J3R: Joint Multi-task Learning of Ratings and Review Summaries for Explainable Recommendation
Joint European Conference on Machine Learning and Knowledge Discovery in Databases(2019)
摘要
We learn user preferences from ratings and reviews by using multi-task learning (MTL) of rating prediction and summarization of item reviews. Reviews of an item tend to describe detailed user preferences (eg, the cast, genre, or screenplay of a movie). A summary of such a review or a rating describes an overall user experience of the item. Our objective is to learn latent vectors which are shared across rating prediction and review summary generation. Additionally, the learned latent vectors and the generated summary act as explanations for the recommendation. Our MTL-based approach J3R uses a multi-layer perceptron for rating prediction, combined with pointer-generator networks with attention mechanism for the summarization component. We provide empirical evidence for joint learning of rating prediction and summary generation being beneficial for recommendation by conducting experiments on the Yelp …
更多查看译文
关键词
Personalized recommendation, Summarization, Natural Language Processing, Explainable AI
AI 理解论文
溯源树
样例
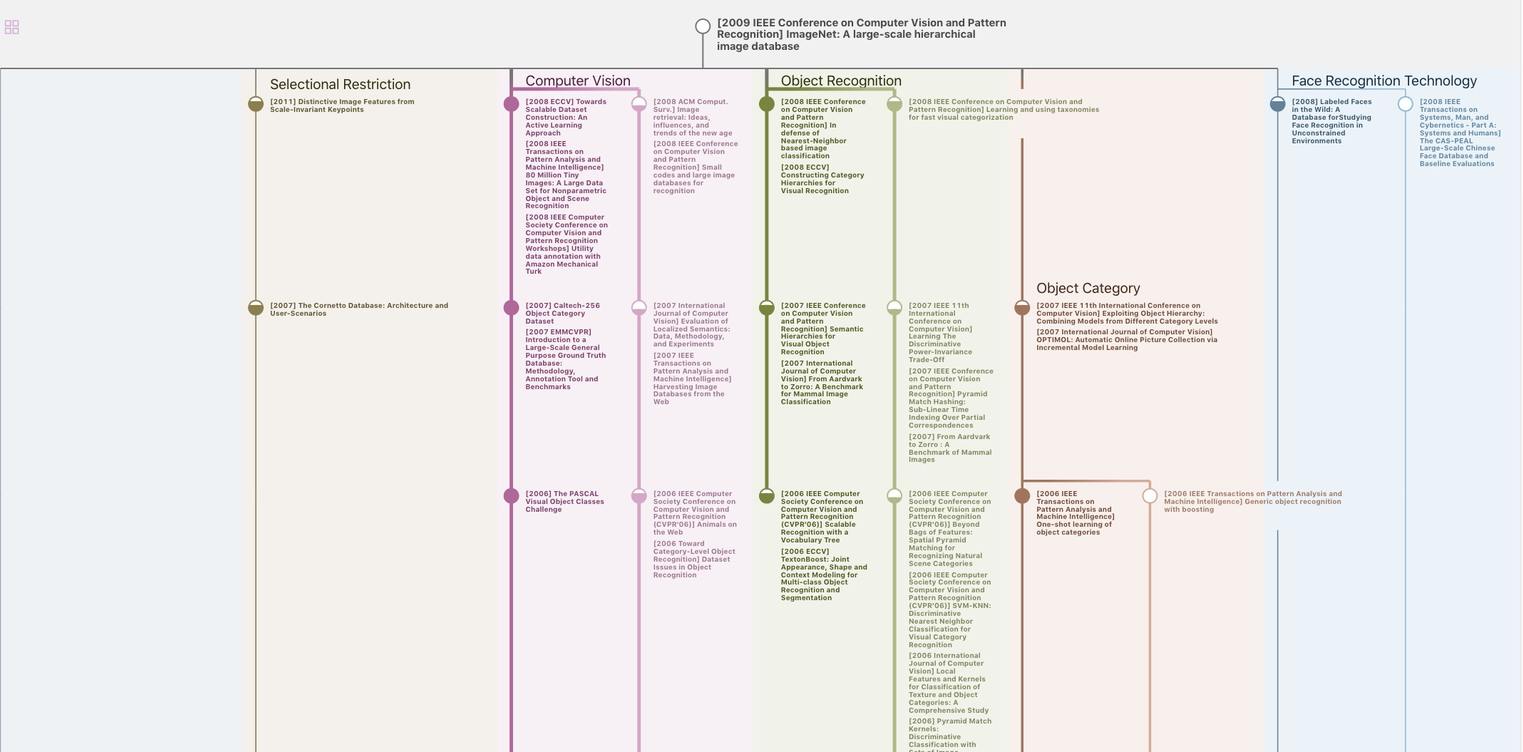
生成溯源树,研究论文发展脉络
Chat Paper
正在生成论文摘要