Zero-Shot Learning with Common Sense Knowledge Graphs
arxiv(2022)
摘要
Zero-shot learning relies on semantic class representations such as hand-engineered attributes or learned embeddings to predict classes without any labeled examples. We propose to learn class representations by embedding nodes from common sense knowledge graphs in a vector space. Common sense knowledge graphs are an untapped source of explicit high-level knowledge that requires little human effort to apply to a range of tasks. To capture the knowledge in the graph, we introduce ZSL-KG, a general-purpose framework with a novel transformer graph convolutional network (TrGCN) for generating class representations. Our proposed TrGCN architecture computes non-linear combinations of node neighbourhoods. Our results show that ZSL-KG improves over existing WordNet-based methods on five out of six zero-shot benchmark datasets in language and vision.
更多查看译文
关键词
knowledge,learning,sense,zero-shot
AI 理解论文
溯源树
样例
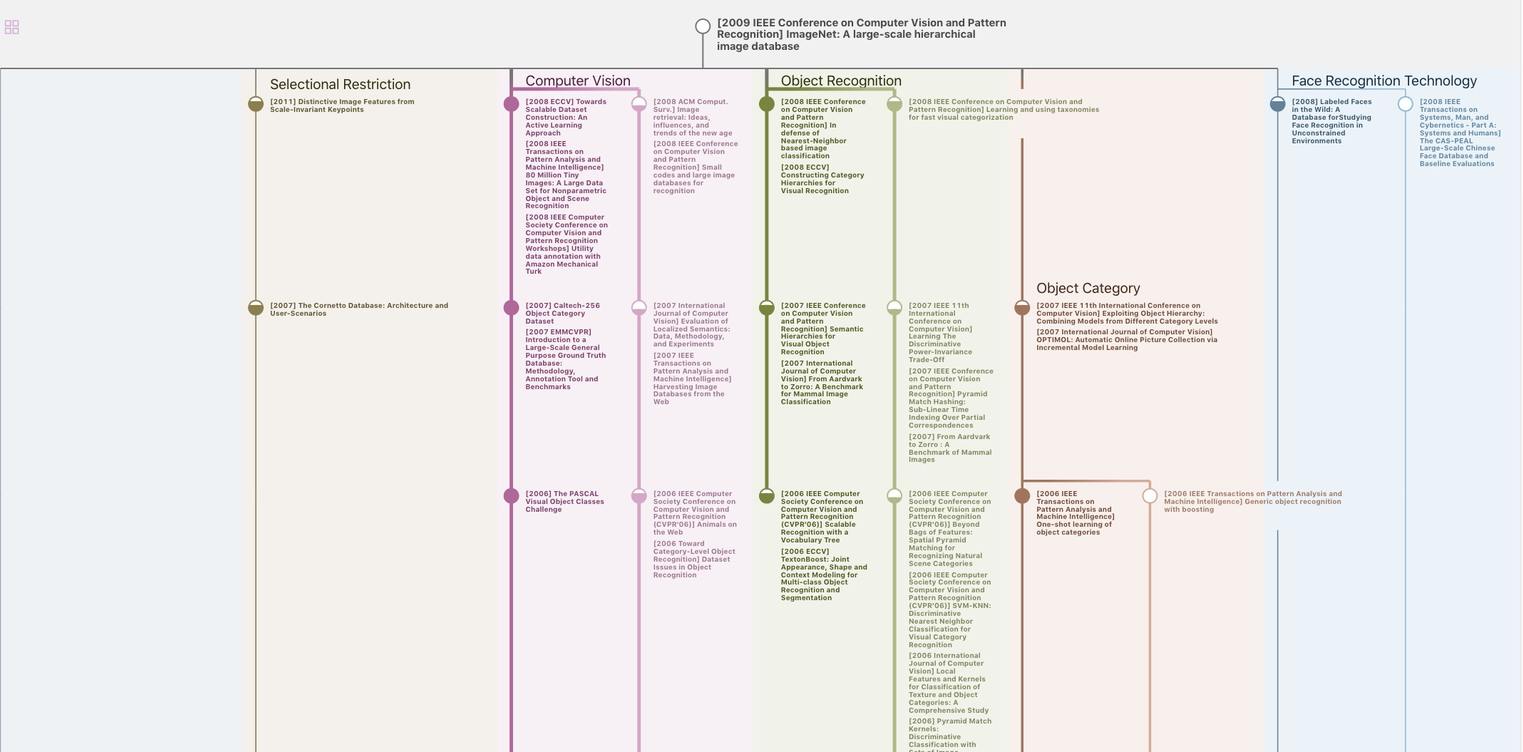
生成溯源树,研究论文发展脉络
Chat Paper
正在生成论文摘要