Regional Image Perturbation Reduces $L_p$ Norms of Adversarial Examples While Maintaining Model-to-model Transferability
CoRR(2020)
摘要
Regional adversarial attacks often rely on complicated methods for generating adversarial perturbations, making it hard to compare their efficacy against well-known attacks. In this study, we show that effective regional perturbations can be generated without resorting to complex methods. We develop a very simple regional adversarial perturbation attack method using cross-entropy sign, one of the most commonly used losses in adversarial machine learning. Our experiments on ImageNet with multiple models reveal that, on average, $76\%$ of the generated adversarial examples maintain model-to-model transferability when the perturbation is applied to local image regions. Depending on the selected region, these localized adversarial examples require significantly less $L_p$ norm distortion (for $p \in \{0, 2, \infty\}$) compared to their non-local counterparts. These localized attacks therefore have the potential to undermine defenses that claim robustness under the aforementioned norms.
更多查看译文
关键词
adversarial examples,model-to-model
AI 理解论文
溯源树
样例
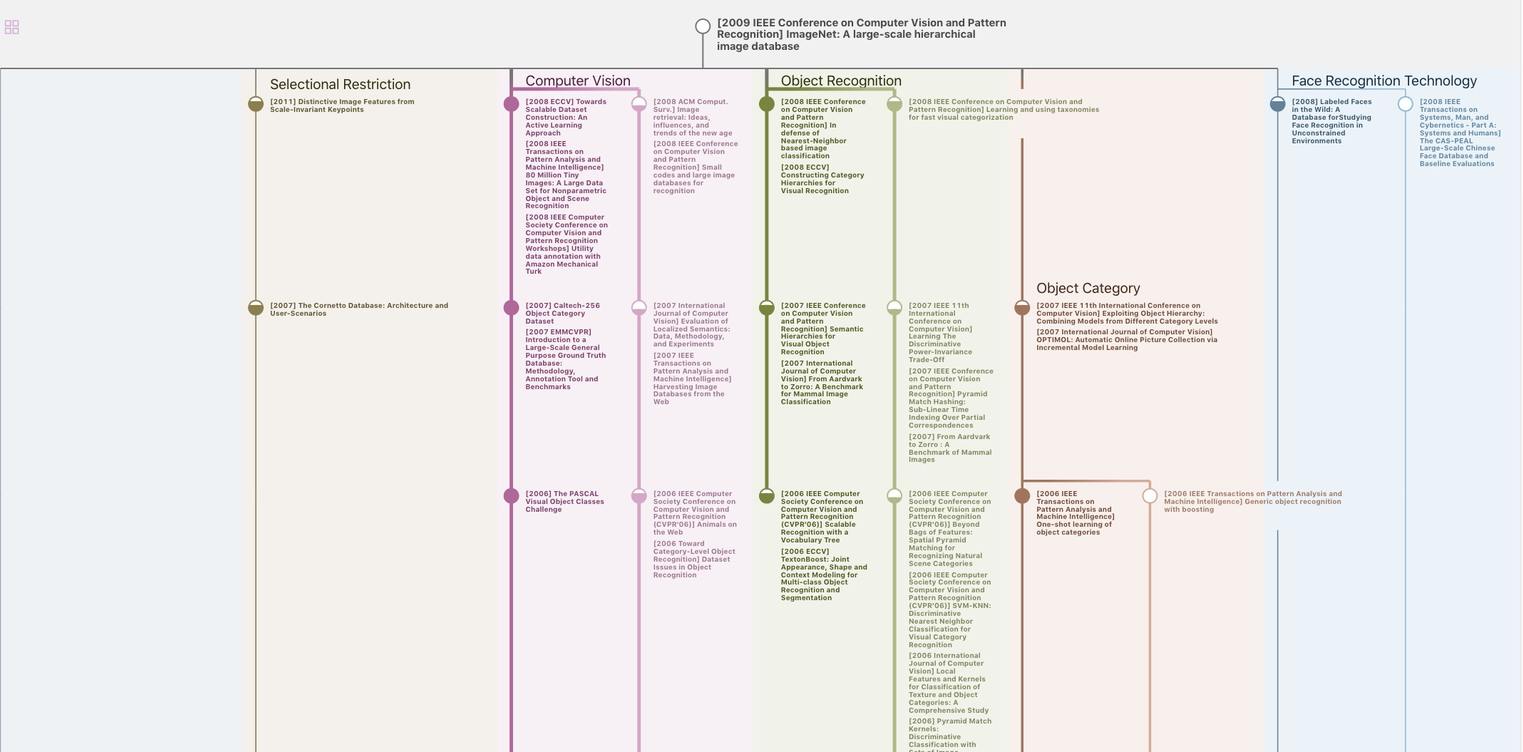
生成溯源树,研究论文发展脉络
Chat Paper
正在生成论文摘要