Trajectory Privacy-preserving through Significant Stays Protection
semanticscholar(2011)
摘要
Publication of moving objects’ everyday life trajectories may cause serious personal privacy leakage. Existing trajectory privacy-preserving methods try to anonymize k whole trajectories together, which may result in complicated algorithms and extra information loss. We observe that, background information are more relevant to where the moving objects really visit rather than where they just pass by. In this paper, we propose an approach called You Can Walk Alone (YCWA) to protect trajectory privacy through generalization of stay points on trajectories. By protecting stay points, sensitive information is protected, while the probability of whole trajectories’ exposure is reduced. Moreover, the information loss caused by the privacy-preserving process is reduced. To the best of our knowledge, this is the first research that protects trajectory privacy through protecting significant stays or similar concepts. At last, we conduct a set of comparative experimental study on real-world dataset, the results show advantages of our approach.
更多查看译文
AI 理解论文
溯源树
样例
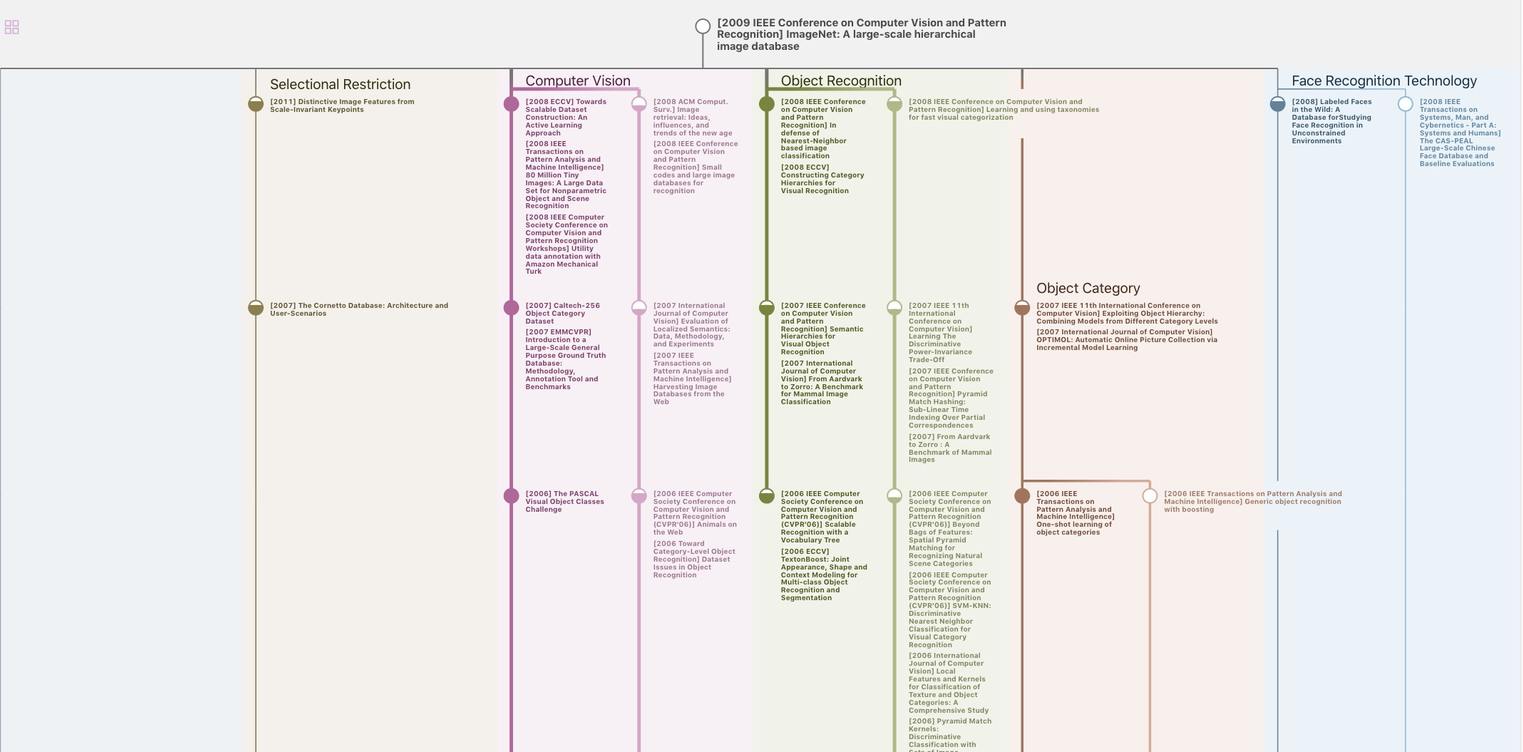
生成溯源树,研究论文发展脉络
Chat Paper
正在生成论文摘要