Taming Neural Networks with TUSLA: Nonconvex Learning via Adaptive Stochastic Gradient Langevin Algorithms
arXiv (Cornell University)(2023)
摘要
Artificial neural networks (ANNs) are typically highly nonlinear systems which are finely tuned via the optimization of their associated, nonconvex loss functions. In many cases, the gradient of any such loss function has superlinear growth, making the use of the widely accepted (stochastic) gradient descent methods, which are based on Euler numerical schemes, problematic. We offer a new learning algorithm based on an appropriately constructed variant of the popular stochastic gradient Langevin dynamics (SGLD), which is called the tamed unadjusted stochastic Langevin algorithm (TUSLA). We also provide a nonasymptotic analysis of the new algorithm's convergence properties in the context of nonconvex learning problems with the use of ANNs. Thus, we provide finite-time guarantees for TUSLA to find approximate minimizers of both empirical and population risks. The roots of the TUSLA algorithm are based on the taming technology for diffusion processes with superlinear coefficients as developed in [S. Sabanis, Electron. Commun. Probab., 18 (2013), pp. 1--10] and [S. Sabanis, Ann. Appl. Probab., 26 (2016), pp. 2083--2105] and for Markov chain Monte 129 (2019), pp. 3638-3663]. Numerical experiments are presented which confirm the theoretical findings and illustrate the need for the use of the new algorithm in comparison to vanilla SGLD within the framework of ANNs.
更多查看译文
关键词
stochastic optimization,nonconvex learning,SGLD,taming,neural networks
AI 理解论文
溯源树
样例
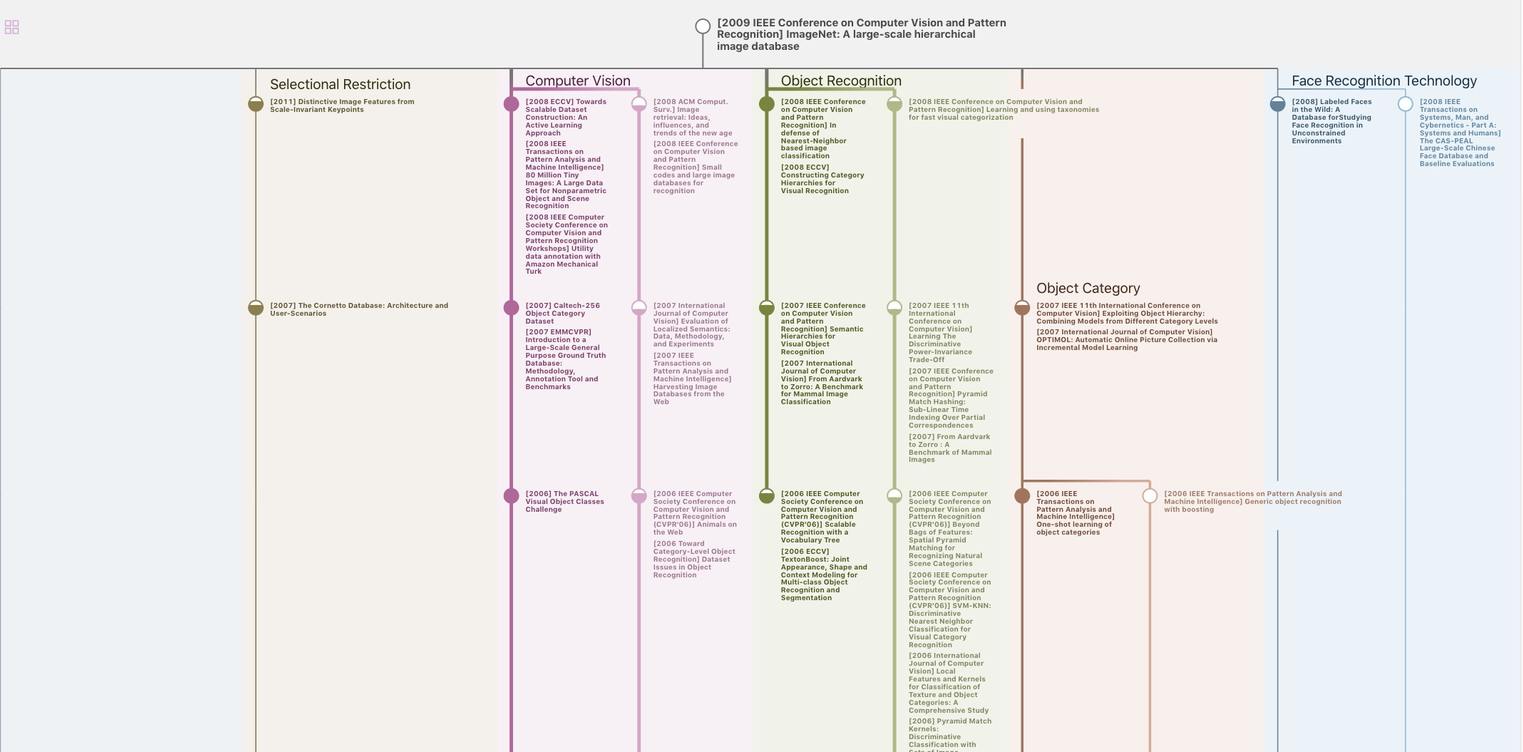
生成溯源树,研究论文发展脉络
Chat Paper
正在生成论文摘要