Dependent randomized rounding for clustering and partition systems with knapsack constraints
INTERNATIONAL CONFERENCE ON ARTIFICIAL INTELLIGENCE AND STATISTICS, VOL 108(2022)
摘要
Clustering problems are fundamental to unsupervised learning. There is an increased emphasis on fairness in machine learning and AI; one representative notion of fairness is that no single group should be over-represented among the cluster-centers. This, and much more general clus-tering problems, can be formulated with "knapsack" and "partition" constraints. We develop new randomized algorithms targeting such problems, and study two in particular: multi-knapsack me-dian and multi-knapsack center. Our rounding algorithms give new approximation and pseudo -approximation algorithms for these problems.One key technical tool, which may be of independent interest, is a new tail bound analogous to Feige (2006) for sums of random variables with unbounded variances. Such bounds can be useful in inferring properties of large networks using few samples.
更多查看译文
关键词
Dependent rounding, clustering, fairness
AI 理解论文
溯源树
样例
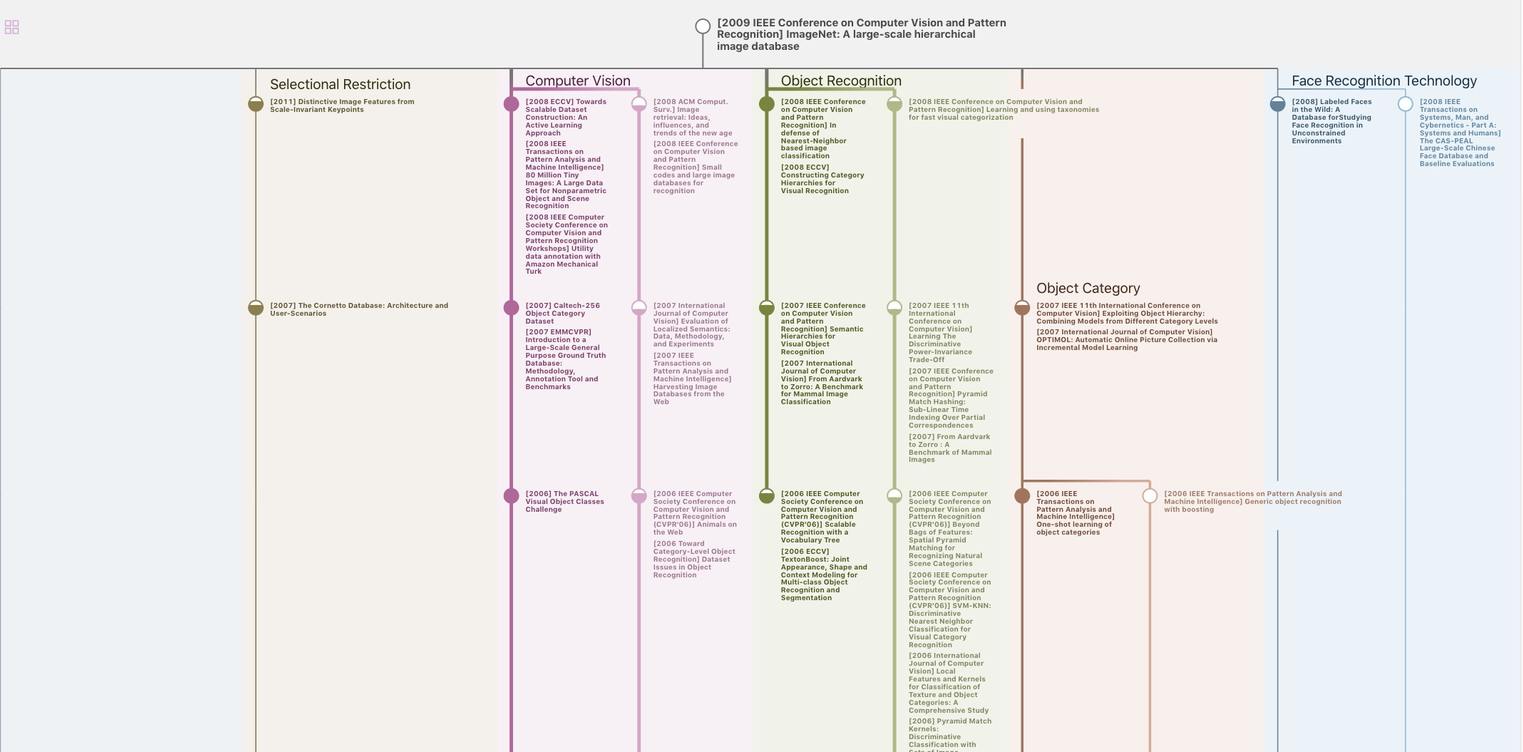
生成溯源树,研究论文发展脉络
Chat Paper
正在生成论文摘要