A Deep Convolutional Neural Network Model For Rapid Prediction Of Fluvial Flood Inundation
arXiv (Cornell University)(2020)
Abstract
Most of the two-dimensional (2D) hydraulic/hydrodynamic models are still computationally too demanding for real-time applications. In this paper, an innovative modelling approach based on a deep convolutional neural network (CNN) method is presented for rapid prediction of fluvial flood inundation. The CNN model is trained using outputs from a 2D hydraulic model (i.e. LISFLOOD-FP) to predict water depths. The pre-trained model is then applied to simulate the January 2005 and December 2015 floods in Carlisle, UK. The CNN predictions are compared favourably with the outputs produced by LISFLOOD-FP. The performance of the CNN model is further confirmed by benchmarking against a support vector regression (SVR) method. The results show that the CNN model outperforms SVR by a large margin. The CNN model is highly accurate in capturing flooded cells as indicated by several quantitative assessment matrices. The estimated error for reproducing maximum flood depth is 0-0.2 m for the 2005 event and 0-0.5 m for the 2015 event at over 99% of the cells covering the computational domain. The proposed CNN method offers great potential for real-time flood modelling/forecasting considering its simplicity, superior performance and computational efficiency.
MoreTranslated text
Key words
Rapid flood modelling, Deep learning, Convolutional neural network, Machine learning, Flood inundation
AI Read Science
Must-Reading Tree
Example
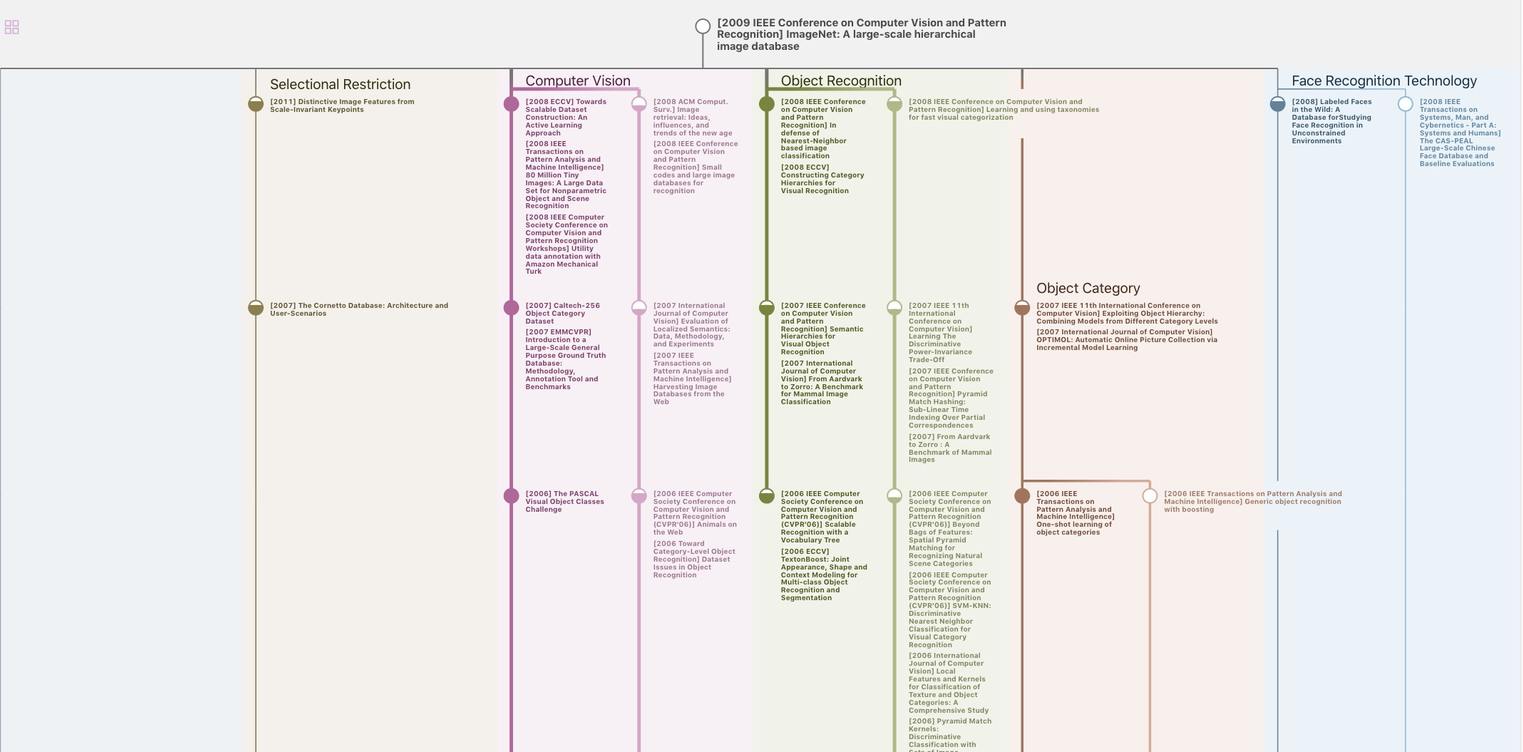
Generate MRT to find the research sequence of this paper
Chat Paper
Summary is being generated by the instructions you defined