Information-Theoretic Approach to Navigation for Efficient Detection and Classification of Underwater Objects
arxiv(2020)
摘要
This paper addresses an autonomous exploration problem in which a mobile sensor, placed in a previously unseen search area, utilizes an information-theoretic navigation cost function to dynamically select the next sensing action, i.e., location from which to take a measurement, to efficiently detect and classify objects of interest within the area. The information-theoretic cost function proposed in this paper consist of two \textit{information gain} terms, one for detection and localization of objects and the other for sequential classification of the detected objects. We present a novel closed-form representation for the cost function, derived from the definition of mutual information. We evaluate three different policies for choosing the next sensing action: lawn mower, greedy, and non-greedy. For these three policies, we compare the results from our information-theoretic cost functions to the results of other information-theoretic inspired cost functions. Our simulation results show that search efficiency is greater using the proposed cost functions compared to those of the other methods, and that the greedy and non-greedy policies outperform the lawn mower policy.
更多查看译文
关键词
efficient detection,navigation,underwater,classification,information-theoretic
AI 理解论文
溯源树
样例
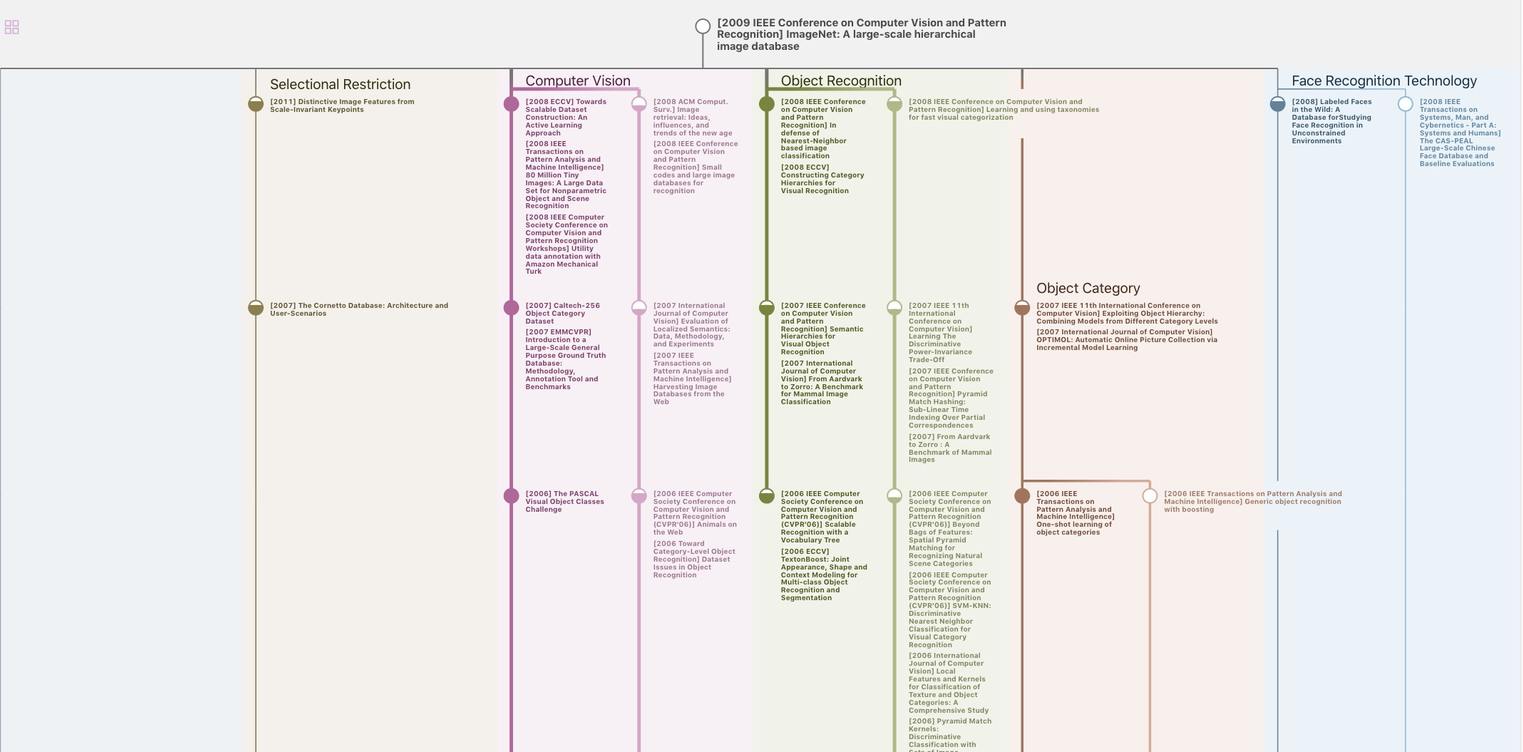
生成溯源树,研究论文发展脉络
Chat Paper
正在生成论文摘要