Active learning and neural network potentials accelerate molecular screening of ether-based solvate ionic liquids.
CHEMICAL COMMUNICATIONS(2020)
摘要
Solvate ionic liquids (SIL) have promising applications as electrolyte materials. Despite the broad design space of oligoether ligands, most reported SILs are based on simple tri- and tetraglyme. Here, we describe a computational search for complex ethers that can better stabilize SILs. Through active learning, a neural network interatomic potential is trained from density functional theory data. The learned potential fulfills two key requirements: transferability across composition space, and high speed and accuracy to find low-energy ligand-ion poses across configurational space. Candidate ether ligands for Li+, Mg(2+)and Na(+)SILs with higher binding affinity and electrochemical stability than the reference compounds are identified. Lastly, their properties are related to the geometry of the coordination sphere.
更多查看译文
AI 理解论文
溯源树
样例
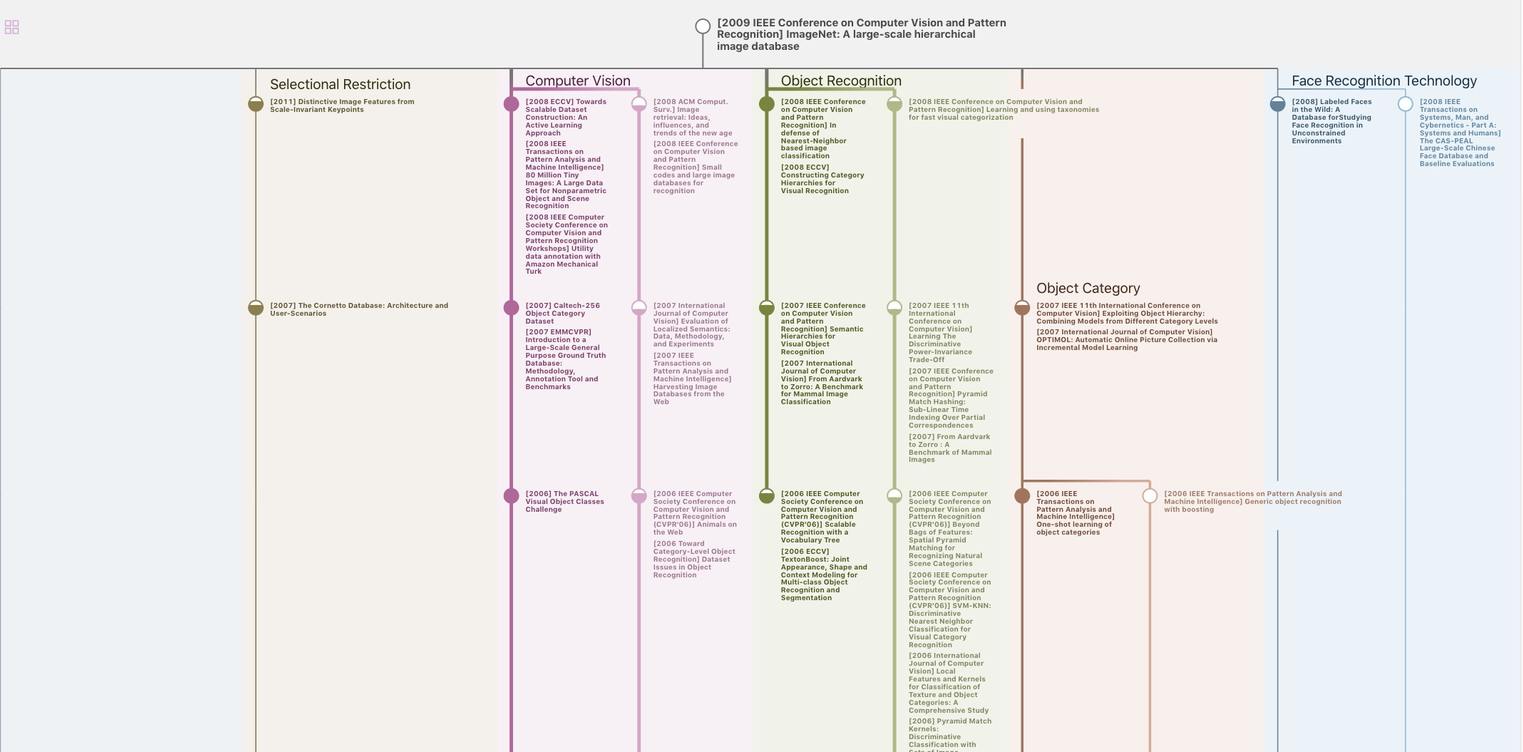
生成溯源树,研究论文发展脉络
Chat Paper
正在生成论文摘要