Light-field-depth-estimation network based on epipolar geometry and image segmentation.
JOURNAL OF THE OPTICAL SOCIETY OF AMERICA A-OPTICS IMAGE SCIENCE AND VISION(2020)
Abstract
In this paper, we propose a convolutional neural network based on epipolar geometry and image segmentation for light-field depth estimation. Epipolar geometry is utilized to estimate the initial disparity map. Multi-orientation epipolar images are selected as input data, and the convolutional blocks are adopted based on the disparity of different-direction epipolar images. Image segmentation is used to obtain the edge information of the central sub-aperture image. By concatenating the output of the two parts, an accurate depth map could be generated with fast speed. Our method achieves a high rank on most quality assessment metrics in the HCI 4D Light Field Benchmark and also shows effectiveness in estimating accurate depth on real-world light-field images. (C) 2020 Optical Society of America
MoreTranslated text
Key words
light-field-depth-estimation light-field-depth-estimation,epipolar geometry,image segmentation
AI Read Science
Must-Reading Tree
Example
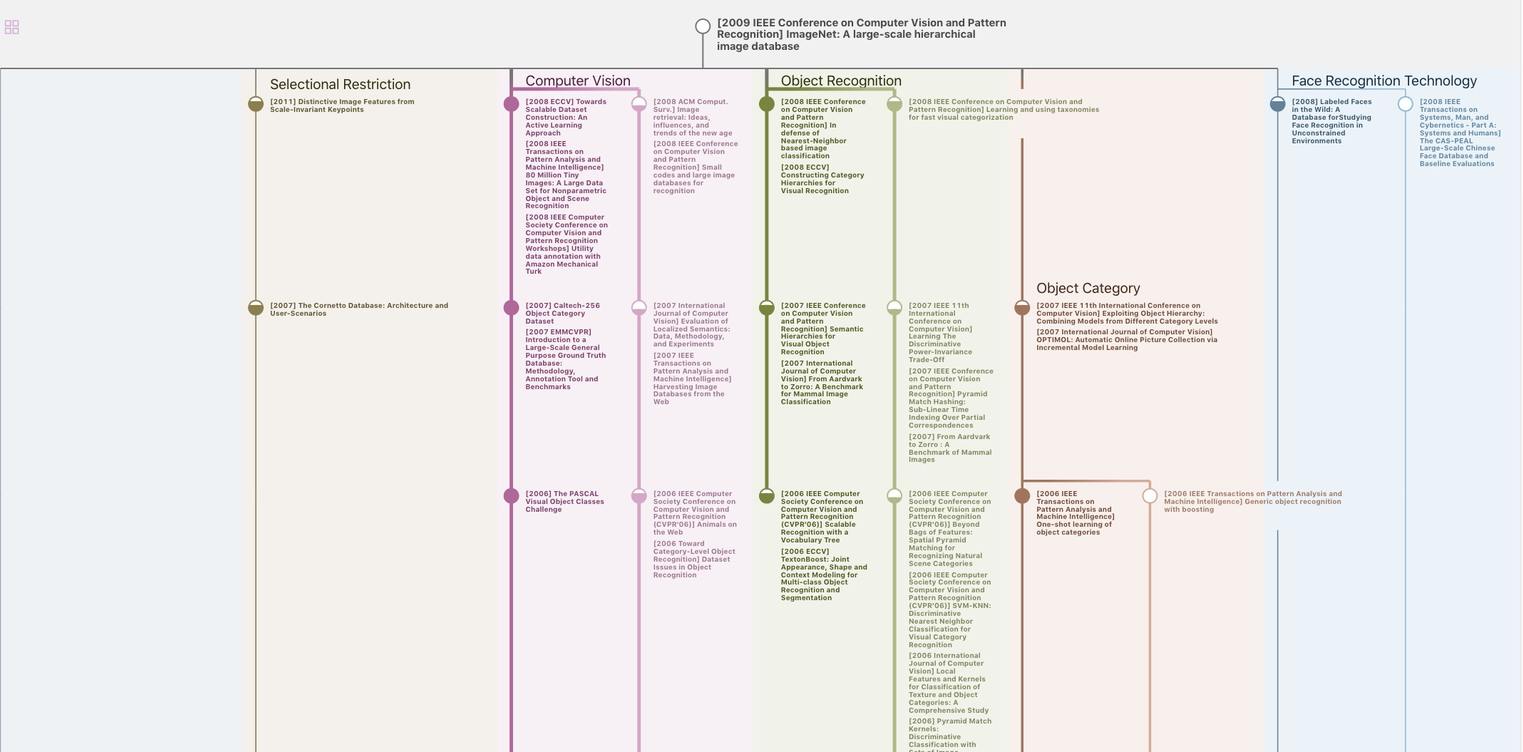
Generate MRT to find the research sequence of this paper
Chat Paper
Summary is being generated by the instructions you defined