MTNet: a neural approach for cross-domain recommendation with unstructured text
KDD Deep Learning Day(2018)
摘要
Collaborative filtering (CF) is the key technique for recommender systems (RSs). CF exploits user-item behavior interactions (eg, clicks) only and suffers from the data sparsity issue. One solution is to integrate the content information such as product reviews and news titles, leading to hybrid filtering methods. Another solution is to transfer knowledge from a related source domain such as improving the movie recommendation with the knowledge of the book domain, leading to cross-domain methods where transfer learning is a key technique. In real life, no single service can satisfy a user’s all information needs. Thus it motivates us to exploit information from both the content and across domains for RSs in this paper. We achieve this by developing approaches to capture the text content and to transfer cross-domain knowledge. We propose a novel neural model, MTNet (“M” for memory and “T” for transfer), for cross-domain recommendation with unstructured text in an end-to-end manner. MTNet can attentively extract useful content via a memory network (MNet) and can selectively transfer knowledge from across domains by a transfer network (TNet), a novel network. The principle underlying these two components is the neural attention mechanism. A shared layer of feature interactions is stacked on the top to couple the high-level representations learned from individual networks. On two real-world datasets, MTNet shows better performance in terms of three ranking metrics by comparing with various baselines, including single/cross domain, shallow/deep, and hybrid methods. We conduct thorough analyses to understand how the text content and …
更多查看译文
AI 理解论文
溯源树
样例
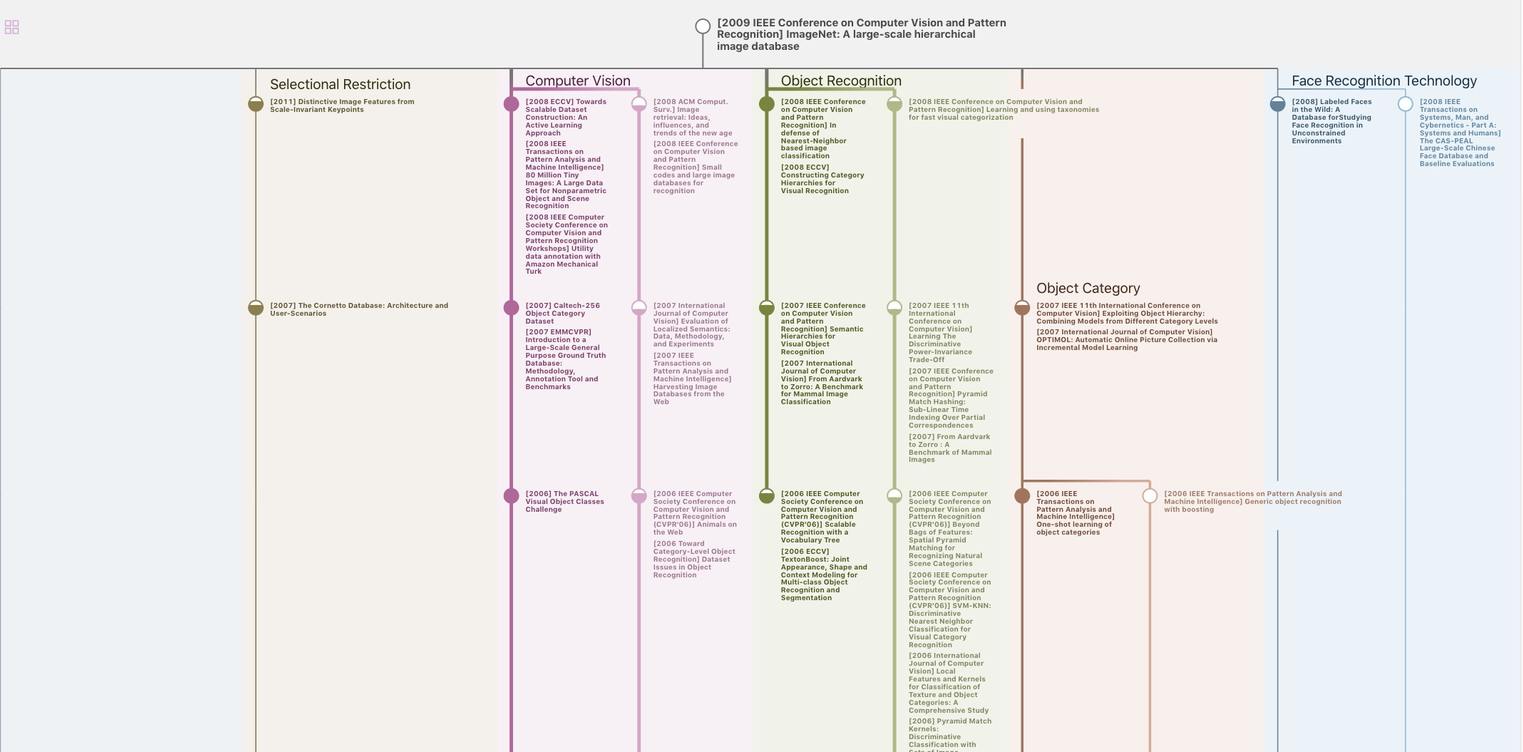
生成溯源树,研究论文发展脉络
Chat Paper
正在生成论文摘要