Data Poisoning Attacks against Differentially Private Recommender Systems
SIGIR '20: The 43rd International ACM SIGIR conference on research and development in Information Retrieval Virtual Event China July, 2020(2020)
摘要
Recommender systems based on collaborative filtering are highly vulnerable to data poisoning attacks, where a determined attacker injects fake users with false user-item feedback, with an objective to either corrupt the recommender system or promote/demote a target set of items. Recently, differential privacy was explored as a defense technique against data poisoning attacks in the typical machine learning setting. In this paper, we study the effectiveness of differential privacy against such attacks on matrix factorization based collaborative filtering systems. Concretely, we conduct extensive experiments for evaluating robustness to injection of malicious user profiles by simulating common types of shilling attacks on real-world data and comparing the predictions of typical matrix factorization with differentially private matrix factorization.
更多查看译文
关键词
Data Poisoning, Shilling Attacks, Differential Privacy, Matrix Factorization, Collaborative Filtering, Recommender Systems
AI 理解论文
溯源树
样例
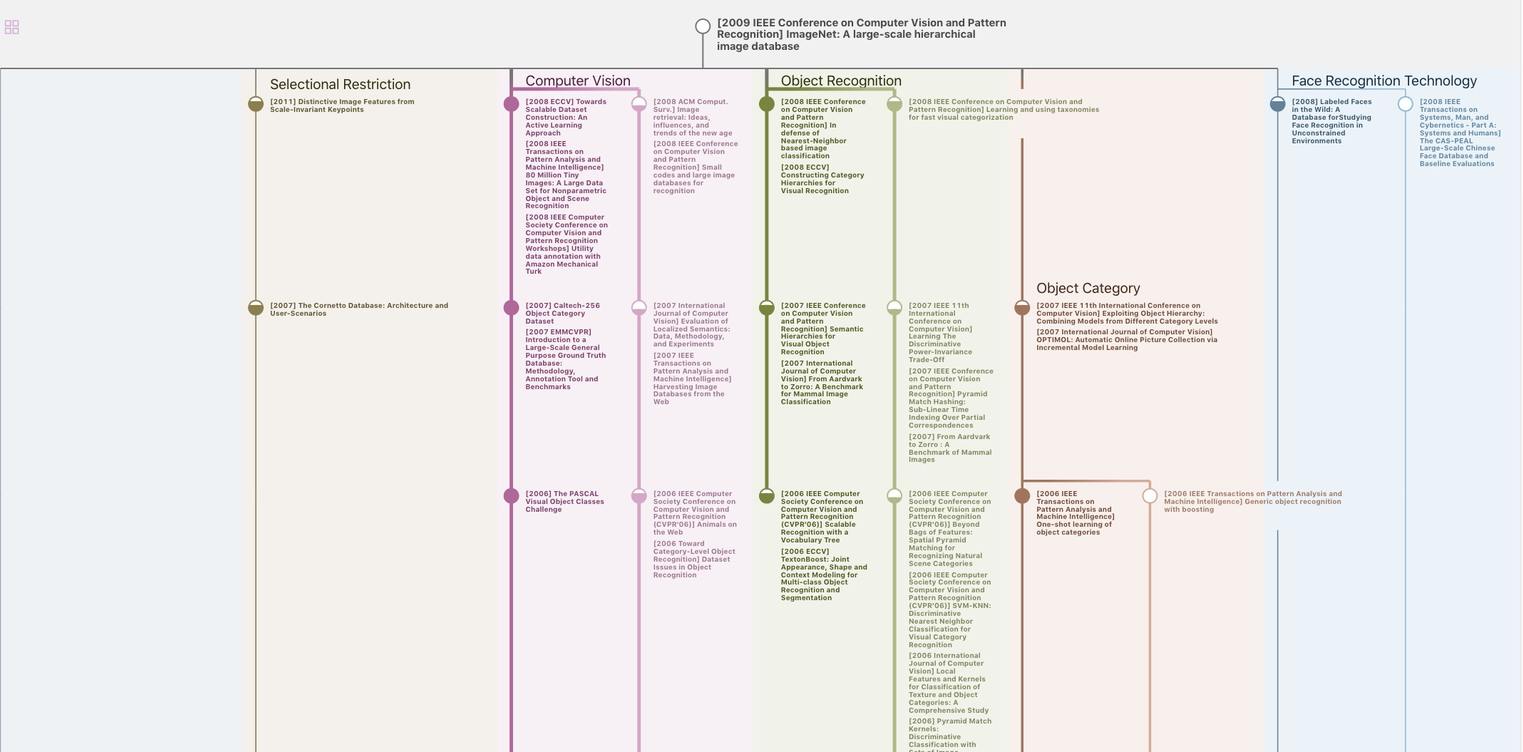
生成溯源树,研究论文发展脉络
Chat Paper
正在生成论文摘要