End-To-End Learned Image Compression With Fixed Point Weight Quantization
2020 IEEE INTERNATIONAL CONFERENCE ON IMAGE PROCESSING (ICIP)(2020)
摘要
Learned image compression (LIC) has reached the traditional hand-crafted methods such as JPEG2000 and BPG in terms of the coding gain. However, the large model size of the network prohibits the usage of LIC on resource-limited embedded systems. This paper presents a LIC with 8-bit fixed-point weights. First, we quantize the weights in groups and propose a non-linear memory-free codebook. Second, we explore the optimal grouping and quantization scheme. Finally, we develop a novel weight clipping fine tuning scheme. Experimental results illustrate that the coding loss caused by the quantization is small, while around 75% model size can be reduced compared with the 32-bit floating-point anchor. As far as we know, this is the first work to explore and evaluate the LIC fully with fixed-point weights, and our proposed quantized LIC is able to outperform BPG in terms of MS-SSIM.
更多查看译文
关键词
Image compression, neural networks, quantization, fixed-point, fine-tuning
AI 理解论文
溯源树
样例
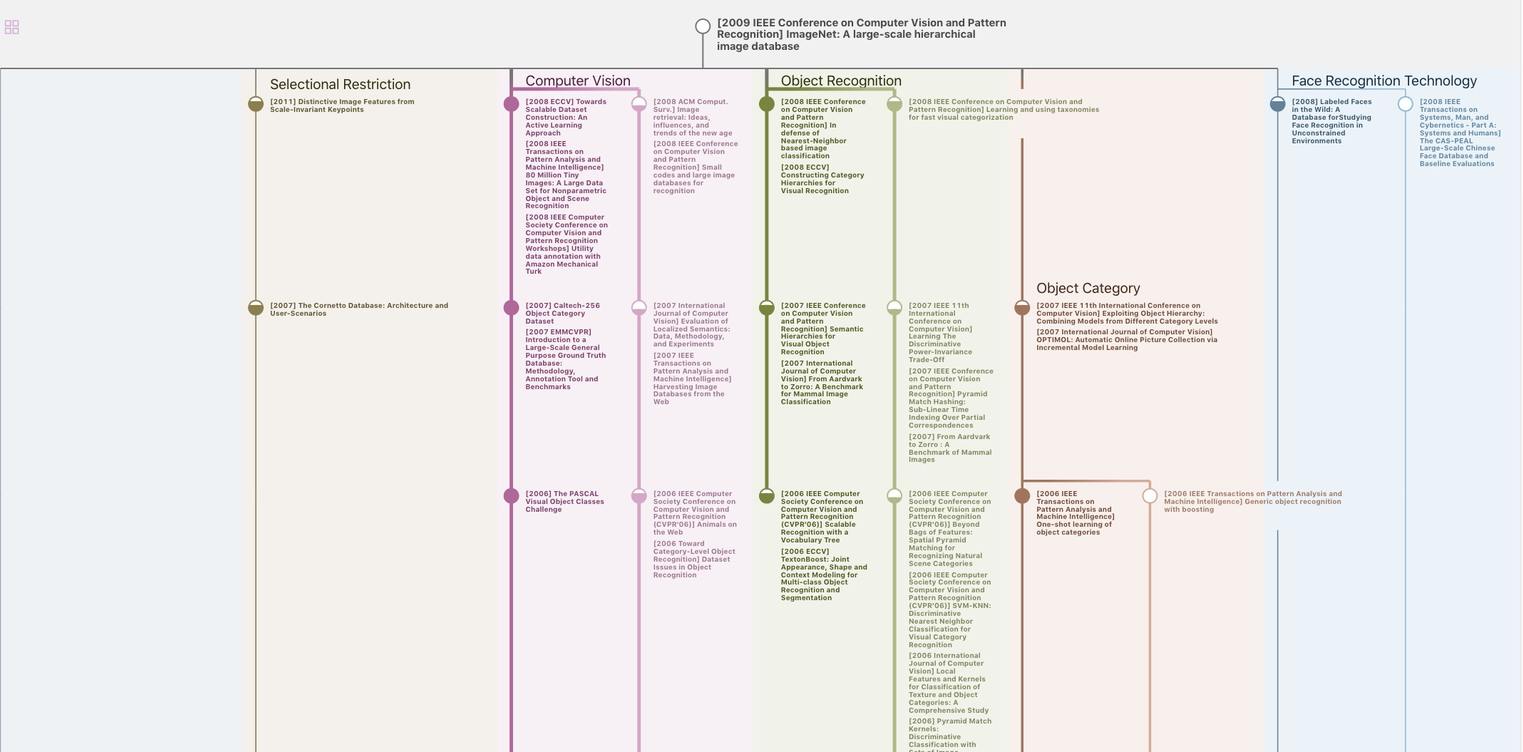
生成溯源树,研究论文发展脉络
Chat Paper
正在生成论文摘要