Automatic Segmentation Of Spinal Ultrasound Landmarks With U-Net Using Multiple Consecutive Images For Input
MEDICAL IMAGING 2020: IMAGE-GUIDED PROCEDURES, ROBOTIC INTERVENTIONS, AND MODELING(2021)
摘要
PURPOSE: Scoliosis screening is currently only implemented in a few countries due to the lack of a safe and accurate measurement method. Spinal ultrasound is a viable alternative to X-ray, but manual annotation of images is difficult and time consuming. We propose using deep learning through a U-net neural network that takes consecutive images per individual input, as an enhancement over using single images as input for the neural network.METHODS: Ultrasound data was collected from nine healthy volunteers. Images were manually segmented. To accommodate for consecutive input images, the ultrasound images were exported along with previous images stacked to serve as input for a modified U-net. Resulting output segmentations were evaluated based on the percentage of true negative and true positive pixel predictions.RESULTS: After comparing the single to five-image input arrays, the three-image input had the best performance in terms of true positive value. The single input and three-input images were then further tested. The single image input neural network had a true negative rate of 99.79%, and a true positive rate of 63.56%. The three-image input neural network had a true negative rate of 99.75%, and a true positive rate of 66.64%.CONCLUSION: The three-image input network outperformed the single input network in terms of the true positive rate by 3.08%. These findings suggest that using two additional input images consecutively preceding the original image assist the neural network in making more accurate predictions.
更多查看译文
AI 理解论文
溯源树
样例
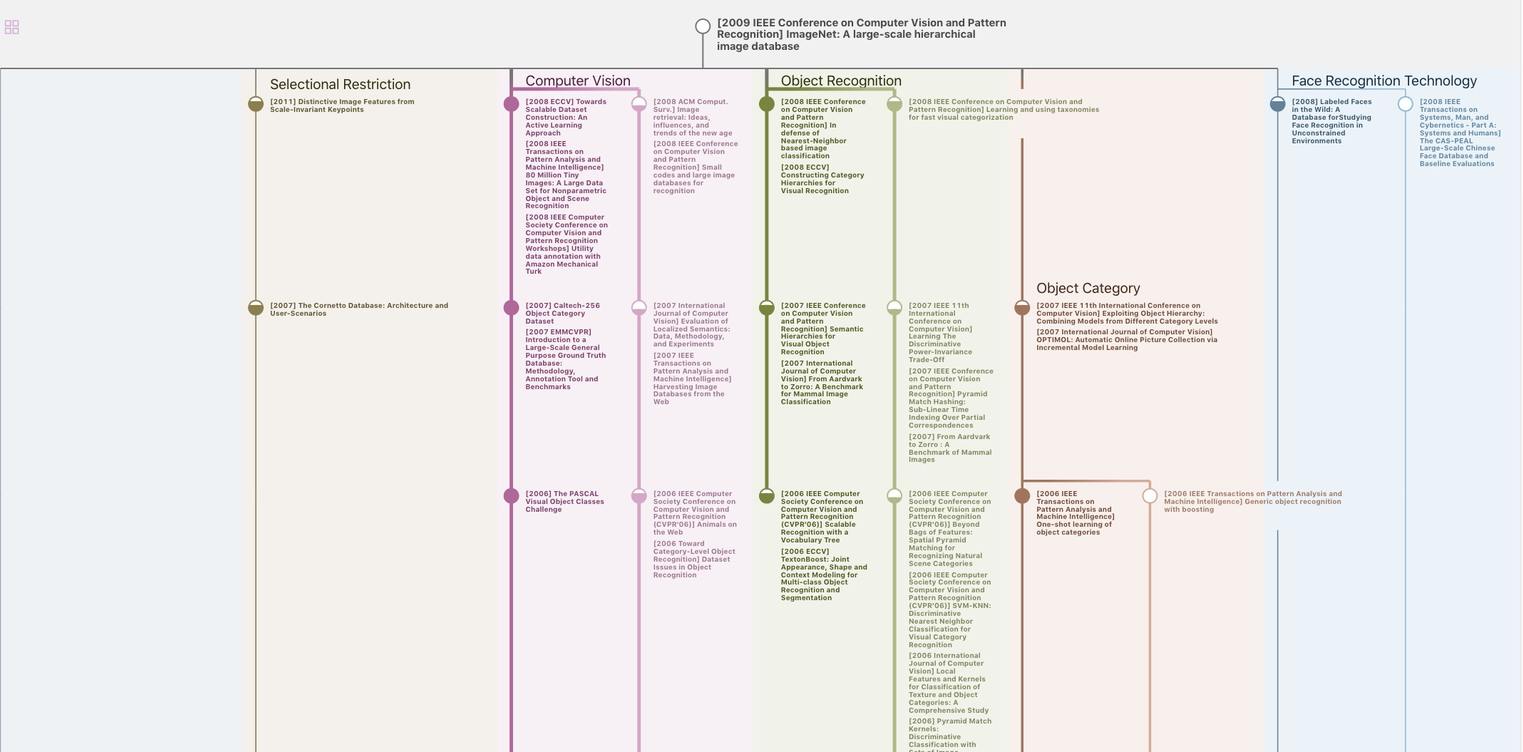
生成溯源树,研究论文发展脉络
Chat Paper
正在生成论文摘要