Quantitative Sensitivity Bounds for Nonlinear Programming and Time-Varying Optimization
IEEE Transactions on Automatic Control(2022)
摘要
Inspired by classical sensitivity results for nonlinear optimization, we derive and discuss new quantitative bounds to characterize the solution map and dual variables of a parametrized nonlinear program. In particular, we derive explicit expressions for the local and global Lipschitz constants of the solution map of nonconvex or convex optimization problems, respectively. Our results are geared towards the study of time-varying optimization problems, which are commonplace in various applications of online optimization, including power systems, robotics, signal processing, and more. In this context, our results can be used to bound the rate of change of the optimizer. To illustrate the use of our sensitivity bounds we generalize existing arguments to quantify the tracking performance of continuous-time, monotone running algorithms. Furthermore, we introduce a new continuous-time running algorithm for time-varying constrained optimization, which we model as a so-called perturbed sweeping process. For this discontinuous scheme we establish an explicit bound on the asymptotic solution tracking for a class of convex problems.
更多查看译文
关键词
Optimization,online optimization
AI 理解论文
溯源树
样例
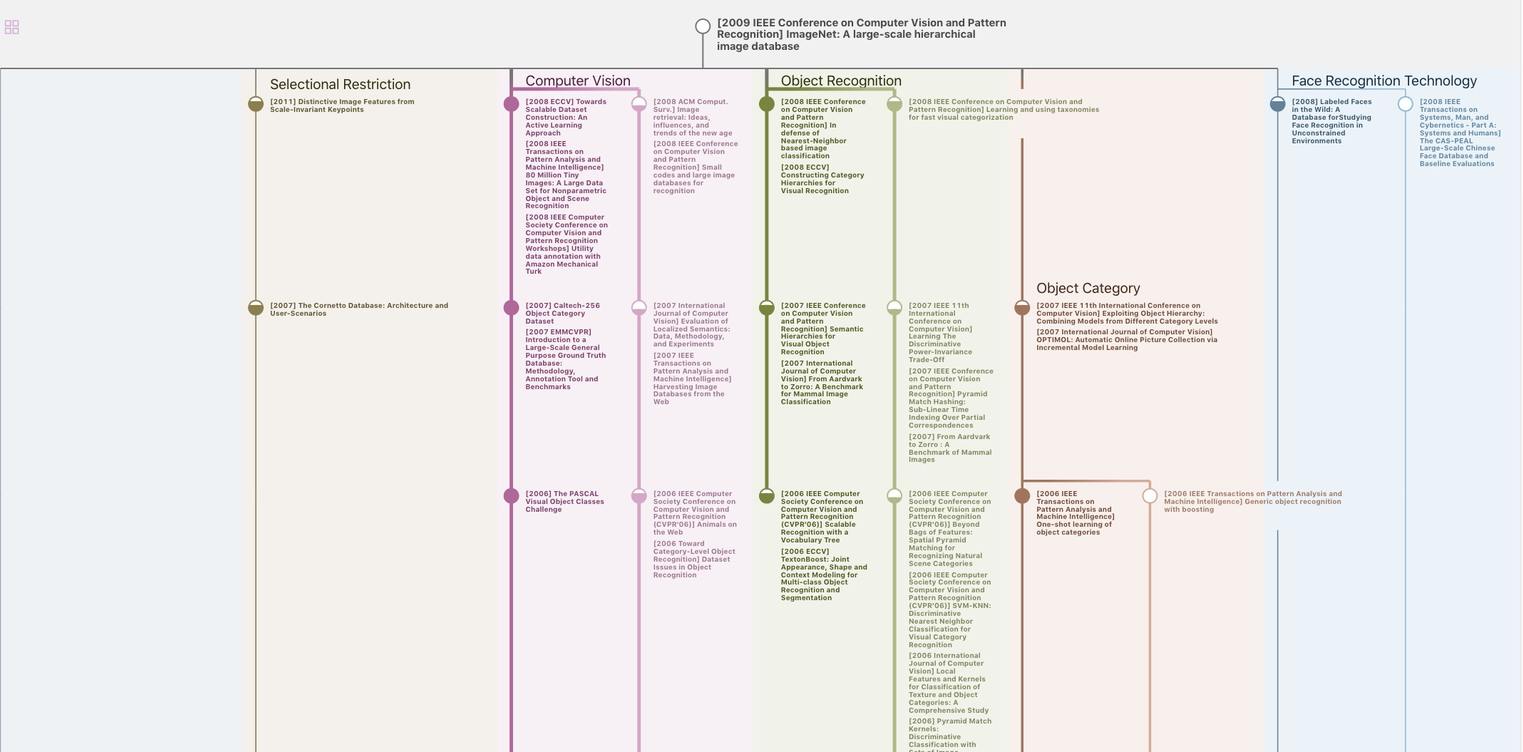
生成溯源树,研究论文发展脉络
Chat Paper
正在生成论文摘要