Accurate stacked-sheet counting method based on deep learning.
JOURNAL OF THE OPTICAL SOCIETY OF AMERICA A-OPTICS IMAGE SCIENCE AND VISION(2020)
Abstract
The accurate counting of laminated sheets, such as packing or printing sheets in industry, is extremely important because it greatly affects the economic cost. However, the different thicknesses, adhesion properties, and breakage points and the low contrast of sheets remain challenges to traditional counting methods based on image processing. This paper proposes a new stacked-sheet counting method with a deep learning approach using the U-Net architecture. A specific dataset according to the characteristics of stack side images is collected. The stripe of the center line of each sheet is used for semantic segmentation, and the complete side images of the slices are segmented via training with small image patches and testing with original large images. With this model, each pixel is classified by multi-layer convolution and deconvolution to determine whether it is the target object to be detected. After the model is trained, the test set is used to test the model, and a center region segmentation map based on the pixel points is obtained. By calculating the statistical median value of centerline points across different sections in these segmented images, the number of sheets can be obtained. Compared with traditional image algorithms in real product counting experiments, the proposed method can achieve better performance with higher accuracy and a lower error rate. (C) 2020 Optical Society of America
MoreTranslated text
Key words
deep learning,stacked-sheet
AI Read Science
Must-Reading Tree
Example
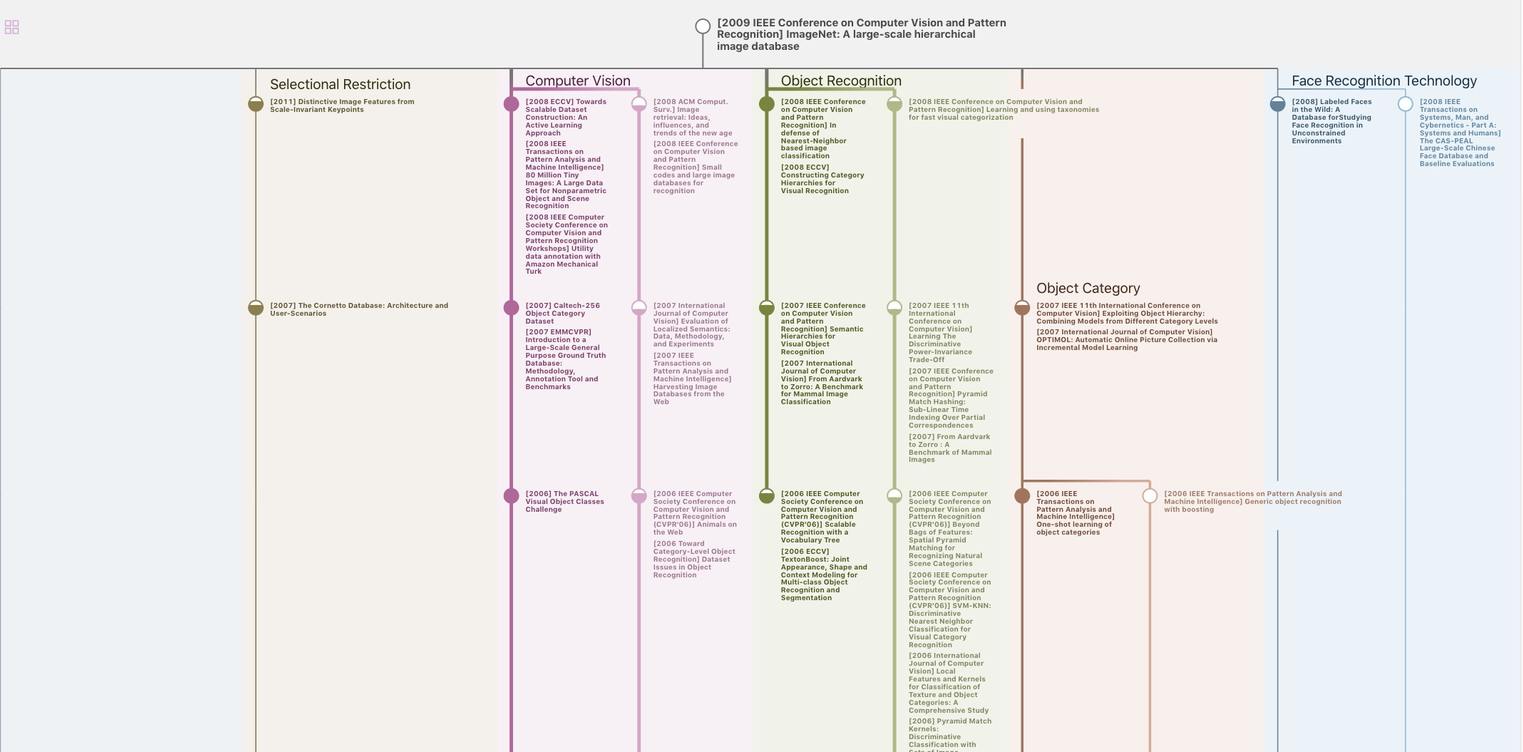
Generate MRT to find the research sequence of this paper
Chat Paper
Summary is being generated by the instructions you defined