Linear inverse problems with nonnegativity constraints: singularity of optimisers
arxiv(2023)
摘要
We look at continuum solutions in optimisation problems associated to linear inverse problems $y = Ax$ with non-negativity constraint $x \geq 0$. We focus on the case where the noise model leads to maximum likelihood estimation through general divergences, which covers a wide range of common noise statistics such as Gaussian and Poisson. Considering $x$ as a Radon measure over the domain on which the reconstruction is taking place, we show a general singularity result. In the high noise regime corresponding to $y \notin \{{Ax}\mid{x \geq 0}\}$ and under a key assumption on the divergence as well as on the operator $A$, any optimiser has a singular part with respect to the Lebesgue measure. We hence provide an explanation as to why any possible algorithm successfully solving the optimisation problem will lead to undesirably spiky-looking images when the image resolution gets finer, a phenomenon well documented in the literature. We illustrate these results with several numerical examples inspired by medical imaging.
更多查看译文
关键词
nonnegativity constraints,linear inverse problems,inverse problems,optimisers
AI 理解论文
溯源树
样例
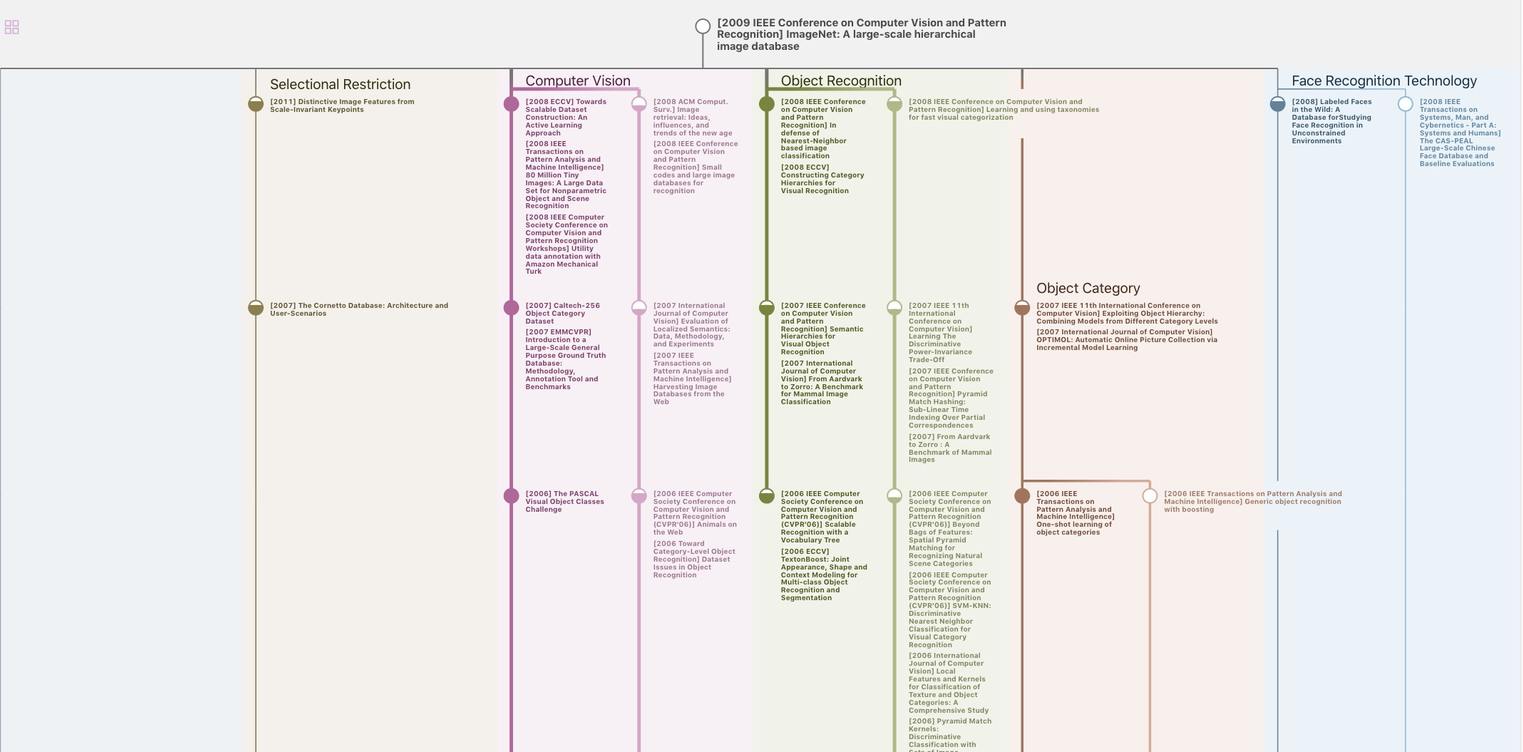
生成溯源树,研究论文发展脉络
Chat Paper
正在生成论文摘要