Asymptotic Errors for High-Dimensional Convex Penalized Linear Regression beyond Gaussian Matrices
COLT 2020(2020)
Abstract
We consider the problem of learning a coefficient vector $\bf x_0 \in \mathbb R^N$ from noisy linear observations $\mathbf{y} = \mathbf{F}{\mathbf{x}_{0}}+\mathbf{w} \in \mathbb R^M$ in high dimensional limit $M,N \to \infty$ with $\alpha \equiv M/N$ fixed. We provide a rigorous derivation of an explicit formula —first conjectured using heuristics method from statistical physics— for the asymptotic mean squared error obtained by penalized convex estimators such as the LASSO or the elastic net, for a sequence of very generic random matrix $\mathbf{F}$ corresponding to rotationally invariant data matrices of arbitrary spectrum. The proof is based on a convergence analysis of an oracle version of vector approximate message-passing (oracle-VAMP) and on the properties of its state evolution equations. Our method leverages on and highlights the link between vector approximate message-passing, Douglas-Rachford splitting and proximal descent algorithms, extending previous results obtained with i.i.d. matrices for a large class of problems. We illustrate our results on some concrete examples and show that even though they are asymptotic, our predictions agree remarkably well with numerics even for very moderate sizes.
MoreTranslated text
Key words
asymptotic errors,linear regression,gaussian matrices,convex,high-dimensional
AI Read Science
Must-Reading Tree
Example
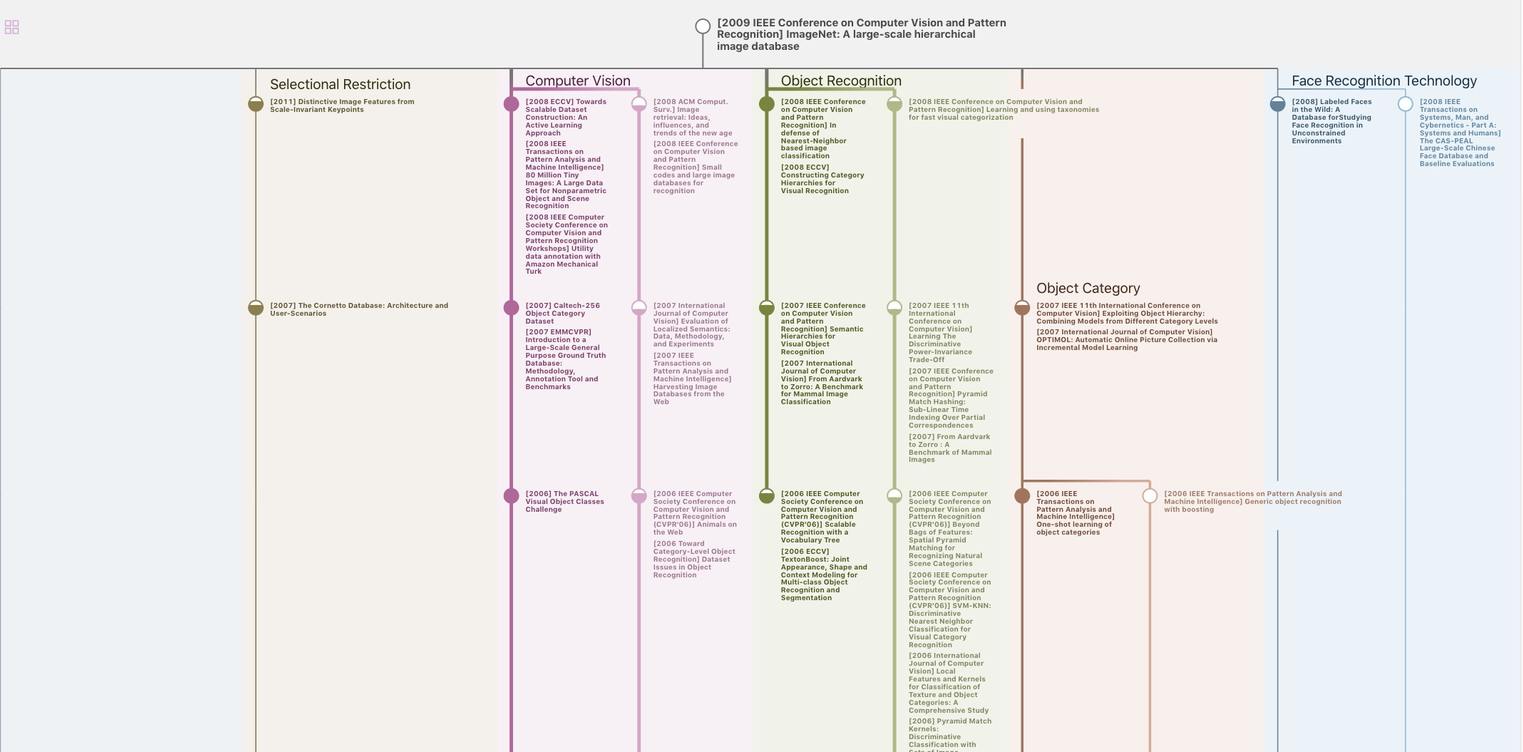
Generate MRT to find the research sequence of this paper
Chat Paper
Summary is being generated by the instructions you defined