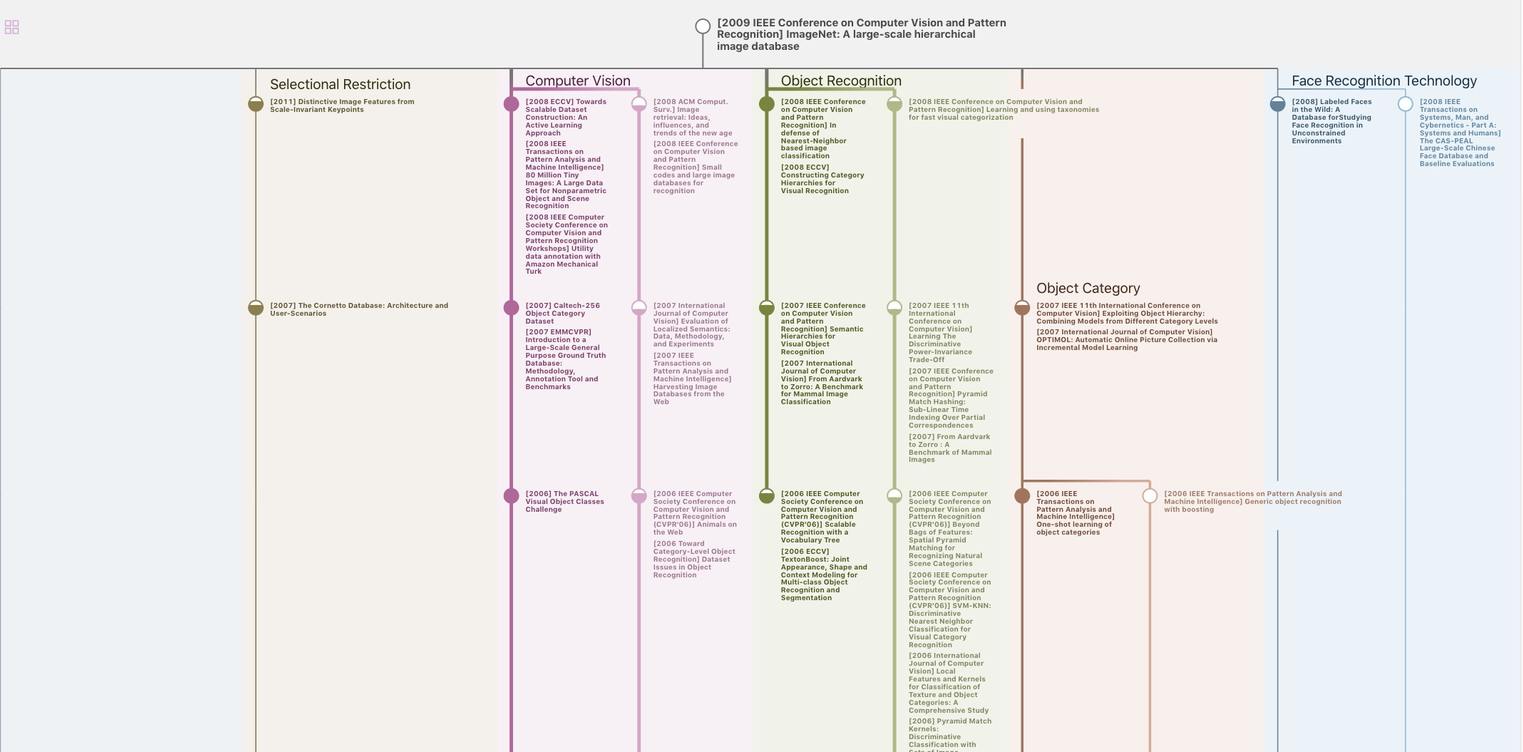
生成溯源树,研究论文发展脉络
Chat Paper
正在生成论文摘要