Beware the Black-Box: On the Robustness of Recent Defenses to Adversarial Examples
ENTROPY(2021)
摘要
Many defenses have recently been proposed at venues like NIPS, ICML, ICLR and CVPR. These defenses are mainly focused on mitigating white-box attacks. They do not properly examine black-box attacks. In this paper, we expand upon the analyses of these defenses to include adaptive black-box adversaries. Our evaluation is done on nine defenses including Barrage of Random Transforms, ComDefend, Ensemble Diversity, Feature Distillation, The Odds are Odd, Error Correcting Codes, Distribution Classifier Defense, K-Winner Take All and Buffer Zones. Our investigation is done using two black-box adversarial models and six widely studied adversarial attacks for CIFAR-10 and Fashion-MNIST datasets. Our analyses show most recent defenses (7 out of 9) provide only marginal improvements in security (< 25%), as compared to undefended networks. For every defense, we also show the relationship between the amount of data the adversary has at their disposal, and the effectiveness of adaptive black-box attacks. Overall, our results paint a clear picture: defenses need both thorough white-box and black-box analyses to be considered secure. We provide this large scale study and analyses to motivate the field to move towards the development of more robust black-box defenses.
更多查看译文
关键词
adversarial machine learning, black-box attacks, security
AI 理解论文
溯源树
样例
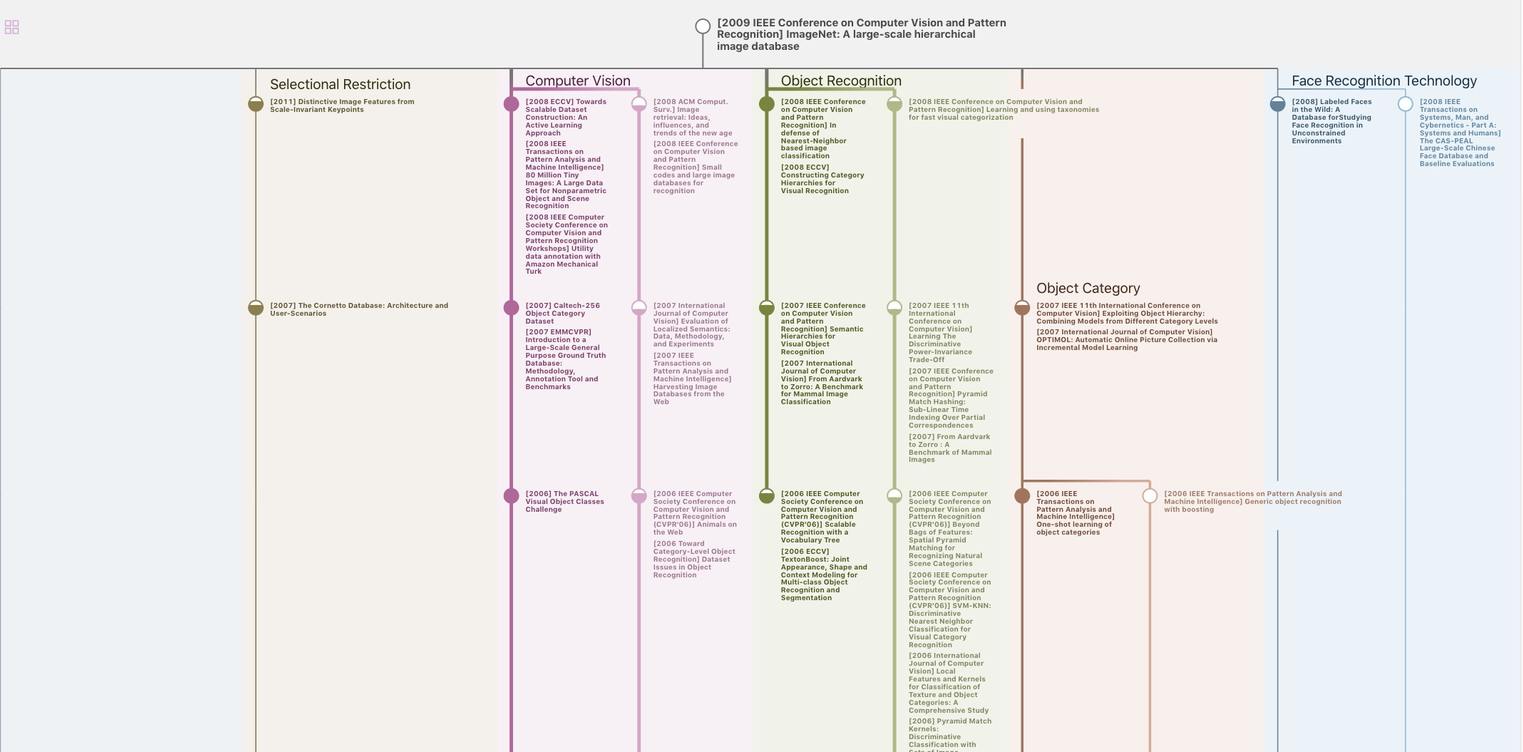
生成溯源树,研究论文发展脉络
Chat Paper
正在生成论文摘要