Multi-Layer Bilinear Generalized Approximate Message Passing
IEEE TRANSACTIONS ON SIGNAL PROCESSING(2021)
摘要
In this paper, we extend the bilinear generalized approximate message passing (BiG-AMP) approach, originally proposed for high-dimensional generalized bilinear regression, to the multi-layer case for the handling of cascaded problem such as matrix-factorization problem arising in relay communication among others. Assuming statistically independent matrix entries with known priors, the new algorithm called ML-BiGAMP could approximate the general sum-product loopy belief propagation (LBP) in the high-dimensional limit enjoying a substantial reduction in computational complexity. We demonstrate that, in large system limit, the asymptotic MSE performance of ML-BiGAMP could be fully characterized via a set of simple one-dimensional equations termed state evolution (SE). We establish that the asymptotic MSE predicted by ML-BiGAMP' SE matches perfectly the exact MMSE predicted by the replica method, which is well-known to be Bayes-optimal but infeasible in practice. This consistency indicates that the ML-BiGAMP may still retain the same Bayes-optimal performance as the MMSE estimator in high-dimensional applications, although ML-BiGAMP's computational burden is far lower. As an illustrative example of the general ML-BiGAMP, we provide a detector design that could estimate the channel fading and the data symbols jointly with high precision for the two-hop amplify-and-forward relay communication systems.
更多查看译文
关键词
Fading channels, Communication systems, Message passing, Signal processing algorithms, Channel estimation, Detectors, Approximation algorithms, Multi-layer generalized bilinear regression, Bayesian inference, message passing, state evolution, replica method
AI 理解论文
溯源树
样例
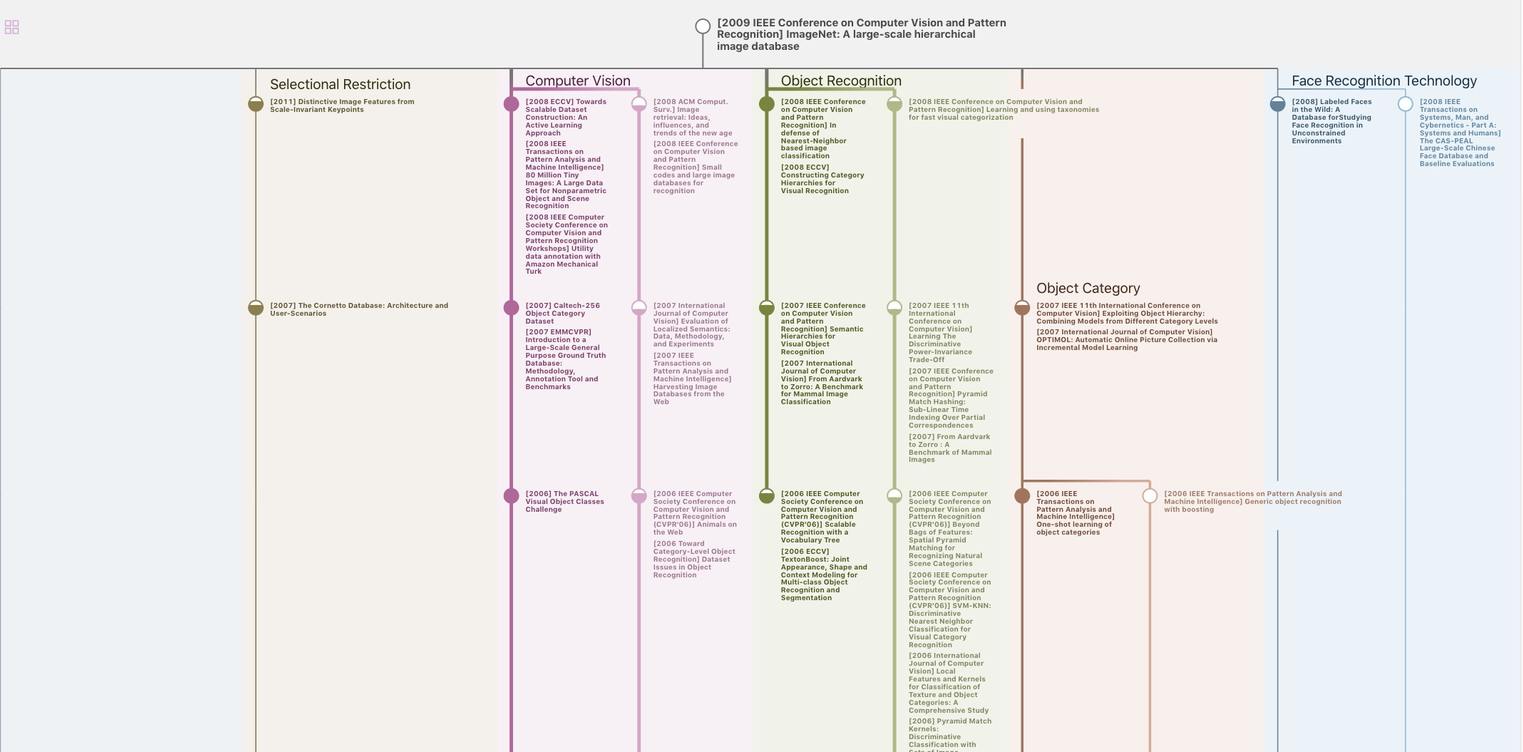
生成溯源树,研究论文发展脉络
Chat Paper
正在生成论文摘要