A simple bipartite graph projection model for clustering in networks
arxiv(2020)
摘要
Graph datasets are frequently constructed by a projection of a bipartite graph, where two nodes are connected in the projection if they share a common neighbor in the bipartite graph; for example, a coauthorship graph is a projection of an author-publication bipartite graph. Analyzing the structure of the projected graph is common, but we do not have a good understanding of the consequences of the projection on such analyses. Here, we propose and analyze a random graph model to study what properties we can expect from the projection step. Our model is based on a Chung-Lu random graph for constructing the bipartite representation, which enables us to rigorously analyze the projected graph. We show that common network properties such as sparsity, heavy-tailed degree distributions, local clustering at nodes, the inverse relationship between node degree, and global transitivity can be explained and analyzed through this simple model. We also develop a fast sampling algorithm for our model, which we show is provably optimal for certain input distributions. Numerical simulations where model parameters come from real-world datasets show that much of the clustering behavior in some datasets can just be explained by the projection step.
更多查看译文
关键词
clustering,networks,graph
AI 理解论文
溯源树
样例
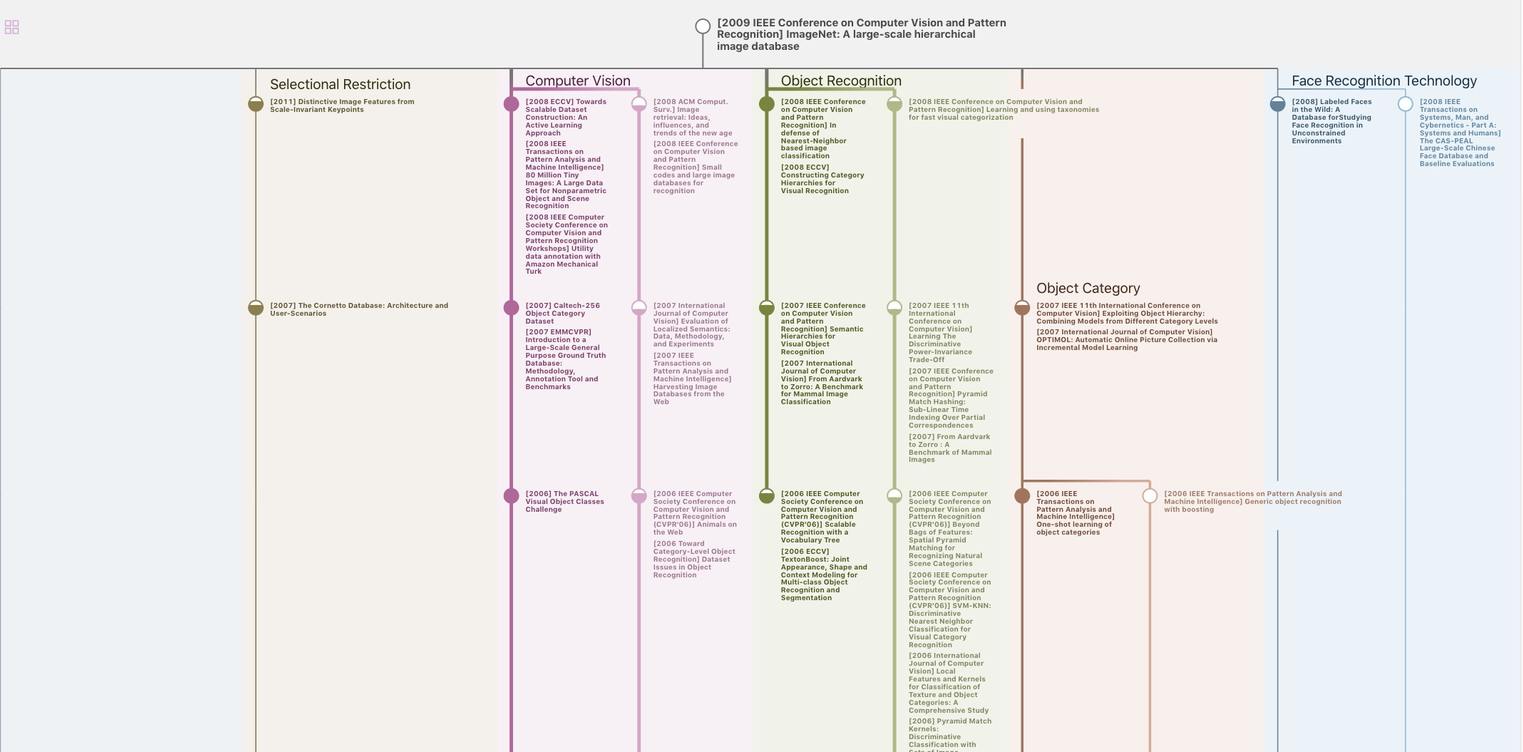
生成溯源树,研究论文发展脉络
Chat Paper
正在生成论文摘要