PointMask: Towards Interpretable and Bias-Resilient Point Cloud Processing
arxiv(2020)
摘要
Deep classifiers tend to associate a few discriminative input variables with their objective function, which in turn, may hurt their generalization capabilities. To address this, one can design systematic experiments and/or inspect the models via interpretability methods. In this paper, we investigate both of these strategies on deep models operating on point clouds. We propose PointMask, a model-agnostic interpretable information-bottleneck approach for attribution in point cloud models. PointMask encourages exploring the majority of variation factors in the input space while gradually converging to a general solution. More specifically, PointMask introduces a regularization term that minimizes the mutual information between the input and the latent features used to masks out irrelevant variables. We show that coupling a PointMask layer with an arbitrary model can discern the points in the input space which contribute the most to the prediction score, thereby leading to interpretability. Through designed bias experiments, we also show that thanks to its gradual masking feature, our proposed method is effective in handling data bias.
更多查看译文
关键词
pointmask,interpretable,bias-resilient
AI 理解论文
溯源树
样例
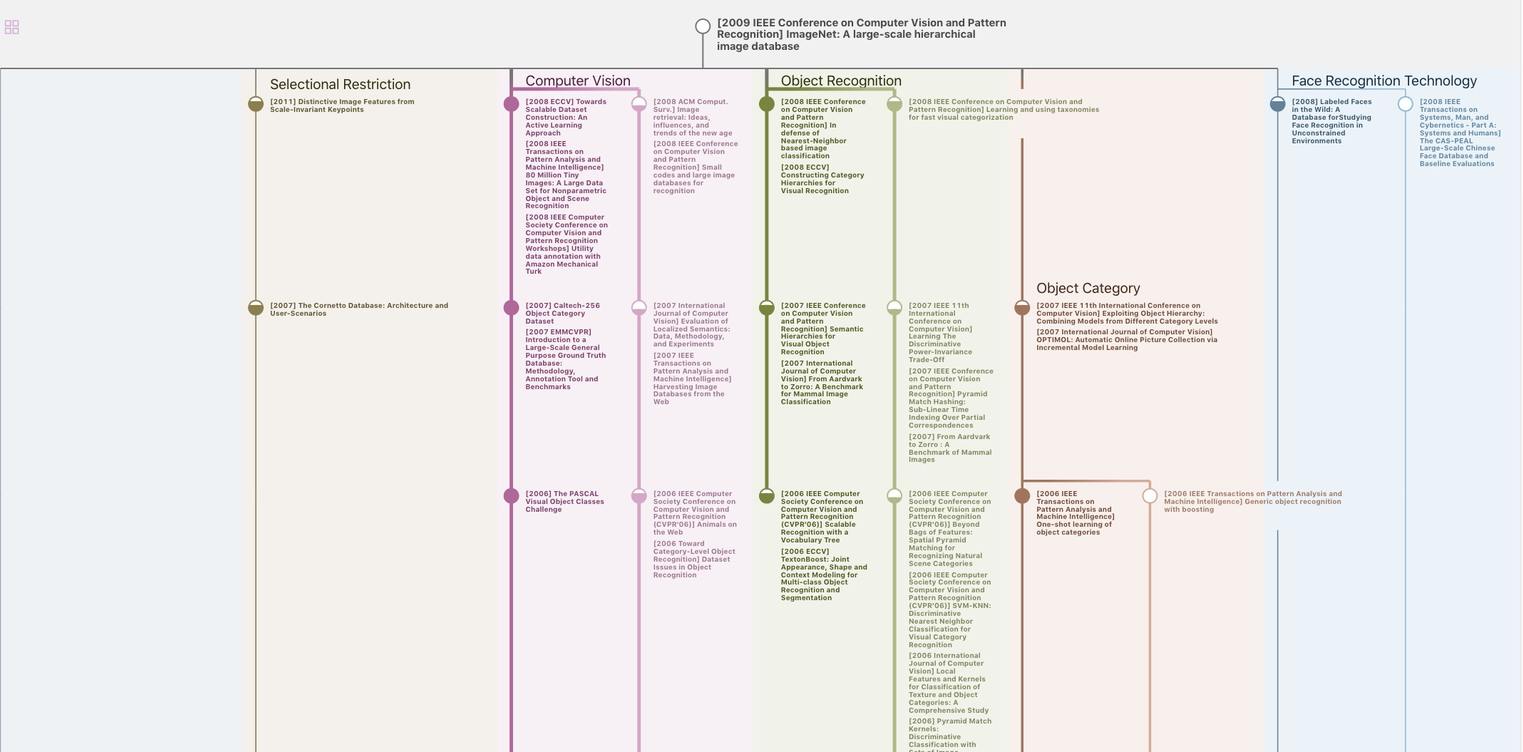
生成溯源树,研究论文发展脉络
Chat Paper
正在生成论文摘要