Decoy Meta–Clustering Through Rough Graded Possibilistic C-Medoids
2020 IEEE Conference on Evolving and Adaptive Intelligent Systems (EAIS)(2020)
摘要
Current ab initio methods for structure-prediction of proteins explore multiple simulated conformations, called decoys, to generate families of folds, one of which is the closest to the native one. To limit the exploration of the conformational space, clustering algorithms are routinely applied to group similar decoys and then finding the most plausible cluster centroid, based on the hypothesis that there are more low-energy conformations surrounding the native fold than the others; nevertheless different clustering algorithms, or different parameters, are likely to output different partitions of the input data and choosing only one of the possible solutions can be too restrictive and unreliable. meta-clustering algorithms allow to reconcile multiple clustering solutions by grouping them into meta-clusters (i.e. clusters of clusterings), so that similar partitions are grouped in the same meta-cluster. In this paper the use of meta-clustering is proposed for the selection of lowest energy decoys, testing the Rough Graded Possibilistic c-medoids clustering algorithm for both baseline clustering and meta-clustering. Preliminary tests on real data suggest that meta-clustering is effective in reducing the sensitivity to parameters of the clustering algorithm and to expand the explored space.
更多查看译文
关键词
Decoy Meta–Clustering,Rough Graded Possibilistic,C-Medoids
AI 理解论文
溯源树
样例
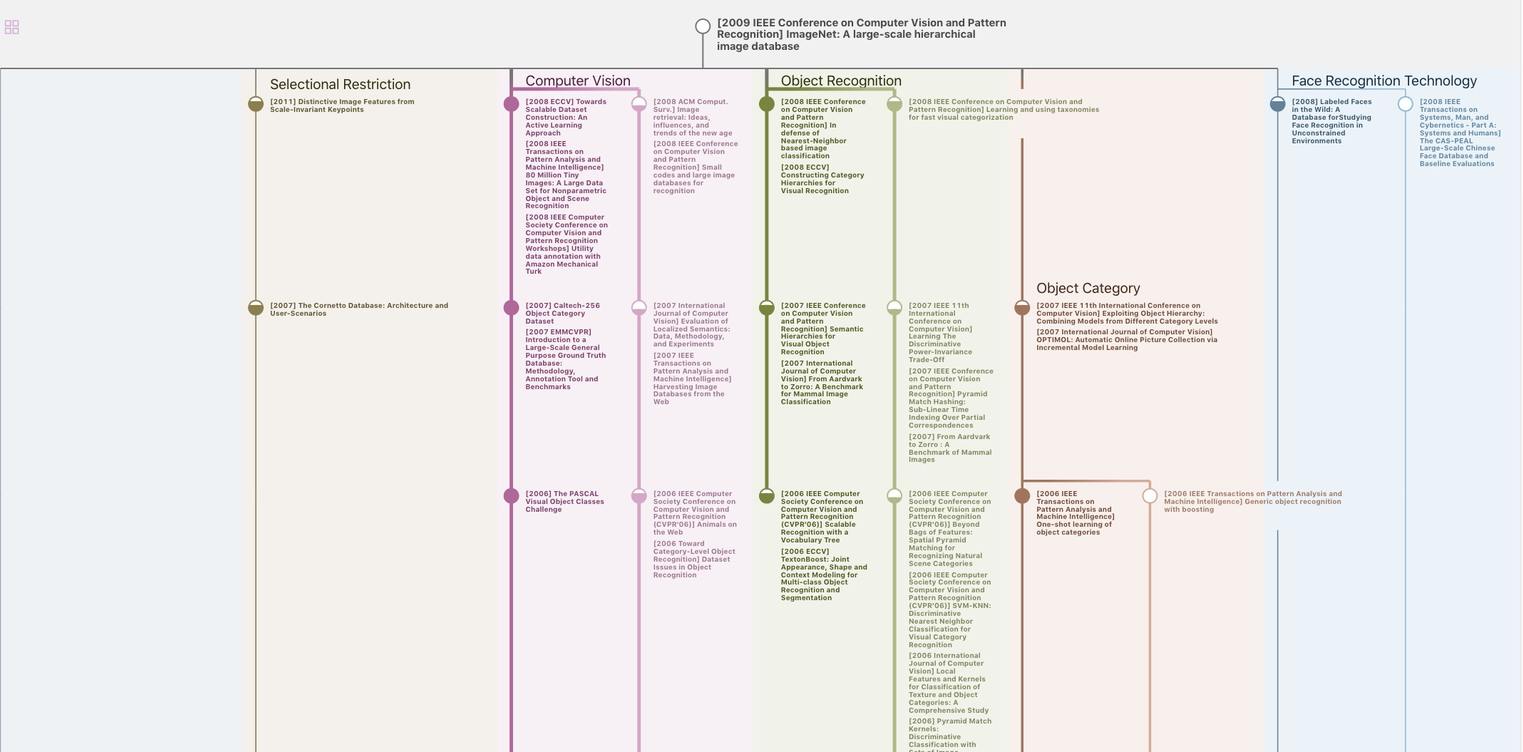
生成溯源树,研究论文发展脉络
Chat Paper
正在生成论文摘要