A Survey Of Network Embedding For Drug Analysis And Prediction
CURRENT PROTEIN & PEPTIDE SCIENCE(2021)
摘要
Traditional network-based computational methods have shown good results in drug analysis and prediction. However, these methods are time-consuming and lack universality, and it is difficult to exploit the auxiliary information of nodes and edges. Network embedding provides a promising way for alleviating the above problems by transforming the network into a low-dimensional space while preserving network structure and auxiliary information. This thus facilitates the application of machine learning algorithms for subsequent processing. Network embedding has been introduced into drug analysis and prediction in the last few years, and has shown superior performance over traditional methods. However, there is no systematic review of this issue. This article offers a comprehensive survey of the primary network embedding methods and their applications in drug analysis and prediction. The network embedding technologies applied in homogeneous network and heterogeneous network are investigated and compared, including matrix decomposition, random walk, and deep learning. Especially, the Graph neural network (GNN) methods in deep learning are highlighted. Furthermore, the applications of network embedding in drug similarity estimation, drug-target interaction prediction, adverse drug reactions prediction, protein function and therapeutic peptides prediction are discussed. Several future potential research directions are also discussed.
更多查看译文
关键词
Network embedding, drug discovery, drug similarity estimation, drug-target prediction, adverse drug reactions prediction, protein function and therapeutic peptides prediction
AI 理解论文
溯源树
样例
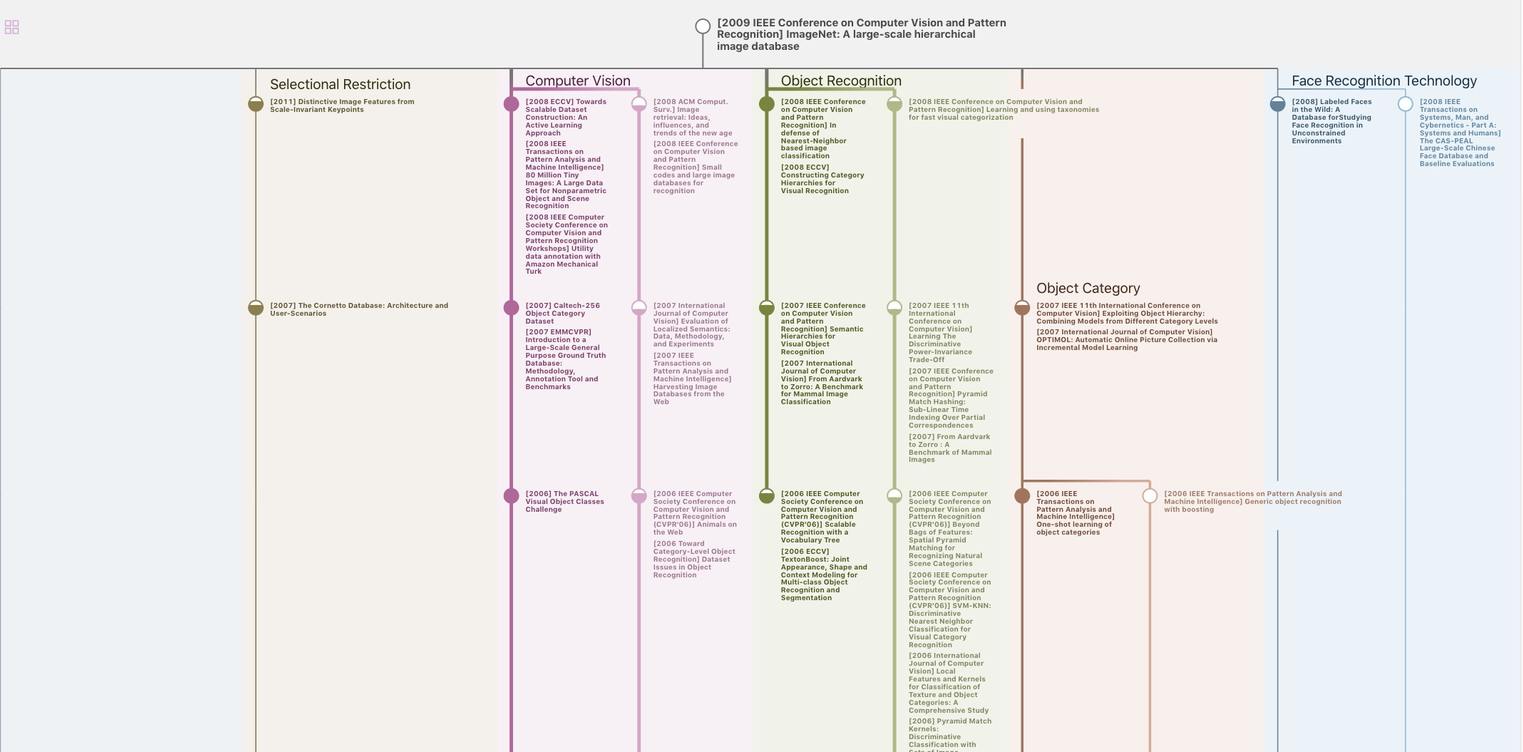
生成溯源树,研究论文发展脉络
Chat Paper
正在生成论文摘要