Grasp State Assessment of Deformable Objects Using Visual-Tactile Fusion Perception
2020 IEEE International Conference on Robotics and Automation (ICRA)(2020)
摘要
Humans can quickly determine the force required to grasp a deformable object to prevent its sliding or excessive deformation through vision and touch, which is still a challenging task for robots. To address this issue, we propose a novel 3D convolution-based visual-tactile fusion deep neural network (C3D-VTFN) to evaluate the grasp state of various deformable objects in this paper. Specifically, we divide the grasp states of deformable objects into three categories of sliding, appropriate and excessive. Also, a dataset for training and testing the proposed network is built by extensive grasping and lifting experiments with different widths and forces on 16 various deformable objects with a robotic arm equipped with a wrist camera and a tactile sensor. As a result, a classification accuracy as high as 99.97% is achieved. Furthermore, some delicate grasp experiments based on the proposed network are implemented in this paper. The experimental results demonstrate that the C3D-VTFN is accurate and efficient enough for grasp state assessment, which can be widely applied to automatic force control, adaptive grasping, and other visual-tactile spatiotemporal sequence learning problems.
更多查看译文
关键词
dexterity grasping,automatic force control,classification,tactile sensor,wrist camera,robotic arm,deformable objects,3D convolution based visual tactile fusion deep neural network,adaptive grasping,extensive grasping,C3D-VTFN,sliding deformation,grasp state assessment
AI 理解论文
溯源树
样例
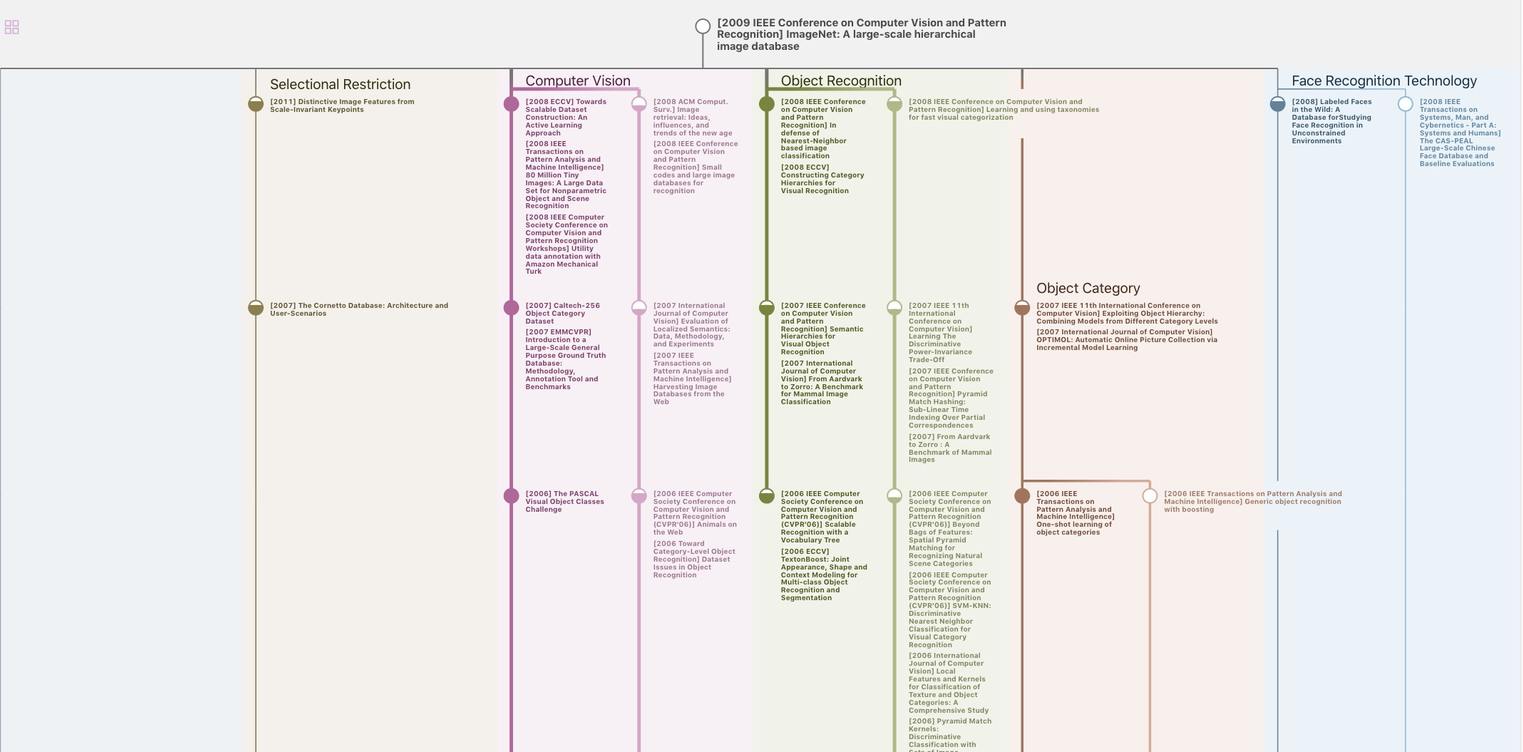
生成溯源树,研究论文发展脉络
Chat Paper
正在生成论文摘要