Ultrasound Image Simulation With Generative Adversarial Network
MEDICAL IMAGING 2020: IMAGE-GUIDED PROCEDURES, ROBOTIC INTERVENTIONS, AND MODELING(2021)
摘要
PURPOSE: It is difficult to simulate realistic ultrasound images due to the complexity of acoustic artifacts that contribute to a real ultrasound image. We propose to evaluate the realism of ultrasound images simulated using a generative adversarial network.METHODS: To achieve our goal, kidney ultrasounds were collected, and relevant anatomy was segmented to create anatomical label-maps using 3D Slicer. Adversarial networks were trained to generate ultrasound images from these label maps. Finally, a two-part survey of 4 participants with sonography experience was conducted to assess the realism of the generated images. The first part of the survey consisted of 50 kidney ultrasound images; half of which were real while the other half were simulated. Participants were asked to label each of the 50 ultrasound images as either real or simulated. In the second part of the survey, the participants were presented with ten simulated images not included in the first part of the survey and asked to evaluate the realism of the images.RESULTS: The average number of correctly identified images was 28 of 50 (56%). On a scale of 1-5, where 5 is indistinguishable from real US, the generated images received an average score of 3.75 for realistic anatomy and 4.0 for realistic ultrasound effects.CONCLUSIONS: We evaluated the realism of kidney ultrasound images generated using adversarial networks. Generative adversarial networks appear to be a promising method of simulating realistic ultrasound images from cross-sectional anatomical label-maps.
更多查看译文
关键词
Generative Adversarial Networks, Machine Learning, Ultrasound, Segmentation, 3D Slicer, SlicerIGT, PLUS Toolkit
AI 理解论文
溯源树
样例
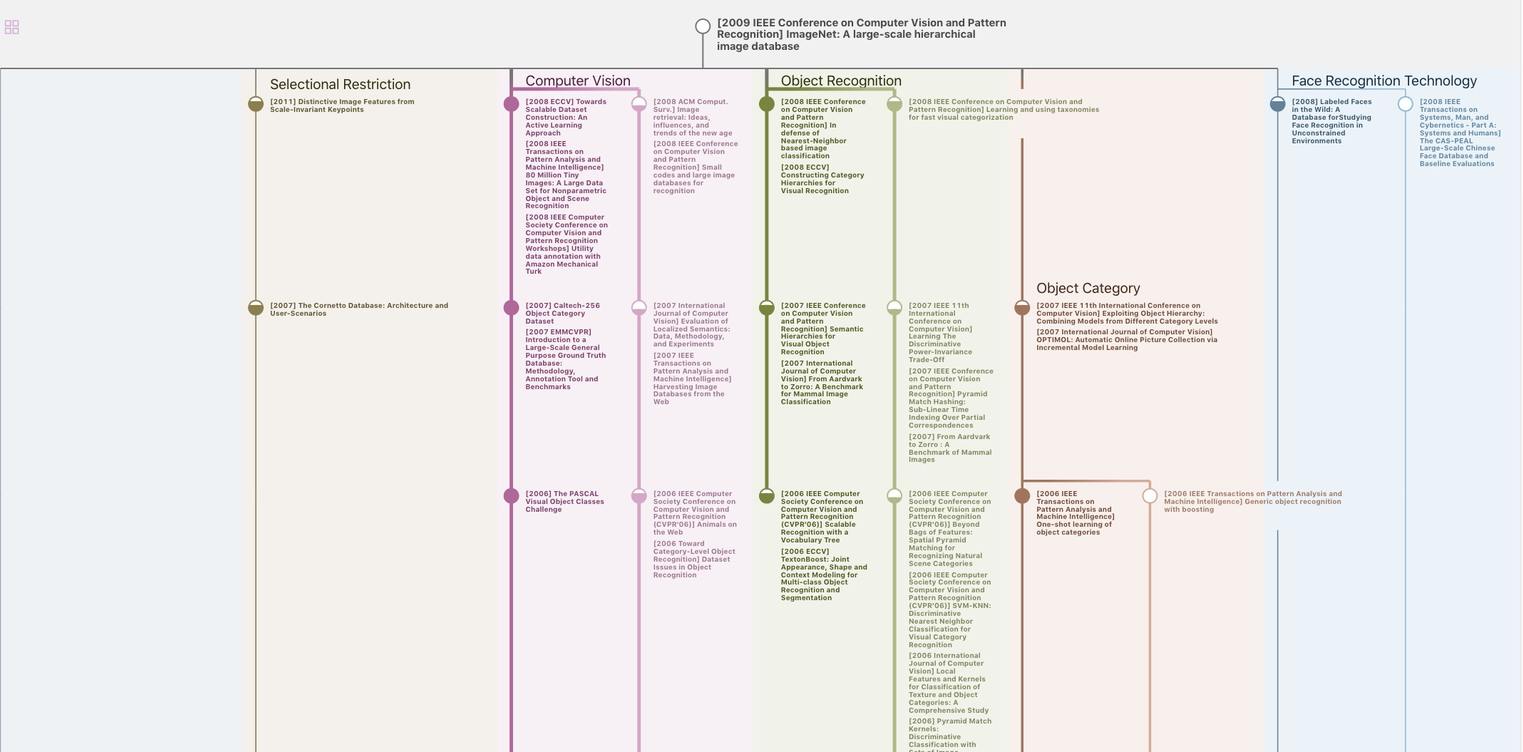
生成溯源树,研究论文发展脉络
Chat Paper
正在生成论文摘要