Sadroid: A Deep Classification Model For Android Malware Detection Based On Semantic Analysis
2020 IEEE WIRELESS COMMUNICATIONS AND NETWORKING CONFERENCE (WCNC)(2020)
摘要
Previous works have designed many deep learning models for Android malware detection using various features (e.g. permissions, APIs et.) to achieve better classification performance. However, these methods usually input each feature into the classifier independently and completely (using One-Hot Encoding) so that features are orthogonal to each other. This discrete representation is difficult to preserve the semantic information of features. In this paper, we design two feature segmentation methods to enhance the semantics of the features in preprocessing. Besides that, we propose a malware detection model that consists of a distributed representation process for Android features and an optimized convolutional neural network for classification, named Semantic Analysis Detection (SADroid). In SADroid, the distance between features with similar semantics is closer in vector space. It provides the semantic information of features to the classifier to improve the classification performance. In the evaluation, SADroid outperforms the advanced models in detection accuracy on a data set of 19,600 applications, while maintaining a low computational cost.
更多查看译文
关键词
Deep learning, Android malware detection, Semantic information, Distributed representation
AI 理解论文
溯源树
样例
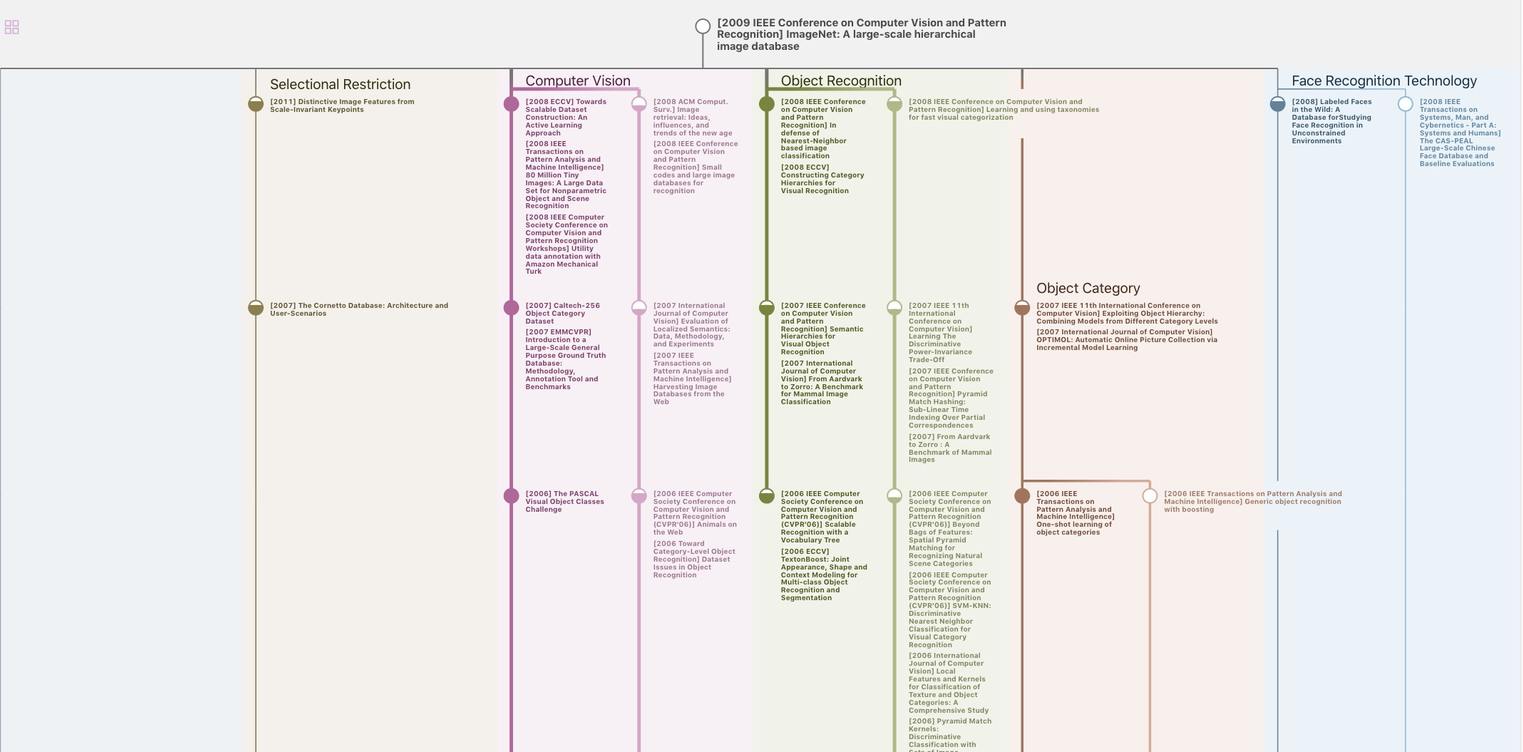
生成溯源树,研究论文发展脉络
Chat Paper
正在生成论文摘要