RiderS: Towards a Privacy-Aware Decentralized Self-Driving Ride-sharing Ecosystem
2020 IEEE International Conference on Decentralized Applications and Infrastructures (DAPPS)(2020)
摘要
Computer vision, machine learning, and decen-tralized applications are paving the way for a future where autonomous systems will be able to truly interact without humans in the loop. Though in its infancy, self-driving technologies are a prime example of this phenomena. This paper proposes RiderS, a novel privacy-first decentralized self-driving ride-sharing ecosystem, which consists of everything needed to allow autonomous/self-driving vehicles to participate in a decentralized ride-sharing economy. At its core, RiderS relies on a self-sovereign biometric solution, which leverages computer vision to register and authenticate users via a privacy-first biometric authentication algorithm. We then propose a novel decentralized architecture to support a self-sustained ride-sharing ecosystem. We propose a novel proof-of-matching geo-aware consensus algorithm, which is used to validate, and reward self-driving vehicles. The key concept being that each vehicle leverages its built-in compute power to assist in verifying transactions in order to keep the ecosystem in check while being rewarded for the work. Experimental results show that we can perform about 10K Matches per second (KMs) using an NVIDIA Jetson AGX Xavier development kit. We analyzed the privacy aspects as well as the accuracy of our biometric solution with good matching rates. Furthermore, we evaluated the performance and scalability of our proposed consensus algorithm and compared it to similar proof-of-work based blockchains yielding promising results. We modeled our ride-sharing ecosystem using published statistics from Uber and show that we can support Uber-scale ride-sharing using our geo-location aware consensus algorithm through a what-if simulated exercise.
更多查看译文
关键词
self-driving,decentralized,blockchain,identity,biometrics,cancelable
AI 理解论文
溯源树
样例
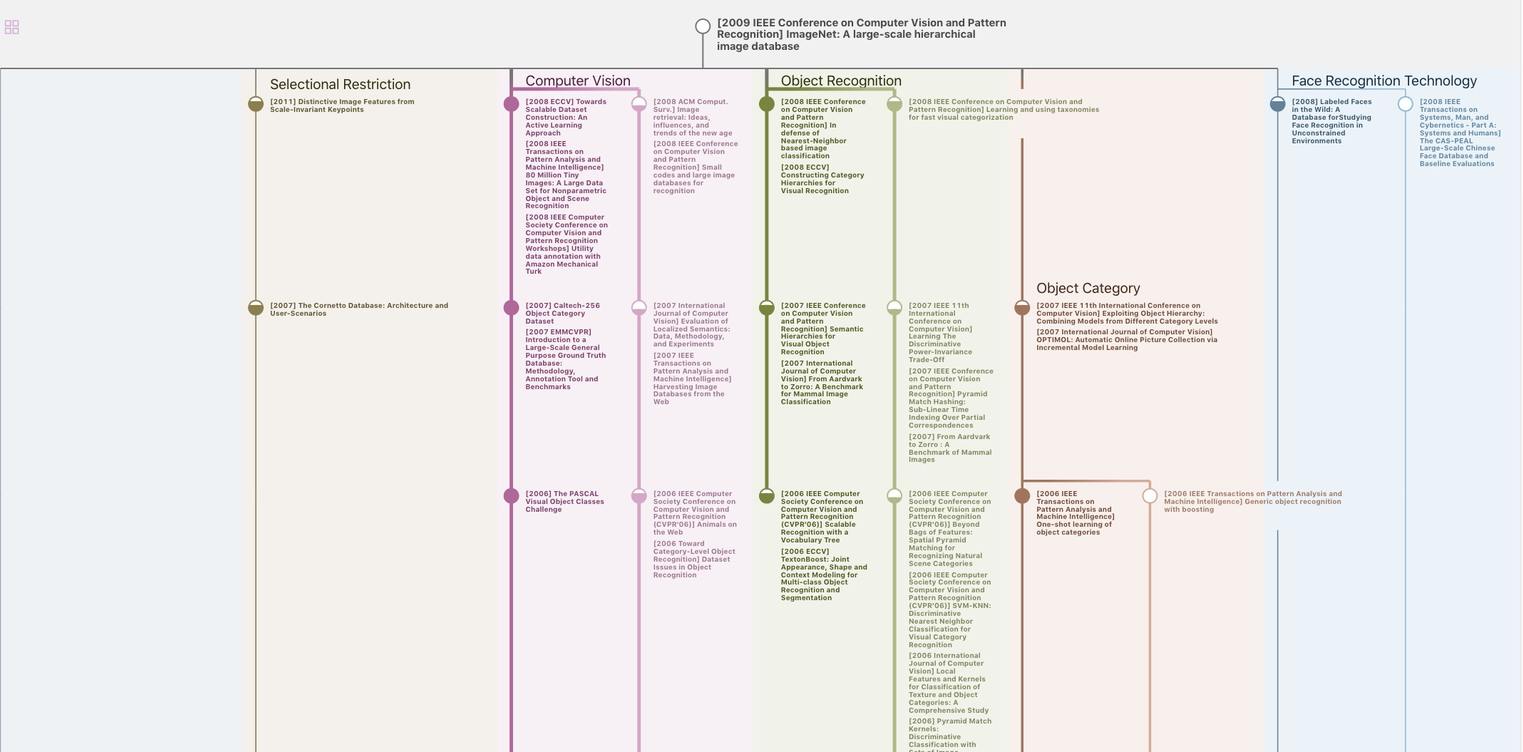
生成溯源树,研究论文发展脉络
Chat Paper
正在生成论文摘要