MAPS: Multi-Agent reinforcement learning-based Portfolio management System.
IJCAI 2020(2020)
摘要
Generating an investment strategy using advanced deep learning methods in stock markets has recently been a topic of interest. Most existing deep learning methods focus on proposing an optimal model or network architecture by maximizing return. However, these models often fail to consider and adapt to the continuously changing market conditions. In this paper, we propose the Multi-Agent reinforcement learning-based Portfolio management System (MAPS). MAPS is a cooperative system in which each agent is an independent "investor" creating its own portfolio. In the training procedure, each agent is guided to act as diversely as possible while maximizing its own return with a carefully designed loss function. As a result, MAPS as a system ends up with a diversified portfolio. Experiment results with 12 years of US market data show that MAPS outperforms most of the baselines in terms of Sharpe ratio. Furthermore, our results show that adding more agents to our system would allow us to get a higher Sharpe ratio by lowering risk with a more diversified portfolio.
更多查看译文
关键词
portfolio management system,reinforcement,maps,multi-agent,learning-based
AI 理解论文
溯源树
样例
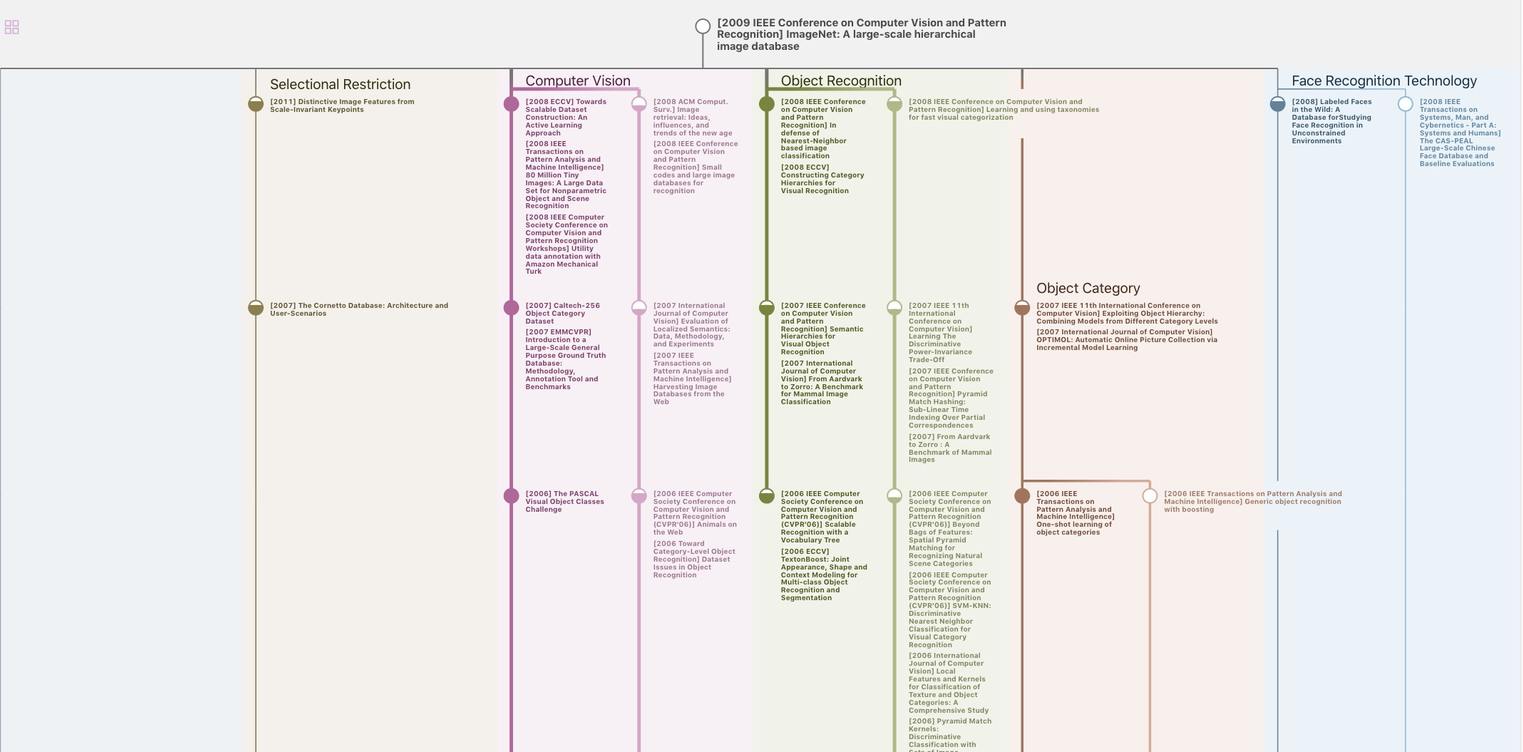
生成溯源树,研究论文发展脉络
Chat Paper
正在生成论文摘要