Optimal Design Of Large-Scale Bayesian Linear Inverse Problems Under Reducible Model Uncertainty: Good To Know What You Don'T Know
SIAM-ASA JOURNAL ON UNCERTAINTY QUANTIFICATION(2021)
摘要
We consider optimal design of infinite-dimensional Bayesian linear inverse problems governed by partial differential equations that contain secondary reducible model uncertainties, in addition to the uncertainty in the inversion parameters. By reducible uncertainties we refer to parametric uncertainties that can be reduced through parameter inference. We seek experimental designs that minimize the posterior uncertainty in the primary parameters while accounting for the uncertainty in secondary parameters. We accomplish this by deriving a marginalized A-optimality criterion and developing an efficient computational approach for its optimization. We illustrate our approach for estimating an uncertain time-dependent source in a contaminant transport model with an uncertain initial state as secondary uncertainty. Our results indicate that accounting for additional model uncertainty in the experimental design process is crucial.
更多查看译文
关键词
optimal experimental design, Bayesian inference, inverse problems, model uncertainty, sensor placement, sparsified designs
AI 理解论文
溯源树
样例
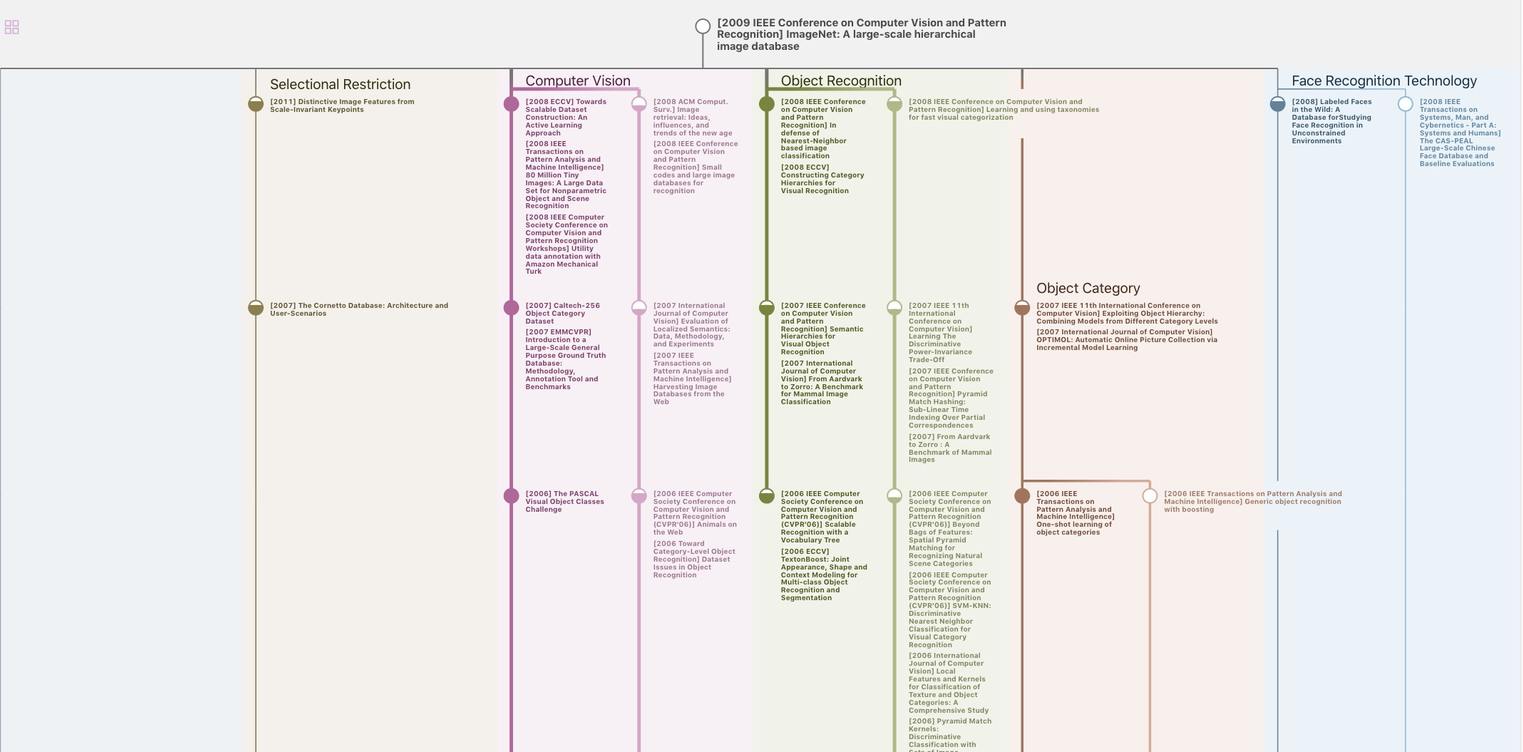
生成溯源树,研究论文发展脉络
Chat Paper
正在生成论文摘要