Interpretation Method For Continuous Glucose Monitoring With Subsequence Time-Series Clustering
DIGITAL PERSONALIZED HEALTH AND MEDICINE(2020)
摘要
We propose mini-batch top-n k-medoids to sequential pattern mining to improve CGM interpretation. Mecical workers can treat specific patient groups better by understanding the time series variation of blood glucose results. For 10 years, continuous glucose monitoring (CGM) has provided time-series data of blood glucose thanks to the invention of devices with low measurement errors. We conducted two experiments. In the first experiment, we evaluated the proposed method with a manually created dataset and confirmed that the method provides more accurate patterns than other clustering methods. In the second experiment, we applied the proposed method to a CGM dataset consisting of real data from 163 patients. We created two labels based on blood glucose (BG) statistics and found patterns that correlated with a specific label in each case.
更多查看译文
关键词
continuous glucose monitoring, subsequence time-series clustering
AI 理解论文
溯源树
样例
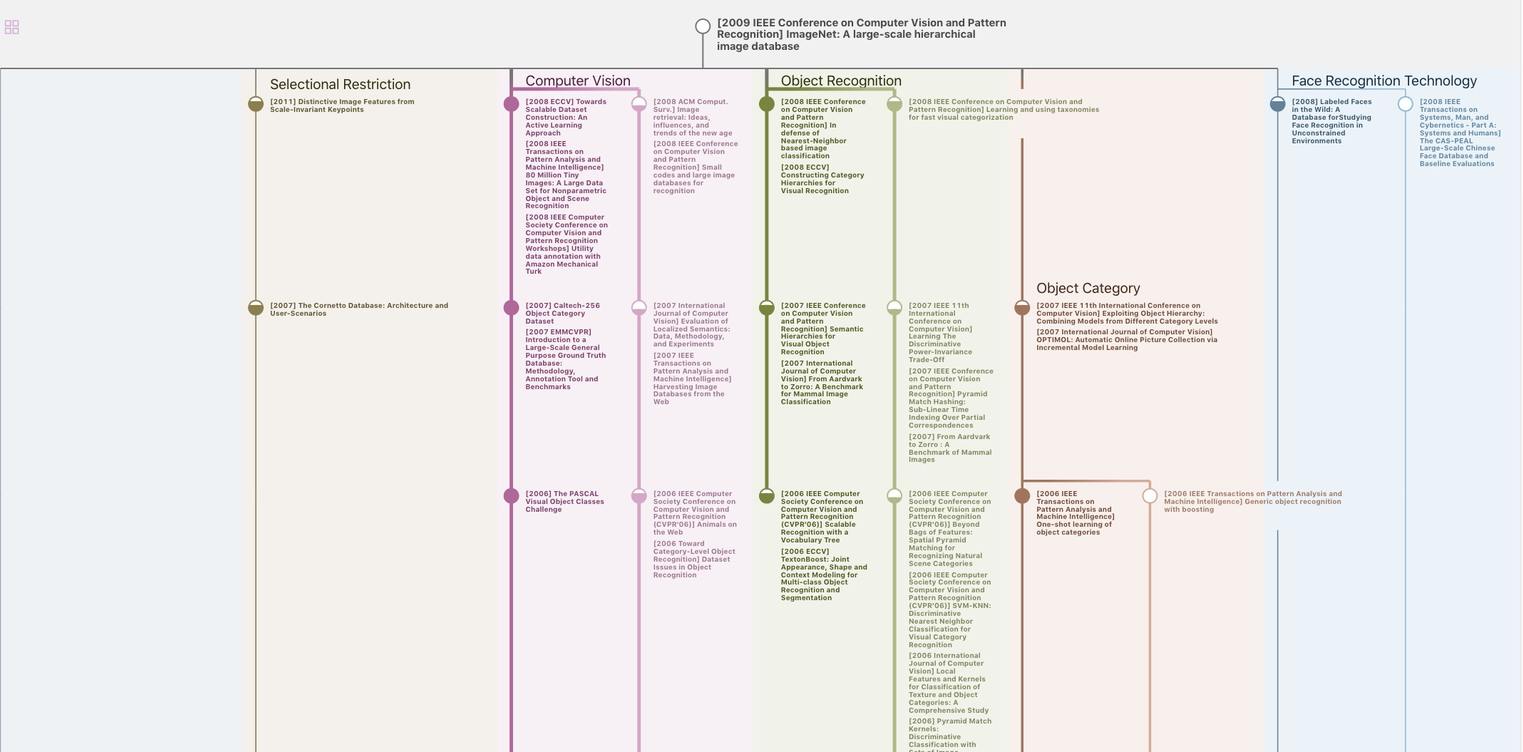
生成溯源树,研究论文发展脉络
Chat Paper
正在生成论文摘要