Feature sensitivity criterion-based sampling strategy from the Optimization based on Phylogram Analysis (Fs-OPA) and Cox regression applied to mental disorder datasets.
PloS one(2020)
摘要
Digital datasets in several health care facilities, as hospitals and prehospital services, accumulated data from thousands of patients for more than a decade. In general, there is no local team with enough experts with the required different skills capable of analyzing them in entirety. The integration of those abilities usually demands a relatively long-period and is cost. Considering that scenario, this paper proposes a new Feature Sensitivity technique that can automatically deal with a large dataset. It uses a criterion-based sampling strategy from the Optimization based on Phylogram Analysis. Called FS-opa, the new approach seems proper for dealing with any types of raw data from health centers and manipulate their entire datasets. Besides, FS-opa can find the principal features for the construction of inference models without depending on expert knowledge of the problem domain. The selected features can be combined with usual statistical or machine learning methods to perform predictions. The new method can mine entire datasets from scratch. FS-opa was evaluated using a relatively large dataset from electronic health records of mental disorder prehospital services in Brazil. Cox's approach was integrated to FS-opa to generate survival analysis models related to the length of stay (LOS) in hospitals, assuming that it is a relevant aspect that can benefit estimates of the efficiency of hospitals and the quality of patient treatments. Since FS-opa can work with raw datasets, no knowledge from the problem domain was used to obtain the preliminary prediction models found. Results show that FS-opa succeeded in performing a feature sensitivity analysis using only the raw data available. In this way, FS-opa can find the principal features without bias of an inference model, since the proposed method does not use it. Moreover, the experiments show that FS-opa can provide models with a useful trade-off according to their representativeness and parsimony. It can benefit further analyses by experts since they can focus on aspects that benefit problem modeling.
更多查看译文
AI 理解论文
溯源树
样例
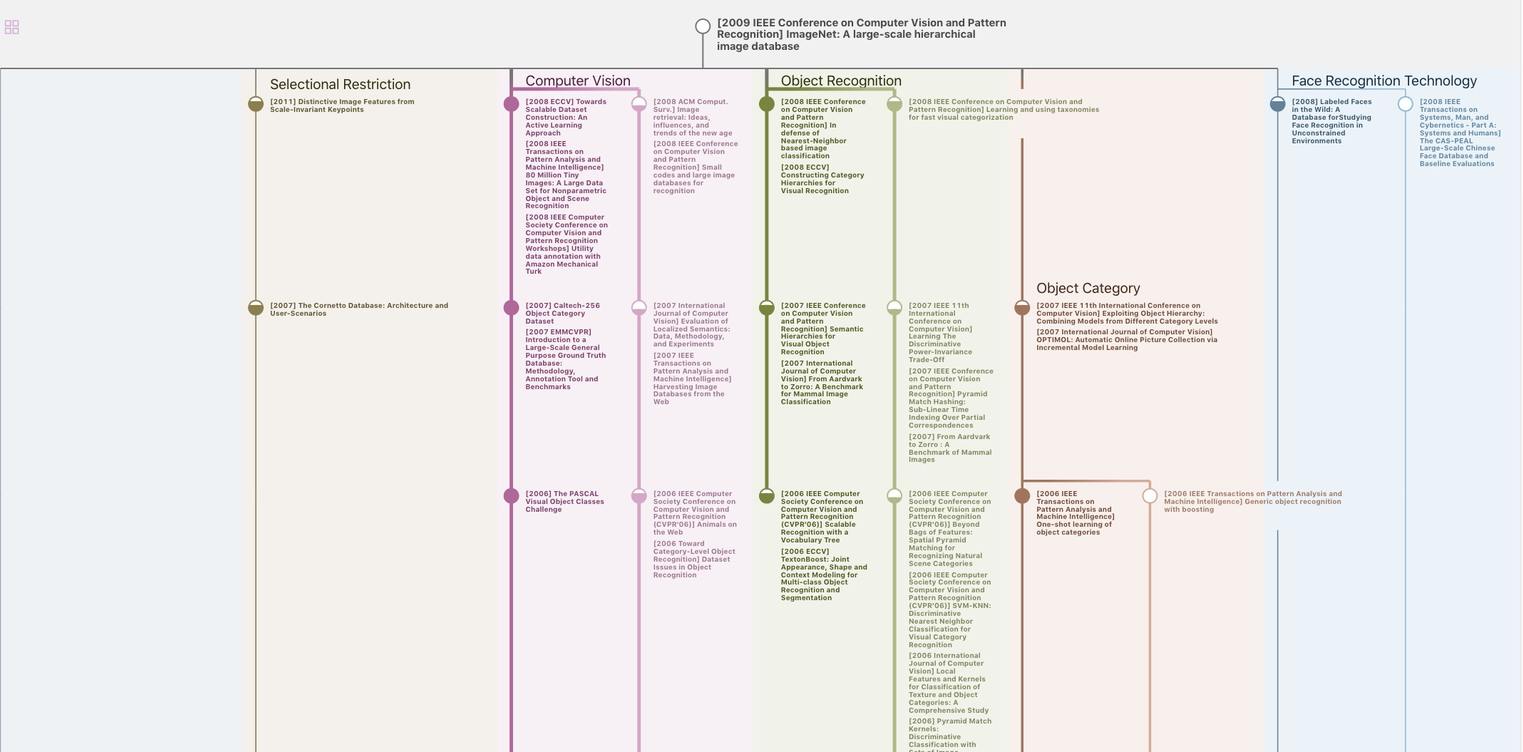
生成溯源树,研究论文发展脉络
Chat Paper
正在生成论文摘要