Product title classification versus text classification
UTexas, Austin(2012)
摘要
In most e-commerce platforms, product title classification is a crucial task. It can assist sellers listing an item in an appropriate category. At first glance, product title classification is merely an instance of text classification problems, which are well-studied in literature. However, product titles possess some properties very different from general documents. A title is usually a very short description, and an incomplete sentence. A product title classifier may need to be designed differently from a text classifier, although this issue has not been thoroughly studied. In this work, using a large-scale real-world data set, we examine conventional text-classification procedures on product title data. These procedures include word stemming, stop-word removal, feature representation and multi-class classification. Our major findings include that stemming and stop-word removal are harmful, and bigrams or degree-2 polynomial mappings are very effective. Further, if linear classifiers such as SVMs are applied, instance normalization does not downgrade the performance and binary/TF-IDF representations perform similarly. These results lead to a concrete guideline for practitioners on product title classification.
更多查看译文
AI 理解论文
溯源树
样例
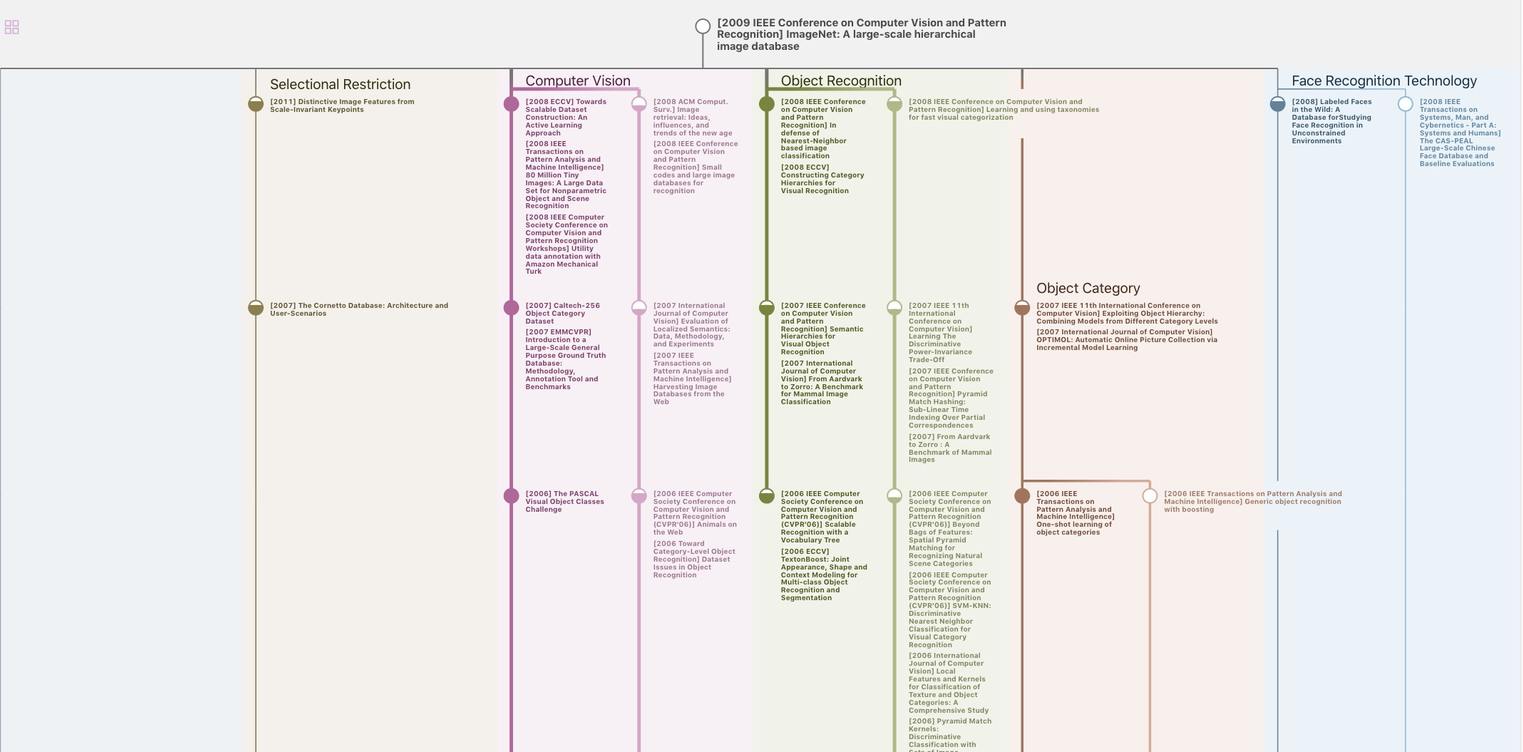
生成溯源树,研究论文发展脉络
Chat Paper
正在生成论文摘要