Autoregressive Mixture Models for Serial Correlation Clustering of Time Series Data
arXiv (Cornell University)(2020)
Abstract
Clustering time series into similar groups can improve models by combining information across like time series. While there is a well developed body of literature for clustering of time series, these approaches tend to generate clusters independently of model training which can lead to poor model fit. We propose a novel distributed approach that simultaneously clusters and fits autoregression models for groups of similar individuals. We apply a Wishart mixture model so as to cluster individuals while modeling the corresponding autocovariance matrices at the same time. The fitted Wishart scale matrices map to cluster-level autoregressive coefficients through the Yule-Walker equations, fitting robust parsimonious autoregressive mixture models. This approach is able to discern differences in underlying autocorrelation variation of time series in settings with large heterogeneous datasets. We prove consistency of our cluster membership estimator, asymptotic distributions of coefficients and compare our approach against competing methods through simulation as well as by fitting a COVID-19 forecast model.
MoreTranslated text
Key words
serial correlation clustering,autoregressive mixture models,time
AI Read Science
Must-Reading Tree
Example
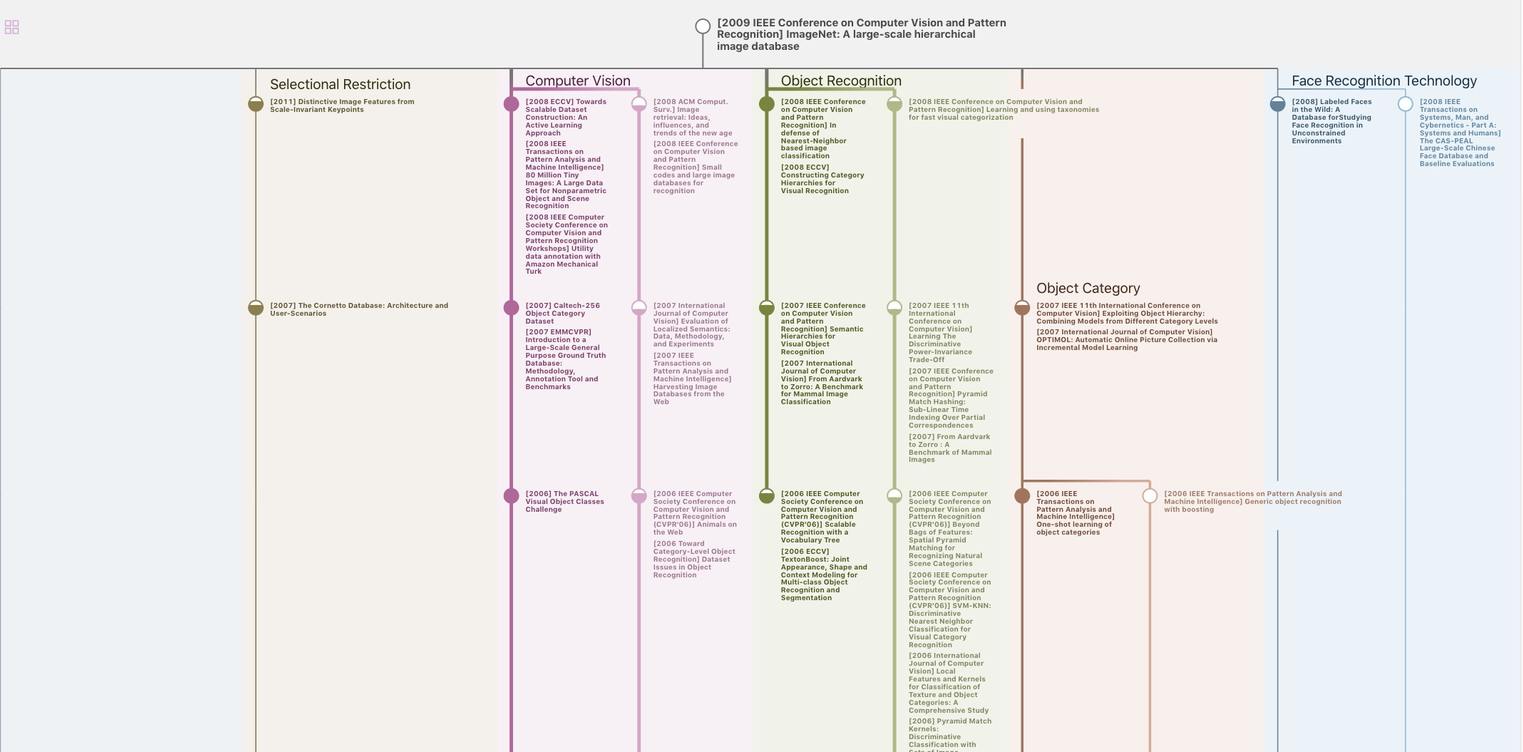
Generate MRT to find the research sequence of this paper
Chat Paper
Summary is being generated by the instructions you defined