A Novel Spatio-Temporal Multi-Task Approach for the Prediction of Diabetes-Related Complication: a Cardiopathy Case of Study
PROCEEDINGS OF THE TWENTY-NINTH INTERNATIONAL JOINT CONFERENCE ON ARTIFICIAL INTELLIGENCE(2020)
Abstract
The prediction of the risk profile related to the cardiopathy complication is a core research task that could support clinical decision making. However, the design and implementation of a clinical decision support system based on Electronic Health Record (EHR) temporal data comprise of several challenges. Several single task learning approaches consider the prediction of the risk profile related to a specific diabetes complication (i.e., cardiopathy) independent from other complications. Accordingly, the state-of-the-art multi-task learning (MTL) model encapsulates only the temporal relatedness among the EHR data. However, this assumption might be restricted in the clinical scenario where both spatio-temporal constraints should be taken into account. The aim of this study is the proposal of two different MTL procedures, called spatio-temporal lasso (STL-MTL) and spatio-temporal group lasso (STGL-MTL), which encode the spatio-temporal relatedness using a regularization term and a graph-based approach (i.e., encoding the task relatedness using the structure matrix). Experimental results on a real-world EHR dataset demonstrate the robust performance and the interpretability of the proposed approach.
MoreTranslated text
Key words
Heart Disease Prediction,Medical Concept Embedding,Disease Risk Prediction,Clinical Event Prediction,Temporal Data Analysis
AI Read Science
Must-Reading Tree
Example
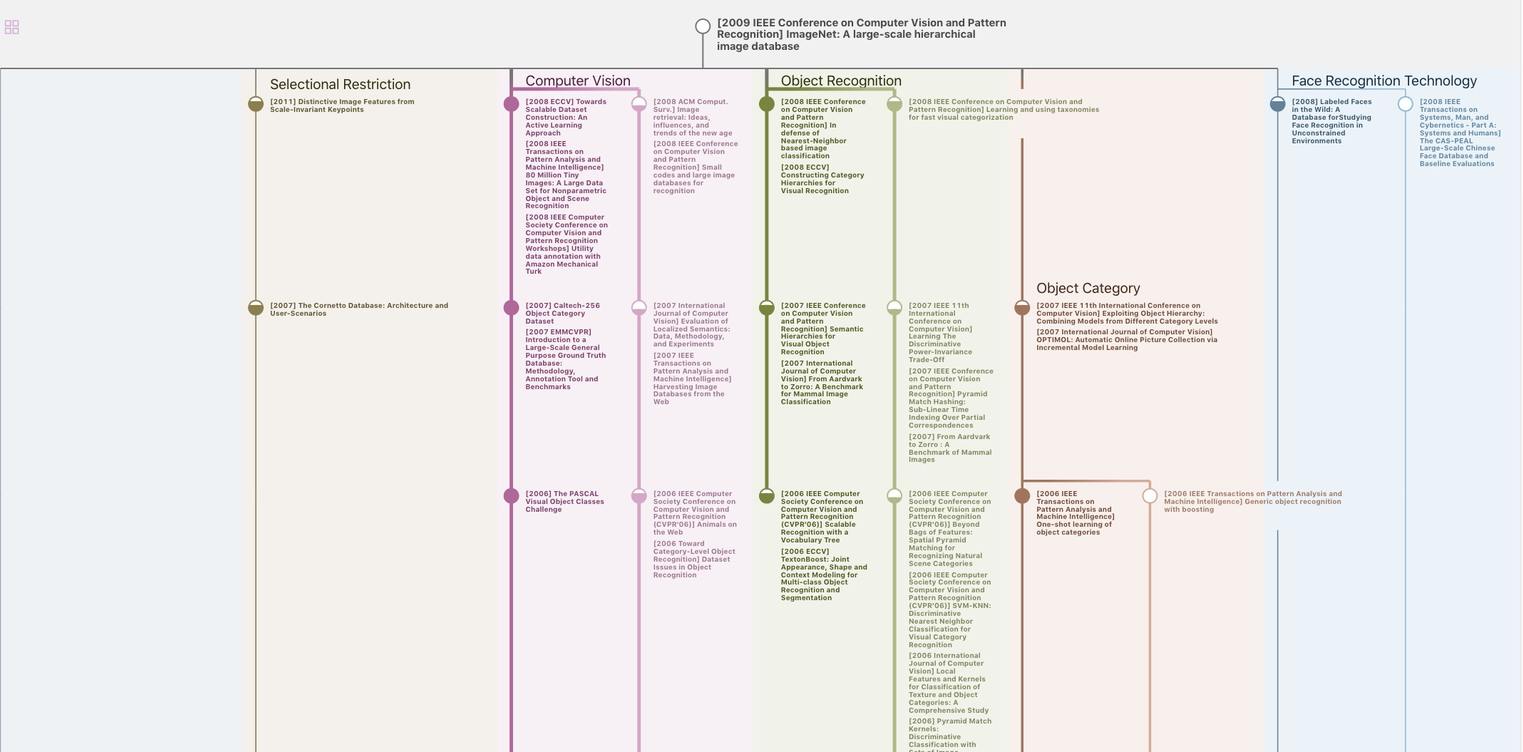
Generate MRT to find the research sequence of this paper
Chat Paper
Summary is being generated by the instructions you defined